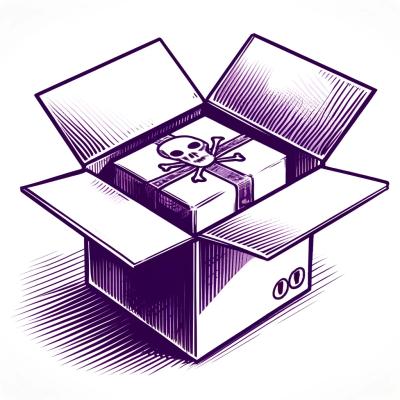
Security News
Research
Data Theft Repackaged: A Case Study in Malicious Wrapper Packages on npm
The Socket Research Team breaks down a malicious wrapper package that uses obfuscation to harvest credentials and exfiltrate sensitive data.
This library uses the Tensorflow & Tensorflow-Probability deep learning libraries to implement & train the models.
Tensorflow [2.4.0+ ]
Tensorflow-Probability [0.10.0+ ]
Note: If upgrading Tensorflow, skip v2.6.0 (buggy) & go to 2.7.0 or higher
A typical workflow will look like this:
import tfr
import pandas as pd
import numpy as np
import pprint
# Ensure the dataset meets the following criteria:
a) No NaNs or infs
b) No mixed datatypes in any column
b) No column names may contain spaces
df = pd.read_csv(...)
'id_col': Unique identifier for time-series' in the dataset. Mandatory.
'target_col': Target Column. Mandatory.
'time_index_col': Any Date or Integer index column that can be used to sort the time-series in ascending order. Mandatory.
'static_num_col_list': A list of numeric columns which are static features i.e. don't change with time. If N/A specify an empty list: []
'static_cat_col_list': A list of string/categorical columns which are static features. If N/A specify empty list: []
'temporal_known_num_col_list': A list of time varying numeric columns which are known at the time of inference for the required Forecast horizon. If N/A spcify empty list [].
'temporal_unknown_num_col_list': A list of time varying numeric columns for which only historical values are known. If N/A spcify empty list [].
'temporal_known_cat_col_list': A list of time varying categorical columns which are known at the time of inference for the required Forecast horizon. If N/A spcify empty list [].
'temporal_unknown_cat_col_list': A list of time varying categorical columns for which only historical values are known. If N/A spcify empty list [].
'strata_col_list': A list of categorical columns to use for stratified sampling. If N/A specify empty list [].
'sort_col_list': A list of columns to be used for sorting the dataframe. Typically ['id_col','time_index_col']. Mandatory.
'wt_col': A numeric column to be used for weighted sampling of time-series'. If N/A specify: None.
columns_dict = {'id_col':'id',
'target_col':'Sales',
'time_index_col':'date',
'static_num_col_list':[],
'static_cat_col_list':['item_id','cat_id','store_id','state_id'],
'temporal_known_num_col_list':['abs_age'],
'temporal_unknown_num_col_list':['sell_price'],
'temporal_known_cat_col_list':['month','wday','Week','event_name_1','event_type_1'],
'temporal_unknown_cat_col_list':['snap_CA','snap_TX','snap_WI'],
'strata_col_list':['state_id','store_id'],
'sort_col_list':['id','date'],
'wt_col':'Weight'}
col_dict: Columns grouping dictionary defined above.
window_len: int(maximum look back history + forecast horizon )
fh: int(forecast horizon)
batch: Specifies training & testing batch size. If using stratified sampling, this is the batch size per strata.
min_nz: Min. no. of non zero values in the Target series within the window_len for it to qualify as a training sample.
PARALLEL_DATA_JOBS: Option to use parallel processing for training batches generation.
