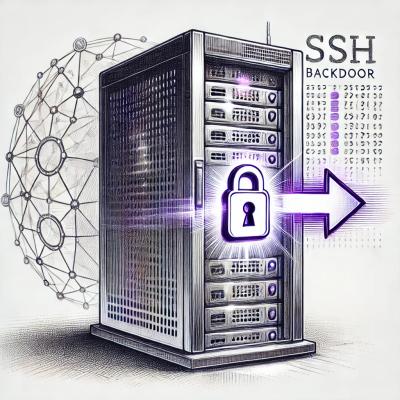
Research
Security News
Malicious npm Packages Inject SSH Backdoors via Typosquatted Libraries
Socket’s threat research team has detected six malicious npm packages typosquatting popular libraries to insert SSH backdoors.
CI/CD | |
Package | |
Meta |
Koheesio - the Finnish word for cohesion - is a robust Python framework designed to build efficient data pipelines. It encourages modularity and collaboration, allowing the creation of complex pipelines from simple, reusable components.
Koheesio is a versatile framework that supports multiple implementations and works seamlessly with various data processing libraries or frameworks. This ensures that Koheesio can handle any data processing task, regardless of the underlying technology or data scale.
Koheesio uses Pydantic for strong typing, data validation, and settings management, ensuring a high level of type safety and structured configurations within pipeline components.
The goal of Koheesio is to ensure predictable pipeline execution through a solid foundation of well-tested code and a rich set of features. This makes it an excellent choice for developers and organizations seeking to build robust and adaptable data pipelines.
Koheesio is not a workflow orchestration tool. It does not serve the same purpose as tools like Luigi, Apache Airflow, or Databricks workflows, which are designed to manage complex computational workflows and generate DAGs (Directed Acyclic Graphs).
Instead, Koheesio is focused on providing a robust, modular, and testable framework for data tasks. It's designed to make it easier to write, maintain, and test data processing code in Python, with a strong emphasis on modularity, reusability, and error handling.
If you're looking for a tool to orchestrate complex workflows or manage dependencies between different tasks, you might want to consider dedicated workflow orchestration tools.
The core strength of Koheesio lies in its focus on the individual tasks within those workflows. It's all about making these tasks as robust, repeatable, and maintainable as possible. Koheesio aims to break down tasks into small, manageable units of work that can be easily tested, reused, and composed into larger workflows orchestrated with other tools or frameworks (such as Apache Airflow, Luigi, or Databricks Workflows).
By using Koheesio, you can ensure that your data tasks are resilient, observable, and repeatable, adhering to good software engineering practices. This makes your data pipelines more reliable and easier to maintain, ultimately leading to more efficient and effective data processing.
Koheesio encapsulates years of software and data engineering expertise. It fosters a collaborative and innovative community, setting itself apart with its unique design and focus on data pipelines, data transformation, ETL jobs, data validation, and large-scale data processing.
The core components of Koheesio are designed to bring strong software engineering principles to data engineering.
'Steps' break down tasks and workflows into manageable, reusable, and testable units. Each 'Step' comes with built-in logging, providing transparency and traceability. The 'Context' component allows for flexible customization of task behavior, making it adaptable to various data processing needs.
In essence, Koheesio is a comprehensive solution for data engineering challenges, designed with the principles of modularity, reusability, testability, and transparency at its core. It aims to provide a rich set of features including utilities, readers, writers, and transformations for any type of data processing. It is not in competition with other libraries, but rather aims to offer wide-ranging support and focus on utility in a multitude of scenarios. Our preference is for integration, not competition.
We invite contributions from all, promoting collaboration and innovation in the data engineering community.
The libraries listed under this section are primarily focused on Machine Learning (ML) workflows. They provide various functionalities, from orchestrating ML and data processing workflows, simplifying the deployment of ML workflows on Kubernetes, to managing the end-to-end ML lifecycle. While these libraries have a strong emphasis on ML, Koheesio is a more general data pipeline framework. It is designed to handle a variety of data processing tasks, not exclusively focused on ML. This makes Koheesio a versatile choice for data pipeline construction, regardless of whether the pipeline involves ML tasks or not.
