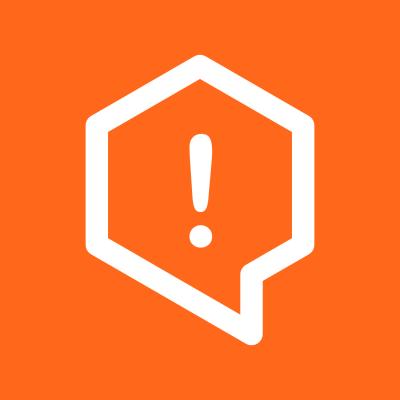
Security News
Fluent Assertions Faces Backlash After Abandoning Open Source Licensing
Fluent Assertions is facing backlash after dropping the Apache license for a commercial model, leaving users blindsided and questioning contributor rights.
With no prior knowledge of machine learning or device-specific deployment, you can deploy a computer vision model to a range of devices and environments using Roboflow Inference.
Inference turns any computer or edge device into a command center for your computer vision projects.
See Example Workflows for common use-cases like detecting small objects with SAHI, multi-model consensus, active learning, reading license plates, blurring faces, background removal, and more.
Install Docker (and NVIDIA Container Toolkit for GPU acceleration if you have a CUDA-enabled GPU). Then run
pip install inference-cli && inference server start --dev
This will pull the proper image for your machine and start it in development mode.
In development mode, a Jupyter notebook server with a quickstart guide runs on
localhost:9002
. Dive in there for a whirlwind tour
of your new Inference Server's functionality!
Now you're ready to connect your camera streams and start building & deploying Workflows in the UI or interacting with your new server via its API.
A key component of Inference is Workflows, composable blocks of common functionality that give models a common interface to make chaining and experimentation easy.
With Workflows, you can:
Workflows allow you to extend simple model predictions to build computer vision micro-services that fit into a larger application or fully self-contained visual agents that run on a video stream.
Learn more, read the Workflows docs, or start building.
Tutorial: Build a Traffic Monitoring Application with Workflows
Once you've installed Infernece, your machine is a fully-featured CV center.
You can use its API to run models and workflows on images and video streams.
By default, the server is running locally on
localhost:9001
.
To interface with your server via Python, use our SDK.
pip install inference-sdk
then run
an example model comparison Workflow
like this:
from inference_sdk import InferenceHTTPClient
client = InferenceHTTPClient(
api_url="http://localhost:9001", # use local inference server
# api_key="<YOUR API KEY>" # optional to access your private data and models
)
result = client.run_workflow(
workspace_name="roboflow-docs",
workflow_id="model-comparison",
images={
"image": "https://media.roboflow.com/workflows/examples/bleachers.jpg"
},
parameters={
"model1": "yolov8n-640",
"model2": "yolov11n-640"
}
)
print(result)
In other languages, use the server's REST API;
you can access the API docs for your server at
/docs
(OpenAPI format) or
/redoc
(Redoc Format).
Check out the inference_sdk docs to see what else you can do with your new server.
The inference server is a video processing beast. You can set it up to run Workflows on RTSP streams, webcam devices, and more. It will handle hardware acceleration, multiprocessing, video decoding and GPU batching to get the most out of your hardware.
This example workflow will watch a stream for frames that CLIP thinks match an inputted text prompt.
from inference_sdk import InferenceHTTPClient
import atexit
import time
max_fps = 4
client = InferenceHTTPClient(
api_url="http://localhost:9001", # use local inference server
# api_key="<YOUR API KEY>" # optional to access your private data and models
)
# Start a stream on an rtsp stream
result = client.start_inference_pipeline_with_workflow(
video_reference=["rtsp://user:password@192.168.0.100:554/"],
workspace_name="roboflow-docs",
workflow_id="clip-frames",
max_fps=max_fps,
workflows_parameters={
"prompt": "blurry", # change to look for something else
"threshold": 0.16
}
)
pipeline_id = result["context"]["pipeline_id"]
# Terminate the pipeline when the script exits
atexit.register(lambda: client.terminate_inference_pipeline(pipeline_id))
while True:
result = client.consume_inference_pipeline_result(pipeline_id=pipeline_id)
if not result["outputs"] or not result["outputs"][0]:
# still initializing
continue
output = result["outputs"][0]
is_match = output.get("is_match")
similarity = round(output.get("similarity")*100, 1)
print(f"Matches prompt? {is_match} (similarity: {similarity}%)")
time.sleep(1/max_fps)
Pipeline outputs can be consumed via API for downstream processing or the Workflow can be configured to call external services with Notification blocks (like Email or Twilio) or the Webhook block. For more info on video pipeline management, see the Video Processing overview.
If you have a Roboflow account & have linked an API key, you can also remotely monitor and manage your running streams via the Roboflow UI.
Without an API Key, you can access a wide range of pre-trained and foundational models and run Workflows via our JSON API.
Pass an optional Roboflow API Key to the inference_sdk
or API to access additional features.
If you don't want to manage your own infrastructure for self-hosting, Roboflow offers a hosted Inference Server via one-click Dedicated Deployments (CPU and GPU machines) billed hourly, or simple models and Workflows via our serverless Hosted API billed per API-call.
We offer a generous free-tier to get started.