PARALLEL_DATA_JOBS_BATCHSIZE: Batch size to process within each of the parallel jobs.
data_obj = tfr.tfr_dataset(col_dict=columns_dict,
window_len=26,
fh=13,
batch=16,
min_nz=1,
PARALLEL_DATA_JOBS=1,
PARALLEL_DATA_JOBS_BATCHSIZE=64)
df = Processed Pandas Dataframe read earlier.
train_till = Date/time_index_col cut-off for training data.
test_till = Date/time_index_col cut-off for testing data. Typically this will be 'train_till + forecast_horizon'
trainset, testset = data_obj.train_test_dataset(df,
train_till=pd.to_datetime('2015-12-31', format='%Y-%M-%d'),
test_till=pd.to_datetime('2016-01-31', format='%Y-%M-%d'))
col_index_dict = data_obj.col_index_dict
vocab = data_obj.vocab_list(df)
infer_dataset, actuals_df = data_obj.infer_dataset(df,
history_till=pd.to_datetime('2015-12-31', format='%Y-%M-%d'),
future_till=pd.to_datetime('2016-01-31', format='%Y-%M-%d'))
where, actuals_df is a dataframe of ground_truths (to be used for evaluation)
num_layers: Int. Specify no. of attention layers in the Transformer model. Typical range [1-4]
num_heads: Int. No. of heads to be used for self attention computation. Typical range [1-4]
d_model: Int. Model Dimension. Typical range [32,64,128]. Multiple of num_heads.
forecast_horizon: same as 'fh' defined above.
max_inp_len: = int(window_len - fh)
loss_type: One of ['Point','Quantile'] for Point forecasts or ['Normal','Poisson','Negbin'] for distribution based forecasts
dropout_rate: % Dropout for regularization
trainset, testset: tf.data.Dataset datasources obtained above
Returns the model object
Select a loss_type & loss_function from the following:
pprint.pprint(tfr.supported_losses)
{'Huber': ['loss_type: Point', 'Usage: Huber(delta=1.0, sample_weights=False)'],
'Negbin': ['loss_type: Negbin', 'Usage: Negbin_NLL_Loss(sample_weights=False)'],
'Normal': ['loss_type: Normal', 'Usage: Normal_NLL_Loss(sample_weights=False)'],
'Poisson': ['loss_type: Poisson', 'Usage: Poisson_NLL_Loss(sample_weights=False)'],
'Quantile': ['loss_type: Quantile', 'Usage: QuantileLoss_v2(quantiles=[0.5], sample_weights=False)'],
'RMSE': ['loss_type: Point', 'Usage: RMSE(sample_weights=False)']
}
e.g.
loss_type = 'Quantile'
loss_fn = QuantileLoss_Weighted(quantiles=[0.6])
try:
del model
except:
pass
model = Simple_Transformer(col_index_dict = col_index_dict,
vocab_dict = vocab,
num_layers = 2,
num_heads = 4,
d_model = 64,
forecast_horizon = 13,
max_inp_len = 13,
loss_type = 'Quantile,
dropout_rate=0.1)
model.build()
train_dataset, test_dataset: tf.data.Dataset objects
loss_function: One of the supported loss functions. See the output of pprint.pprint(supported_losses) for usage.
metric: 'MAE' or 'MSE'
learning_Rate: Typical range [0.001 - 0.00001]
max_epochs, min_epochs: Max & min training epochs
steps_per_epoch: no. of training batches/gradient descent steps per epoch
patience: how many epochs to wait before terminating in case of non-decreasing loss
weighted_training: True/False.
model_prefix: Path where to save models
logdir: Training logs location. Can be viewed with Tensorboard.
best_model = model.train(train_dataset=trainset,
test_dataset=testset,
loss_function=loss_fn,
metric='MSE',
learning_rate=0.0001,
max_epochs=2,
min_epochs=1,
train_steps_per_epoch=10,
test_steps_per_epoch=5,
patience=2,
weighted_training=True,
model_prefix='test_models\tfr_model',
logdir='test_logs')
Skip 'model.build()' if doing only inference using a saved model.
model.load(model_path='test_models\tfr_model_1')
forecast_df = model.infer(infer_dataset)
model = Feature_Weighted_Transformer(col_index_dict = col_index_dict,
vocab_dict = vocab,
num_layers = 2,
num_heads = 4,
d_model = 64,
forecast_horizon = 13,
max_inp_len = 13,
loss_type = 'Quantile,
dropout_rate=0.1)
model.build()
model.train(...) -- usage identical to Simple_Transformer
# Inference returns two outputs:
forecast_df, feature_imp = model.infer(...)
where,
forecast_df - forecasts dataframe
feature_imp - a list of variable importance dataframes in the following order: static_vars_imp_df, historical_vars_imp_df, future_vars_imp_df
Prepare the baseline dataset:
baseline_infer_dataset = data_obj.baseline_infer_dataset(df,
history_till=pd.to_datetime('2016-01-18', format='%Y-%M-%d'),
future_till=pd.to_datetime('2016-01-31', format='%Y-%M-%d'),
ignore_cols=['event_name_1','event_type_1'])
where, ignore_cols is a list of features to zero out while forecasting so as to eliminate their contribution to total forecast.
