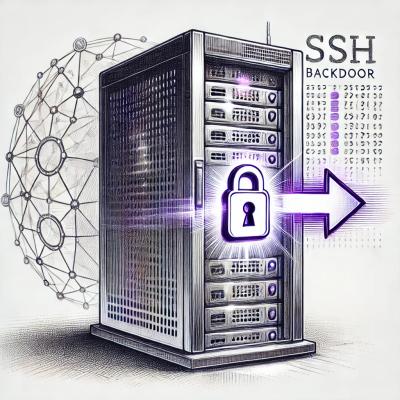
Research
Security News
Malicious npm Packages Inject SSH Backdoors via Typosquatted Libraries
Socket’s threat research team has detected six malicious npm packages typosquatting popular libraries to insert SSH backdoors.
Leveraging graph data structures for complex feature engineering pipelines.
GraphReduce is an abstraction for building machine learning feature engineering pipelines that involve many tables in a composable way. The library is intended to help bridge the gap between research feature definitions and production deployment without the overhead of a full feature store. Underneath the hood, GraphReduce uses graph data structures to represent tables/files as nodes and foreign keys as edges.
Compute backends supported: pandas
, dask
, spark
, AWS Athena, Redshift, Snowflake, postgresql, MySQL
Compute backends coming soon: ray
# from pypi
pip install graphreduce
# from github
pip install 'graphreduce@git+https://github.com/wesmadrigal/graphreduce.git'
# install from source
git clone https://github.com/wesmadrigal/graphreduce && cd graphreduce && python setup.py install
Machine learning requires vectors of data, but our tabular datasets
are disconnected. They can be represented as a graph, where tables
are nodes and join keys are edges. In many model building scenarios
there isn't a nice ML-ready vector waiting for us, so we must curate
the data by joining many tables together to flatten them into a vector.
This is the problem graphreduce
sets out to solve.
featuretools
are limited to pandas
and a couple of SQL databasesspark
but their implementation outside of the paper could not be foundpandas
, dask
, spark
, AWS Athena, AWS Redshift, Snowflake, postgresql, mysql, and more comingAn example dataset might look like the following:
customer
import datetime
import pandas as pd
from graphreduce.node import GraphReduceNode, DynamicNode
from graphreduce.enum import ComputeLayerEnum, PeriodUnit
from graphreduce.graph_reduce import GraphReduce
# source from a csv file with the relationships
# using the file at: https://github.com/wesmadrigal/GraphReduce/blob/master/examples/cust_graph_labels.csv
reldf = pd.read_csv('cust_graph_labels.csv')
# using the data from: https://github.com/wesmadrigal/GraphReduce/tree/master/tests/data/cust_data
files = {
'cust.csv' : {'prefix':'cu'},
'orders.csv':{'prefix':'ord'},
'order_products.csv': {'prefix':'op'},
'notifications.csv':{'prefix':'notif'},
'notification_interactions.csv':{'prefix':'ni'},
'notification_interaction_types.csv':{'prefix':'nit'}
}
# create graph reduce nodes
gr_nodes = {
f.split('/')[-1]: DynamicNode(
fpath=f,
fmt='csv',
pk='id',
prefix=files[f]['prefix'],
date_key=None,
compute_layer=GraphReduceComputeLayerEnum.pandas,
compute_period_val=730,
compute_period_unit=PeriodUnit.day,
)
for f in files.keys()
}
gr = GraphReduce(
name='cust_dynamic_graph',
parent_node=gr_nodes['cust.csv'],
fmt='csv',
cut_date=datetime.datetime(2023,9,1),
compute_layer=GraphReduceComputeLayerEnum.pandas,
auto_features=True,
auto_feature_hops_front=1,
auto_feature_hops_back=2,
label_node=gr_nodes['orders.csv'],
label_operation='count',
label_field='id',
label_period_val=60,
label_period_unit=PeriodUnit.day
)
# Add graph edges
for ix, row in reldf.iterrows():
gr.add_entity_edge(
parent_node=gr_nodes[row['to_name']],
relation_node=gr_nodes[row['from_name']],
parent_key=row['to_key'],
relation_key=row['from_key'],
reduce=True
)
gr.do_transformations()
2024-04-23 13:49:41 [info ] hydrating graph attributes
2024-04-23 13:49:41 [info ] hydrating attributes for DynamicNode
2024-04-23 13:49:41 [info ] hydrating attributes for DynamicNode
2024-04-23 13:49:41 [info ] hydrating attributes for DynamicNode
2024-04-23 13:49:41 [info ] hydrating attributes for DynamicNode
2024-04-23 13:49:41 [info ] hydrating attributes for DynamicNode
