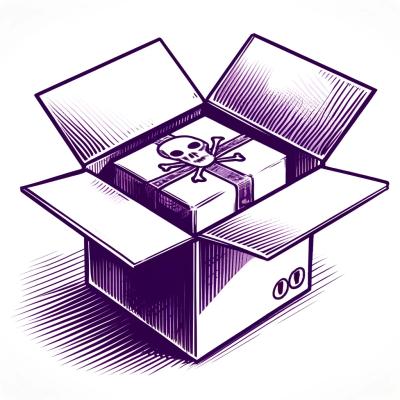
Security News
Research
Data Theft Repackaged: A Case Study in Malicious Wrapper Packages on npm
The Socket Research Team breaks down a malicious wrapper package that uses obfuscation to harvest credentials and exfiltrate sensitive data.
📢 BREAKING NEWS: We released Chronos, a suite of pretrained models for zero-shot time series forecasting. Chronos can generate accurate probabilistic predictions for new time series not seen during training. Check it out here!
GluonTS is a Python package for probabilistic time series modeling, focusing on deep learning based models, based on PyTorch and MXNet.
GluonTS requires Python 3.7 or newer, and the easiest way to install it is via
pip
:
# install with support for torch models
pip install "gluonts[torch]"
# install with support for mxnet models
pip install "gluonts[mxnet]"
See the documentation for more info on how GluonTS can be installed.
To illustrate how to use GluonTS, we train a DeepAR-model and make predictions using the airpassengers dataset. The dataset consists of a single time series of monthly passenger numbers between 1949 and 1960. We train the model on the first nine years and make predictions for the remaining three years.
import pandas as pd
import matplotlib.pyplot as plt
from gluonts.dataset.pandas import PandasDataset
from gluonts.dataset.split import split
from gluonts.torch import DeepAREstimator
# Load data from a CSV file into a PandasDataset
df = pd.read_csv(
"https://raw.githubusercontent.com/AileenNielsen/"
"TimeSeriesAnalysisWithPython/master/data/AirPassengers.csv",
index_col=0,
parse_dates=True,
)
dataset = PandasDataset(df, target="#Passengers")
# Split the data for training and testing
training_data, test_gen = split(dataset, offset=-36)
test_data = test_gen.generate_instances(prediction_length=12, windows=3)
# Train the model and make predictions
model = DeepAREstimator(
prediction_length=12, freq="M", trainer_kwargs={"max_epochs": 5}
).train(training_data)
forecasts = list(model.predict(test_data.input))
# Plot predictions
plt.plot(df["1954":], color="black")
for forecast in forecasts:
forecast.plot()
plt.legend(["True values"], loc="upper left", fontsize="xx-large")
plt.show()
Note, the forecasts are displayed in terms of a probability distribution and the shaded areas represent the 50% and 90% prediction intervals.
If you wish to contribute to the project, please refer to our contribution guidelines.
If you use GluonTS in a scientific publication, we encourage you to add the following references to the related papers, in addition to any model-specific references that are relevant for your work:
@article{gluonts_jmlr,
author = {Alexander Alexandrov and Konstantinos Benidis and Michael Bohlke-Schneider
and Valentin Flunkert and Jan Gasthaus and Tim Januschowski and Danielle C. Maddix
and Syama Rangapuram and David Salinas and Jasper Schulz and Lorenzo Stella and
Ali Caner Türkmen and Yuyang Wang},
title = {{GluonTS: Probabilistic and Neural Time Series Modeling in Python}},
journal = {Journal of Machine Learning Research},
year = {2020},
volume = {21},
number = {116},
pages = {1-6},
url = {http://jmlr.org/papers/v21/19-820.html}
}
@article{gluonts_arxiv,
author = {Alexandrov, A. and Benidis, K. and Bohlke-Schneider, M. and
Flunkert, V. and Gasthaus, J. and Januschowski, T. and Maddix, D. C.
and Rangapuram, S. and Salinas, D. and Schulz, J. and Stella, L. and
Türkmen, A. C. and Wang, Y.},
title = {{GluonTS: Probabilistic Time Series Modeling in Python}},
journal = {arXiv preprint arXiv:1906.05264},
year = {2019}
}
FAQs
Probabilistic time series modeling in Python.
We found that gluonts demonstrated a healthy version release cadence and project activity because the last version was released less than a year ago. It has 3 open source maintainers collaborating on the project.
Did you know?
Socket for GitHub automatically highlights issues in each pull request and monitors the health of all your open source dependencies. Discover the contents of your packages and block harmful activity before you install or update your dependencies.
Security News
Research
The Socket Research Team breaks down a malicious wrapper package that uses obfuscation to harvest credentials and exfiltrate sensitive data.
Research
Security News
Attackers used a malicious npm package typosquatting a popular ESLint plugin to steal sensitive data, execute commands, and exploit developer systems.
Security News
The Ultralytics' PyPI Package was compromised four times in one weekend through GitHub Actions cache poisoning and failure to rotate previously compromised API tokens.