Call infer as usual:
baseline_forecast_df, _ = model.infer(baseline_infer_dataset)
Evaluation produces two metrics: Forecast_Accuracy & Forecast_Bias expressed as percentages
eval_df = model.evaluate(forecasts=forecast_df, actuals=actuals_df, aggregate_on=['item_id','state_id'])
where, aggregate_on is a list of static categorical columns which provides the level at which to summarize forecast accuracy & bias.
Build Model:
model = Sparse_Simple_Transformer(col_index_dict = col_index_dict,
vocab_dict = vocab,
num_layers = 2,
num_heads = 4,
num_blocks = 2,
kernel_size = 5,
d_model = 64,
forecast_horizon = 13,
max_inp_len = 14,
loss_type = 'Point',
dropout_rate=0.1)
or
model = Sparse_Feature_Weighted_Transformer(col_index_dict = col_index_dict,
vocab_dict = vocab,
num_layers = 2,
num_heads = 4,
num_blocks = 2,
kernel_size = 5,
d_model = 64,
forecast_horizon = 13,
max_inp_len = 14,
loss_type = 'Point',
dropout_rate=0.1)
model.build()
Where,
num_blocks - local attention window size. max_inp_len should be a multiple of num_blocks.
Specify num_blocks > 1 only if working with long sequences.
kernel_size - Conv1D causal convolution layer's kernel size. Basically, the look_back_window at each timestep.
Typical values: [3,5,7,9]
Train: Same as Feature_Weighted_Transformer
Added switch 'low_memory' & 'use_memmap' to the tfr_dataset.train_test_dataset method.
Default: low_memory = True (uses tf.data.Dataset.from_generator API for generating train/test batches). Uses less memory at the expense of speed.
low_memory = False, uses numpy arrays in tf.data.Dataset.from_tensor_slices(). Initial trainset/testset creation takes time but the training speed improves by 4x.
Default: use_memmap = True (uses numpy.memmap files to reduce memory usage). If False, builds train/test arrays in memory (high mem usage)
trainset, testset = data_obj.train_test_dataset(df,
train_till=pd.to_datetime('2015-12-31', format='%Y-%M-%d'),
test_till=pd.to_datetime('2016-01-31', format='%Y-%M-%d'),
low_memory=False,
use_memmap=False)
Plot sample raw time-series:
data_obj.show_ts_samples(data=df, sample_ids=[], n_samples=10, n_col=2, plot_size=(300,600), save=True, filename='ts_samples.html')
Plot sample processed time-series:
data_obj.show_processed_ts_samples(data=df, n_samples=10, n_col=2, plot_size=(300,400), save=True, filename='ts_processed_samples.html')
Create Interactive EDA Report
import eda
eda_object = eda.eda(col_dict=columns_dict, PARALLEL_DATA_JOBS=4, PARALLEL_DATA_JOBS_BATCHSIZE=128) # 'columns_dict' -- similar to the one used in 'tfr_dataset'
eda_object.create_report(data=df, filename='eda_report.html') # df is the pandas dataframe, filename is the full path of the to-be generated report
The create_report method takes a few more arguments:
n_col (default (int): 2) # Configures the grid layout
plot_size (default (tuple of ints): (400,800)) # (Height,Width) of the plot in pixels
time_lags (default (list of ints): [-1,0,1]) # Used for non-linear correlation density plots between target_col & various numeric & categorical columns for specified lags.
max_static_col_levels (default (int): 100) # If there are too many levels to a static feature, the report can get crowded with redundant plots. This parameter helps skip crowded plots with little utility.