2024-04-23 13:49:41 [info ] hydrating attributes for DynamicNode
2024-04-23 13:49:41 [info ] hydrating graph data
2024-04-23 13:49:41 [info ] checking for prefix uniqueness
2024-04-23 13:49:41 [info ] running filters, normalize, and annotations for <GraphReduceNode: fpath=notification_interaction_types.csv fmt=csv>
2024-04-23 13:49:41 [info ] running filters, normalize, and annotations for <GraphReduceNode: fpath=notification_interactions.csv fmt=csv>
2024-04-23 13:49:41 [info ] running filters, normalize, and annotations for <GraphReduceNode: fpath=notifications.csv fmt=csv>
2024-04-23 13:49:41 [info ] running filters, normalize, and annotations for <GraphReduceNode: fpath=orders.csv fmt=csv>
2024-04-23 13:49:41 [info ] running filters, normalize, and annotations for <GraphReduceNode: fpath=order_products.csv fmt=csv>
2024-04-23 13:49:41 [info ] running filters, normalize, and annotations for <GraphReduceNode: fpath=cust.csv fmt=csv>
2024-04-23 13:49:41 [info ] depth-first traversal through the graph from source: <GraphReduceNode: fpath=cust.csv fmt=csv>
2024-04-23 13:49:41 [info ] reducing relation <GraphReduceNode: fpath=notification_interactions.csv fmt=csv>
2024-04-23 13:49:41 [info ] performing auto_features on node <GraphReduceNode: fpath=notification_interactions.csv fmt=csv>
2024-04-23 13:49:41 [info ] joining <GraphReduceNode: fpath=notification_interactions.csv fmt=csv> to <GraphReduceNode: fpath=notifications.csv fmt=csv>
2024-04-23 13:49:41 [info ] reducing relation <GraphReduceNode: fpath=notifications.csv fmt=csv>
2024-04-23 13:49:41 [info ] performing auto_features on node <GraphReduceNode: fpath=notifications.csv fmt=csv>
2024-04-23 13:49:41 [info ] joining <GraphReduceNode: fpath=notifications.csv fmt=csv> to <GraphReduceNode: fpath=cust.csv fmt=csv>
2024-04-23 13:49:41 [info ] reducing relation <GraphReduceNode: fpath=order_products.csv fmt=csv>
2024-04-23 13:49:41 [info ] performing auto_features on node <GraphReduceNode: fpath=order_products.csv fmt=csv>
2024-04-23 13:49:41 [info ] joining <GraphReduceNode: fpath=order_products.csv fmt=csv> to <GraphReduceNode: fpath=orders.csv fmt=csv>
2024-04-23 13:49:41 [info ] reducing relation <GraphReduceNode: fpath=orders.csv fmt=csv>
2024-04-23 13:49:41 [info ] performing auto_features on node <GraphReduceNode: fpath=orders.csv fmt=csv>
2024-04-23 13:49:41 [info ] joining <GraphReduceNode: fpath=orders.csv fmt=csv> to <GraphReduceNode: fpath=cust.csv fmt=csv>
2024-04-23 13:49:41 [info ] Had label node <GraphReduceNode: fpath=orders.csv fmt=csv>
2024-04-23 13:49:41 [info ] computed labels for <GraphReduceNode: fpath=orders.csv fmt=csv>
gr.parent_node.df
cu_id cu_name notif_customer_id notif_id_count notif_customer_id_count notif_ts_first notif_ts_min notif_ts_max ni_notification_id_min ni_notification_id_max ni_notification_id_sum ni_id_count_min ni_id_count_max ni_id_count_sum ni_notification_id_count_min ni_notification_id_count_max ni_notification_id_count_sum ni_interaction_type_id_count_min ni_interaction_type_id_count_max ni_interaction_type_id_count_sum ni_ts_first_first ni_ts_first_min ni_ts_first_max ni_ts_min_first ni_ts_min_min ni_ts_min_max ni_ts_max_first ni_ts_max_min ni_ts_max_max ord_customer_id ord_id_count ord_customer_id_count ord_ts_first ord_ts_min ord_ts_max op_order_id_min op_order_id_max op_order_id_sum op_id_count_min op_id_count_max op_id_count_sum op_order_id_count_min op_order_id_count_max op_order_id_count_sum op_product_id_count_min op_product_id_count_max op_product_id_count_sum ord_customer_id_dupe ord_id_label
0 1 wes 1 6 6 2022-08-05 2022-08-05 2023-06-23 101.0 106.0 621.0 1.0 3.0 14.0 1.0 3.0 14.0 1.0 3.0 14.0 2022-08-06 2022-08-06 2023-05-15 2022-08-06 2022-08-06 2023-05-15 2022-08-08 2022-08-08 2023-05-15 1.0 2.0 2.0 2023-05-12 2023-05-12 2023-06-01 1.0 2.0 3.0 4.0 4.0 8.0 4.0 4.0 8.0 4.0 4.0 8.0 1.0 1.0