The libraries listed under this section are primarily focused on workflow orchestration. They provide various functionalities, from authoring, scheduling, and monitoring workflows, to building complex pipelines of batch jobs, and creating and executing Directed Acyclic Graphs (DAGs). Some of these libraries are designed for modern infrastructure and powered by open-source workflow engines, while others use a Python-style language for defining workflows. While these libraries have a strong emphasis on workflow orchestration, Koheesio is a more general data pipeline framework. It is designed to handle a variety of data processing tasks, not limited to workflow orchestration.Ccode written with Koheesio is often compatible with these orchestration engines. This makes Koheesio a versatile choice for data pipeline construction, regardless of how the pipeline orchestration is set up.
The libraries listed under this section offer a variety of unique functionalities, from parallel and distributed computing, to SQL-first transformation workflows, to data versioning and lineage, to data relation definition and manipulation, and data warehouse management. Some of these libraries are designed for specific tasks such as transforming data in warehouses using SQL, building concurrent, multi-stage data ingestion and processing pipelines, or orchestrating parallel jobs on Kubernetes.
Here are the 3 core components included in Koheesio:
You can install Koheesio using either pip, hatch, or poetry.
To install Koheesio using pip, run the following command in your terminal:
pip install koheesio
If you're using Hatch for package management, you can add Koheesio to your project by simply adding koheesio to your
pyproject.toml
.
[dependencies]
koheesio = "<version>"
If you're using poetry for package management, you can add Koheesio to your project with the following command:
poetry add koheesio
or add the following line to your pyproject.toml
(under [tool.poetry.dependencies]
), making sure to replace
...
with the version you want to have installed:
koheesio = {version = "..."}
Koheesio also provides some additional features that can be useful in certain scenarios. We call these 'integrations'. With an integration we mean a module that requires additional dependencies to be installed.
Extras can be added by adding extras=['name_of_the_extra']
(poetry) or koheesio[name_of_the_extra]
(pip/hatch) to
the pyproject.toml
entry mentioned above or installing through pip.
koheesio.steps.integration.spark.dq.spark_expectations
module; installable through the se
extra.
Box:
Available through the koheesio.integration.box
module; installable through the box
extra.
SFTP:
Available through the koheesio.integration.spark.sftp
module; installable through the sftp
extra.
Note:
Some of the steps require extra dependencies. See the Extras section for additional info.
Extras can be done by addingfeatures=['name_of_the_extra']
to the toml entry mentioned above
We welcome contributions to our project! Here's a brief overview of our development process:
Code Standards: We use pylint
, black
, and mypy
to maintain code standards. Please ensure your code passes
these checks by running make check
. No errors or warnings should be reported by the linter before you submit a pull
request.
Testing: We use pytest
for testing. Run the tests with make test
and ensure all tests pass before submitting
a pull request.
Release Process: We aim for frequent releases. Typically when we have a new feature or bugfix, a developer with admin rights will create a new release on GitHub and publish the new version to PyPI.
For more detailed information, please refer to our contribution guidelines. We also adhere to Nike's Code of Conduct.
FAQs
The steps-based Koheesio framework
We found that koheesio demonstrated a healthy version release cadence and project activity because the last version was released less than a year ago. It has 1 open source maintainer collaborating on the project.
Did you know?
Socket for GitHub automatically highlights issues in each pull request and monitors the health of all your open source dependencies. Discover the contents of your packages and block harmful activity before you install or update your dependencies.
Research
Security News
Socket’s threat research team has detected six malicious npm packages typosquatting popular libraries to insert SSH backdoors.
Security News
MITRE's 2024 CWE Top 25 highlights critical software vulnerabilities like XSS, SQL Injection, and CSRF, reflecting shifts due to a refined ranking methodology.
Security News
In this segment of the Risky Business podcast, Feross Aboukhadijeh and Patrick Gray discuss the challenges of tracking malware discovered in open source softare.