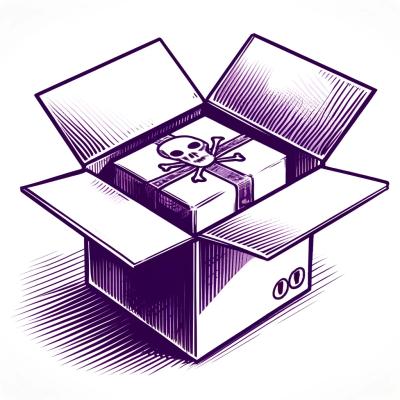
Security News
Research
Data Theft Repackaged: A Case Study in Malicious Wrapper Packages on npm
The Socket Research Team breaks down a malicious wrapper package that uses obfuscation to harvest credentials and exfiltrate sensitive data.
PyTorch implementation of the InfoNCE loss from "Representation Learning with Contrastive Predictive Coding" <https://arxiv.org/abs/1807.03748>
__.
In contrastive learning, we want to learn how to map high dimensional data to a lower dimensional embedding space.
This mapping should place semantically similar samples close together in the embedding space, whilst placing semantically distinct samples further apart.
The InfoNCE loss function can be used for the purpose of contrastive learning.
This package is available on PyPI <https://pypi.org/project/info-nce-pytorch/>
__ and can be installed via:
.. code::
pip install info-nce-pytorch
Can be used without explicit negative keys, whereby each sample is compared with the other samples in the batch.
.. code:: python
loss = InfoNCE()
batch_size, embedding_size = 32, 128
query = torch.randn(batch_size, embedding_size)
positive_key = torch.randn(batch_size, embedding_size)
output = loss(query, positive_key)
Can be used with negative keys, whereby every combination between query and negative key is compared.
.. code:: python
loss = InfoNCE(negative_mode='unpaired') # negative_mode='unpaired' is the default value
batch_size, num_negative, embedding_size = 32, 48, 128
query = torch.randn(batch_size, embedding_size)
positive_key = torch.randn(batch_size, embedding_size)
negative_keys = torch.randn(num_negative, embedding_size)
output = loss(query, positive_key, negative_keys)
Can be used with negative keys, whereby each query sample is compared with only the negative keys it is paired with.
.. code:: python
loss = InfoNCE(negative_mode='paired')
batch_size, num_negative, embedding_size = 32, 6, 128
query = torch.randn(batch_size, embedding_size)
positive_key = torch.randn(batch_size, embedding_size)
negative_keys = torch.randn(batch_size, num_negative, embedding_size)
output = loss(query, positive_key, negative_keys)
Suppose we have some initial mean vectors µ_q
, µ_p
, µ_n
and a covariance matrix Σ = I/10
, then we can plot the value of the InfoNCE loss by sampling from distributions with interpolated mean vectors.
Given interpolation weights α
and β
, we define the distribution Q ~ N(µ_q, Σ)
for the query samples, the distribution P_α ~ N(αµ_q + (1-α)µ_p, Σ)
for the positive samples
and the distribution N_β ~ N(βµ_q + (1-β)µ_n, Σ)
for the negative samples.
Shown below is the value of the loss with inputs sampled from the distributions defined above for different values of α
and β
.
.. image:: https://raw.githubusercontent.com/RElbers/info-nce-pytorch/main/imgs/loss.png
FAQs
PyTorch implementation of the InfoNCE loss for self-supervised learning.
We found that info-nce-pytorch demonstrated a healthy version release cadence and project activity because the last version was released less than a year ago. It has 1 open source maintainer collaborating on the project.
Did you know?
Socket for GitHub automatically highlights issues in each pull request and monitors the health of all your open source dependencies. Discover the contents of your packages and block harmful activity before you install or update your dependencies.
Security News
Research
The Socket Research Team breaks down a malicious wrapper package that uses obfuscation to harvest credentials and exfiltrate sensitive data.
Research
Security News
Attackers used a malicious npm package typosquatting a popular ESLint plugin to steal sensitive data, execute commands, and exploit developer systems.
Security News
The Ultralytics' PyPI Package was compromised four times in one weekend through GitHub Actions cache poisoning and failure to rotate previously compromised API tokens.