TFT sample usage:
import tft
# Create Data Object
data_obj = tft.tft_dataset(col_dict, # Column Groups dictionary (see above)
window_len=192, # Context window size: int(historical series length + forecast_horizon)
fh=24, # forecast_horizon
batch=64, # Specify larger batch size if using 'prefill_buffers=True' in model.train()
min_nz=1, # Minimum non-zero values in the historical sequence to be considered as a training sample
scaling_method='standard_scaling', # scaling method for temporal numeric columns
interleave=1, # legacy. Leave as it is.
PARALLEL_DATA_JOBS=4, # Used for parallelisation. Specify as per available hardware.
PARALLEL_DATA_JOBS_BATCHSIZE=128)
col_index_dict = data_obj.col_index_dict # used to ascertain column positions in the dataframe
vocab = data_obj.vocab_list(df) # get full vocabulary of columns to be embedded
# Create Train & Test sets
trainset, testset = data_obj.train_test_dataset(df,
train_till=pd.to_datetime('2014-08-08 23:00:00', format="%Y-%m-%d %H:%M:%S"),
test_till=pd.to_datetime('2014-08-31 23:00:00', format="%Y-%m-%d %H:%M:%S"))
# Create loss function (a list of supported losses can be found by printing tft.supported_losses)
loss_fn = tft.QuantileLoss_v2(quantiles=[0.5], sample_weights=False)
# Construct Model
model = tft.Temporal_Fusion_Transformer(col_index_dict = col_index_dict,
vocab_dict = vocab,
num_layers = 1,
num_heads = 4,
d_model = 64,
forecast_horizon = 13,
max_inp_len = 13,
loss_type = 'Quantile',
num_quantiles=2,
decoder_start_tokens=1,
dropout_rate=0.1)
model.build()
# Train Model
model.train(train_dataset, # trainset obtain from data_objec using the dataobj.train_test_dataset() method
test_dataset, # testset obtain from data_objec using the dataobj.train_test_dataset() method
loss_function, # Any supported loss function defined in tft.supported_losses
metric='MSE', # Either 'MSE' or 'MAE'
learning_rate=0.0001, # Use higher lr only with valid clipnorm
max_epochs=100,
min_epochs=10,
prefill_buffers=False, # Indicates whether to create a static dataset (requires more memory but trains faster)
num_train_samples=200000, # (NOT USED if prefill_buffers=False)
num_test_samples=50000, # (NOT USED if prefill_buffers=False)
train_batch_size=64, # (NOT USED if prefill_buffers=False, Batch Size specified in data object is used instead)
test_batch_size=128, # (NOT USED if prefill_buffers=False, Batch Size specified in data object is used instead)
train_steps_per_epoch=200, # (NOT USED if prefill_buffers=True)
test_steps_per_epoch=100, # (NOT USED if prefill_buffers=True)
patience=10, # Max epochs to train without further drop in loss value (use higher patience when prefill_buffers=False)
weighted_training=False, # Whether to compute & optimize on the basis of weighted losses
model_prefix='./tft_model',
logdir='/tmp/tft_logs',
opt=None, # provide own optimizer object (default is Adam/Nadam)
clipnorm=0.1, # max global norm applied. Used for stable training. Default is 'None'.
min_delta=0.0001, # min decrease in val. loss to be considered an improvement
shuffle=True) # shuffle training set after each epoch
mode.train returns the path of best trained model.
# Steps to load pre-trained model
# Re-build model
model = tft.Temporal_Fusion_Transformer() # Same parameters as the trained model
model.build()
# load weights
model.load(model_path=model.train())
# Steps to generate forecast
# create infer dataset
infer_dataset, _ = data_obj.infer_dataset(df, history_till=history_till, future_till=future_till)
# infer
forecast_df, features = model.infer(infer_dataset)
STCTN sample usage:
import stctn
... stctn.stctn_dataset
... stctn.supported_losses
model = stctn.Spatial_Temporal_Transformer(col_index_dict = col_index_dict,
vocab_dict = vocab,
num_layers = 4,
num_heads = 1,
d_model = 16,
temporal_kernel_size_list = [1,2,3,4],
spatial_kernel_size = 3,
num_shuffle = 20,
forecast_horizon = 13,
max_inp_len = 13,
loss_type = 'Point',
num_quantiles=1,
dropout_rate=0.1)
model.build()
Train & Infer methods are identical to other transformers.
