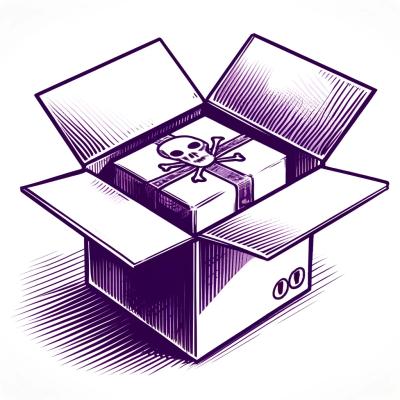
Security News
Research
Data Theft Repackaged: A Case Study in Malicious Wrapper Packages on npm
The Socket Research Team breaks down a malicious wrapper package that uses obfuscation to harvest credentials and exfiltrate sensitive data.
llama-index-llms-bedrock-converse
Advanced tools
%pip install llama-index-llms-bedrock-converse
!pip install llama-index
from llama_index.llms.bedrock_converse import BedrockConverse
# Set your AWS profile name
profile_name = "Your aws profile name"
# Simple completion call
resp = BedrockConverse(
model="anthropic.claude-3-haiku-20240307-v1:0",
profile_name=profile_name,
).complete("Paul Graham is ")
print(resp)
from llama_index.core.llms import ChatMessage
from llama_index.llms.bedrock_converse import BedrockConverse
messages = [
ChatMessage(
role="system", content="You are a pirate with a colorful personality"
),
ChatMessage(role="user", content="Tell me a story"),
]
resp = BedrockConverse(
model="anthropic.claude-3-haiku-20240307-v1:0",
profile_name=profile_name,
).chat(messages)
print(resp)
# Using stream_complete endpoint
from llama_index.llms.bedrock_converse import BedrockConverse
llm = BedrockConverse(
model="anthropic.claude-3-haiku-20240307-v1:0",
profile_name=profile_name,
)
resp = llm.stream_complete("Paul Graham is ")
for r in resp:
print(r.delta, end="")
# Using stream_chat endpoint
from llama_index.llms.bedrock_converse import BedrockConverse
llm = BedrockConverse(
model="anthropic.claude-3-haiku-20240307-v1:0",
profile_name=profile_name,
)
messages = [
ChatMessage(
role="system", content="You are a pirate with a colorful personality"
),
ChatMessage(role="user", content="Tell me a story"),
]
resp = llm.stream_chat(messages)
for r in resp:
print(r.delta, end="")
from llama_index.llms.bedrock_converse import BedrockConverse
llm = BedrockConverse(
model="anthropic.claude-3-haiku-20240307-v1:0",
profile_name=profile_name,
)
resp = llm.complete("Paul Graham is ")
print(resp)
from llama_index.llms.bedrock_converse import BedrockConverse
llm = BedrockConverse(
model="anthropic.claude-3-haiku-20240307-v1:0",
aws_access_key_id="AWS Access Key ID to use",
aws_secret_access_key="AWS Secret Access Key to use",
aws_session_token="AWS Session Token to use",
region_name="AWS Region to use, eg. us-east-1",
)
resp = llm.complete("Paul Graham is ")
print(resp)
# Claude, Command, and Mistral Large models support native function calling through AWS Bedrock Converse.
# There is seamless integration with LlamaIndex tools through the predict_and_call function on the LLM.
from llama_index.llms.bedrock_converse import BedrockConverse
from llama_index.core.tools import FunctionTool
# Define some functions
def multiply(a: int, b: int) -> int:
"""Multiply two integers and return the result"""
return a * b
def mystery(a: int, b: int) -> int:
"""Mystery function on two integers."""
return a * b + a + b
# Create tools from functions
mystery_tool = FunctionTool.from_defaults(fn=mystery)
multiply_tool = FunctionTool.from_defaults(fn=multiply)
# Instantiate the BedrockConverse model
llm = BedrockConverse(
model="anthropic.claude-3-haiku-20240307-v1:0",
profile_name=profile_name,
)
# Use function tools with the LLM
response = llm.predict_and_call(
[mystery_tool, multiply_tool],
user_msg="What happens if I run the mystery function on 5 and 7",
)
print(str(response))
response = llm.predict_and_call(
[mystery_tool, multiply_tool],
user_msg=(
"""What happens if I run the mystery function on the following pairs of numbers?
Generate a separate result for each row:
- 1 and 2
- 8 and 4
- 100 and 20
NOTE: you need to run the mystery function for all of the pairs above at the same time"""
),
allow_parallel_tool_calls=True,
)
print(str(response))
for s in response.sources:
print(f"Name: {s.tool_name}, Input: {s.raw_input}, Output: {str(s)}")
from llama_index.llms.bedrock_converse import BedrockConverse
llm = BedrockConverse(
model="anthropic.claude-3-haiku-20240307-v1:0",
aws_access_key_id="AWS Access Key ID to use",
aws_secret_access_key="AWS Secret Access Key to use",
aws_session_token="AWS Session Token to use",
region_name="AWS Region to use, eg. us-east-1",
)
# Use async complete
resp = await llm.acomplete("Paul Graham is ")
print(resp)
https://docs.llamaindex.ai/en/stable/examples/llm/bedrock_converse/
FAQs
llama-index llms bedrock converse integration
We found that llama-index-llms-bedrock-converse demonstrated a healthy version release cadence and project activity because the last version was released less than a year ago. It has 1 open source maintainer collaborating on the project.
Did you know?
Socket for GitHub automatically highlights issues in each pull request and monitors the health of all your open source dependencies. Discover the contents of your packages and block harmful activity before you install or update your dependencies.
Security News
Research
The Socket Research Team breaks down a malicious wrapper package that uses obfuscation to harvest credentials and exfiltrate sensitive data.
Research
Security News
Attackers used a malicious npm package typosquatting a popular ESLint plugin to steal sensitive data, execute commands, and exploit developer systems.
Security News
The Ultralytics' PyPI Package was compromised four times in one weekend through GitHub Actions cache poisoning and failure to rotate previously compromised API tokens.