1 2 john 2 7 7 2022-09-05 2022-09-05 2023-05-22 107.0 110.0 434.0 1.0 1.0 4.0 1.0 1.0 4.0 1.0 1.0 4.0 2023-06-01 2023-06-01 2023-06-04 2023-06-01 2023-06-01 2023-06-04 2023-06-01 2023-06-01 2023-06-04 2.0 1.0 1.0 2023-01-01 2023-01-01 2023-01-01 3.0 3.0 3.0 4.0 4.0 4.0 4.0 4.0 4.0 4.0 4.0 4.0 NaN NaN
2 3 ryan 3 2 2 2023-06-12 2023-06-12 2023-09-01 NaN NaN 0.0 NaN NaN 0.0 NaN NaN 0.0 NaN NaN 0.0 NaT NaT NaT NaT NaT NaT NaT NaT NaT 3.0 1.0 1.0 2023-06-01 2023-06-01 2023-06-01 5.0 5.0 5.0 1.0 1.0 1.0 1.0 1.0 1.0 1.0 1.0 1.0 NaN NaN
3 4 tianji 4 2 2 2024-02-01 2024-02-01 2024-02-15 NaN NaN 0.0 NaN NaN 0.0 NaN NaN 0.0 NaN NaN 0.0
gr.plot_graph('my_graph_reduce.html')
from sklearn.linear_model import LinearRegression
from sklearn.model_selection import train_test_split
train, test = train_test_split(gr.parent_node.df)
X = [x for x, y in dict(gr.parent_node.df.dtypes).items() if str(y).startswith('int') or str(y).startswith('float')]
# whether or not the user had an order
Y = 'ord_id_label'
mdl = LinearRegression()
mdl.fit(train[X], train[Y])
graphreduce.graph_reduce.GraphReduce
cut_date
controls the date around which we orient the data in the graphcompute_period_val
controls the amount of time back in history we consider during compute over the graphcompute_period_unit
tells us what unit of time we're usingparent_node
specifies the parent-most node in the graph and, typically, the granularity to which to reduce the datafrom graphreduce.graph_reduce import GraphReduce
from graphreduce.enums import PeriodUnit
gr = GraphReduce(
cut_date=datetime.datetime(2023, 2, 1),
compute_period_val=365,
compute_period_unit=PeriodUnit.day,
parent_node=customer
)
do_transformations
perform all data transformationsplot_graph
plot the graphadd_entity_edge
add an edgeadd_node
add a nodegraphreduce.node.GraphReduceNode
do_annotate
annotation definitions (e.g., split a string column into a new column)do_filters
filter the data on column(s)do_normalize
clip anomalies like exceedingly large values and do normalizationpost_join_annotate
annotations on current node after relations are merged in and we have access to their columns, toodo_reduce
the most import node function, reduction operations: group bys, sum, min, max, etc.do_labels
label definitions if any# alternatively can use a dynamic node
from graphreduce.node import DynamicNode
dyna = DynamicNode(
fpath='s3://some.bucket/path.csv',
compute_layer=ComputeLayerEnum.dask,
fmt='csv',
prefix='myprefix',
date_key='ts',
pk='id'
)
colabbr
abbreviate a columnprep_for_features
filter the node's data by the cut date and the compute period for point in time correctness, also referred to as "time travel" in blogsprep_for_labels
filter the node's data by the cut date and the label period to prepare for labelingFAQs
Leveraging graph data structures for complex feature engineering pipelines.
We found that graphreduce demonstrated a healthy version release cadence and project activity because the last version was released less than a year ago. It has 1 open source maintainer collaborating on the project.
Did you know?
Socket for GitHub automatically highlights issues in each pull request and monitors the health of all your open source dependencies. Discover the contents of your packages and block harmful activity before you install or update your dependencies.
Research
Security News
Socket’s threat research team has detected six malicious npm packages typosquatting popular libraries to insert SSH backdoors.
Security News
MITRE's 2024 CWE Top 25 highlights critical software vulnerabilities like XSS, SQL Injection, and CSRF, reflecting shifts due to a refined ranking methodology.
Security News
In this segment of the Risky Business podcast, Feross Aboukhadijeh and Patrick Gray discuss the challenges of tracking malware discovered in open source softare.