ConvTFR usage:
import ctfrv2
# Create Data Object
data_obj = ctfrv2.ctfrv2_dataset(col_dict, # Column Groups dictionary (see above)
window_len=192, # Context window size: int(historical series length + forecast_horizon)
fh=24, # forecast_horizon
batch=64, # Specify larger batch size if using 'prefill_buffers=True' in model.train()
min_nz=1, # Minimum non-zero values in the historical sequence to be considered as a training sample
interleave=1, # legacy. Leave as it is.
PARALLEL_DATA_JOBS=4, # Used for parallelisation. Specify as per available hardware.
PARALLEL_DATA_JOBS_BATCHSIZE=128)
col_index_dict = data_obj.col_index_dict # used to ascertain column positions in the dataframe
vocab = data_obj.vocab_list(df) # get full vocabulary of columns to be embedded
# Create Train & Test sets
trainset, testset = data_obj.train_test_dataset(df,
train_till=pd.to_datetime('2014-08-08 23:00:00', format="%Y-%m-%d %H:%M:%S"),
test_till=pd.to_datetime('2014-08-31 23:00:00', format="%Y-%m-%d %H:%M:%S"))
# Create loss function (a list of supported losses can be found by printing tft.supported_losses)
loss_fn = ctfrv2.QuantileLoss_v2(quantiles=[0.5], sample_weights=False)
var_model = ctfrv2.Feature_Weighted_ConvTransformer(col_index_dict = col_index_dict,
vocab_dict = vocab,
num_layers = 2,
num_heads = 4,
kernel_sizes = [1,3,5],
d_model = 32,
forecast_horizon = 13,
max_inp_len = 13,
loss_type = 'Quantile',
num_quantiles = 1,
decoder_lags = 2,
dropout_rate=0.1)
var_model.build()
var_model.train(train_dataset, # trainset obtain from data_objec using the dataobj.train_test_dataset() method
test_dataset, # testset obtain from data_objec using the dataobj.train_test_dataset() method
loss_function, # Any supported loss function defined in tft.supported_losses
metric='MSE', # Either 'MSE' or 'MAE'
learning_rate=0.0001, # Use higher lr only with valid clipnorm
max_epochs=100,
min_epochs=10,
prefill_buffers=False, # Indicates whether to create a static dataset (requires more memory but trains faster)
num_train_samples=200000, # (NOT USED if prefill_buffers=False)
num_test_samples=50000, # (NOT USED if prefill_buffers=False)
train_batch_size=64, # (NOT USED if prefill_buffers=False, Batch Size specified in data object is used instead)
train_steps_per_epoch=200, # (NOT USED if prefill_buffers=True)
test_steps_per_epoch=100, # (NOT USED if prefill_buffers=True)
patience=10, # Max epochs to train without further drop in loss value (use higher patience when prefill_buffers=False)
weighted_training=False, # Whether to compute & optimize on the basis of weighted losses
model_prefix='./tft_model',
logdir='/tmp/tft_logs',
opt=None, # provide own optimizer object (default is Adam/Nadam)
clipnorm=0.1) # max global norm applied. Used for stable training. Default is 'None'.
var_mode.train returns the path of best trained model.