Inference is designed to run on a wide range of hardware from beefy cloud servers to tiny edge devices. This lets you easily develop against your local machine or our cloud infrastructure and then seamlessly switch to another device for production deployment.
inference server start
attempts to automatically choose the optimal container to optimize performance on your machine, special installation notes and performance tips by device are listed below.
sudo docker run -p 9001:9001 -v ~/.inference/cache:/tmp/cache roboflow/roboflow-inference-server-cpu:latest
To install the python package natively, install via PyPi
pip install inference
inference server start
but, if you need more speed, the inference
Python package supports hardware acceleration via the onnxruntime CoreMLExecutionProvider and the PyTorch mps
device backend. By using these, inference gets a big boost when running outside of Docker on Apple Silicon.
git clone https://github.com/roboflow/inference.git
cd inference
python3 -m venv inf
source inf/bin/activate
pip install .
cp docker/config/cpu_http.py .
Then start the server by running uvicorn
with the cpu_http
module in your virtual environment:
# source inf/bin/activate
uvicorn cpu_http:app --port 9001 --host 0.0.0.0
Your server is now running at localhost:9001
with MPS acceleration.
To run natively in python, pip install inference
will automatically pull in the CoreMLExecutionProvider on Mac.
inference server start
should run the right container automatically.
roboflow/roboflow-inference-server-gpu:latest
docker container with NVIDIA Container Runtime:
sudo docker run --gpus all --net=host -v ~/.inference/cache:/tmp/cache roboflow/roboflow-inference-server-gpu:latest
Or pip install inference-gpu
to run the python package natively.
You can enable TensorRT by adding TensorrtExecutionProvider
to the ONNXRUNTIME_EXECUTION_PROVIDERS
environment variable.
⚠️ Note: TensorRT is not enabled by default due to long (15+ minute) compilation times each time a new model is initialized. We cache the TensorRT engine in /tmp/cache
, which is a Docker volume mounted from ~/.inference/cache
by default.
export ONNXRUNTIME_EXECUTION_PROVIDERS="[TensorrtExecutionProvider,CUDAExecutionProvider,OpenVINOExecutionProvider,CoreMLExecutionProvider,CPUExecutionProvider]"
NVIDIA GPU (Linux)
above.
inference server start
will automatically detect your JetPack version and use the right container.
sudo docker run --runtime=nvidia --net=host -v ~/.inference/cache:/tmp/cache roboflow/roboflow-inference-server-jetson-6.0.0:latest
You can enable TensorRT by adding TensorrtExecutionProvider
to the ONNXRUNTIME_EXECUTION_PROVIDERS
environment variable.
⚠️ Note: TensorRT is not enabled by default due to long (15+ minute) compilation times each time a new model is initialized. We cache the TensorRT engine in /tmp/cache
, which is a Docker volume mounted from ~/.inference/cache
by default.
sudo docker run \
--runtime=nvidia \
--net=host \
-e ONNXRUNTIME_EXECUTION_PROVIDERS="[TensorrtExecutionProvider,CUDAExecutionProvider,OpenVINOExecutionProvider,CoreMLExecutionProvider,CPUExecutionProvider]" \
-v ~/.inference/cache:/tmp/cache \
roboflow/roboflow-inference-server-jetson-6.0.0:latest
inference server start
and you'll be all set.
ONNXRUNTIME_EXECUTION_PROVIDERS
environment variable.
export ONNXRUNTIME_EXECUTION_PROVIDERS="[ROCMExecutionProvider,OpenVINOExecutionProvider,CPUExecutionProvider]"
This is untested and performance improvements are not guaranteed.
For manufacturing and logistics use-cases Roboflow now offers the Flowbox, a ruggedized CV center pre-configured with Inference and optimized for running in secure networks. It has integrated support for machine vision cameras like Basler and Lucid over GigE, supports interfacing with PLCs and HMIs via OPC or MQTT, enables enterprise device management through a DMZ, and comes with the support of our team of computer vision experts to ensure your project is a success.
Visit our documentation to explore comprehensive guides, detailed API references, and a wide array of tutorials designed to help you harness the full potential of the Inference package.
The core of Inference is licensed under Apache 2.0.
Models are subject to licensing which respects the underlying architecture. These licenses are listed in inference/models
. Paid Roboflow accounts include a commercial license for some models (see roboflow.com/licensing for details).
Cloud connected functionality (like our model and Workflows registries, dataset management, model monitoring, device management, and managed infrastructure) requires a Roboflow account and API key & is metered based on usage.
Enterprise functionality is source-available in inference/enterprise
under an enterprise license and usage in production requires an active Enterprise contract in good standing.
See the "Self Hosting and Edge Deployment" section of the Roboflow Licensing documentation for more information on how Roboflow Inference is licensed.
We would love your input to improve Roboflow Inference! Please see our contributing guide to get started. Thank you to all of our contributors! 🙏
FAQs
With no prior knowledge of machine learning or device-specific deployment, you can deploy a computer vision model to a range of devices and environments using Roboflow Inference.
We found that inference-core demonstrated a healthy version release cadence and project activity because the last version was released less than a year ago. It has 5 open source maintainers collaborating on the project.
Did you know?
Socket for GitHub automatically highlights issues in each pull request and monitors the health of all your open source dependencies. Discover the contents of your packages and block harmful activity before you install or update your dependencies.
Security News
Fluent Assertions is facing backlash after dropping the Apache license for a commercial model, leaving users blindsided and questioning contributor rights.
Research
Security News
Socket researchers uncover the risks of a malicious Python package targeting Discord developers.
Security News
The UK is proposing a bold ban on ransomware payments by public entities to disrupt cybercrime, protect critical services, and lead global cybersecurity efforts.