# Steps to load pre-trained model
# Re-build model
var_model = ctfrv2.Feature_Weighted_Transformer() # Same parameters as the trained model
var_model.build()
# load weights
var_model.load(model_path=var_model.train())
# Steps to generate forecast
# create infer dataset
infer_dataset, _ = data_obj.infer_dataset(df, history_till=history_till, future_till=future_till)
# infer
forecast_df, features = var_model.infer(infer_dataset)
data_obj = sage.sage_dataset(...,scaling_method = 'mean_scaling') # Choose one of these methods ['mean_scaling','standard_scaling','no_scaling']
model = sage.SageModel(col_index_dict = col_index_dict,
vocab_dict = vocab,
num_layers = 1,
num_heads = 4,
kernel_sizes = [1],
d_model = 64,
forecast_horizon = int(24),
max_inp_len = int(168),
loss_type = 'Quantile',
num_quantiles = 1,
dropout_rate = 0.1)
# Train Model
model.train(train_dataset, # trainset obtain from data_objec using the dataobj.train_test_dataset() method
test_dataset, # testset obtain from data_objec using the dataobj.train_test_dataset() method
loss_function, # Any supported loss function defined in tft.supported_losses
metric='MSE', # Either 'MSE' or 'MAE'
learning_rate=0.0001, # Use higher lr only with valid clipnorm
max_epochs=100,
min_epochs=10,
prefill_buffers=False, # Indicates whether to create a static dataset (requires more memory but trains faster)
num_train_samples=200000, # (NOT USED if prefill_buffers=False)
num_test_samples=50000, # (NOT USED if prefill_buffers=False)
train_batch_size=64, # (NOT USED if prefill_buffers=False, Batch Size specified in data object is used instead)
test_batch_size=128, # (NOT USED if prefill_buffers=False, Batch Size specified in data object is used instead)
train_steps_per_epoch=200, # (NOT USED if prefill_buffers=True)
test_steps_per_epoch=100, # (NOT USED if prefill_buffers=True)
patience=10, # Max epochs to train without further drop in loss value (use higher patience when prefill_buffers=False)
weighted_training=False, # Whether to compute & optimize on the basis of weighted losses
model_prefix='./tft_model',
logdir='/tmp/tft_logs',
load_model=None, # or, path of a previously saved model to continue training
opt=None, # provide own optimizer object (default is Adam/Nadam)
clipnorm=0.1, # max global norm applied. Used for stable training. Default is 'None'.
min_delta=0.0001, # Min decrease in validation loss to consider an epoch as improvement
shuffle=True) # shuffle train dataset after each epoch
# Inference Steps are similar to TFT or CTFRV2 models
data_obj = [tft_gpu | sage_gpu | ctfrv2_gpu].[tft | sage | ctfrv2]_dataset(col_dict=model_columns_dict,
window_len=int(120),
fh=int(28),
batch=32,
min_nz=1,
max_per_key_train_samples=110,
max_per_key_test_samples=20,
scaling_method='mean_scaling',
interleave=1,
PARALLEL_DATA_JOBS=6,
PARALLEL_DATA_JOBS_BATCHSIZE=128)
where,
batch: no. of unique ids to process at a time
max_per_key_train_samples, max_per_key_test_samples: Max samples to extract from a single time series (default: -1, will extract all possible samples. For e.g. if the timeseries has 100 data points & a window_len of 50 is used, 100 - 50 = 50 samples will be extracted by default)
scaling_method: mean, standard & no (external) scaling supported
model = sage_gpu.SageModel(col_index_dict = col_index_dict,
vocab_dict = vocab,
num_layers = 1,
num_heads = 4,
kernel_sizes = [1],
d_model = 64,
forecast_horizon = int(24),
max_inp_len = int(168),
loss_type = 'Quantile',
num_quantiles = 1,
dropout_rate = 0.1,
seed = <int>,
deterministic_ops = [True | False])
Poisson: ['loss_type: Poisson', 'Usage: Poisson_Loss(log_scale=False, sample_weights=False)']
Tweedie: ['loss_type: Tweedie', 'Usage: Tweedie_Loss(p=1.5, log_scale=False, sample_weights=False)']
FAQs
Forecast ML library
We found that fmldk demonstrated a healthy version release cadence and project activity because the last version was released less than a year ago. It has 1 open source maintainer collaborating on the project.
Did you know?
Socket for GitHub automatically highlights issues in each pull request and monitors the health of all your open source dependencies. Discover the contents of your packages and block harmful activity before you install or update your dependencies.
Security News
Research
The Socket Research Team breaks down a malicious wrapper package that uses obfuscation to harvest credentials and exfiltrate sensitive data.
Research
Security News
Attackers used a malicious npm package typosquatting a popular ESLint plugin to steal sensitive data, execute commands, and exploit developer systems.
Security News
The Ultralytics' PyPI Package was compromised four times in one weekend through GitHub Actions cache poisoning and failure to rotate previously compromised API tokens.