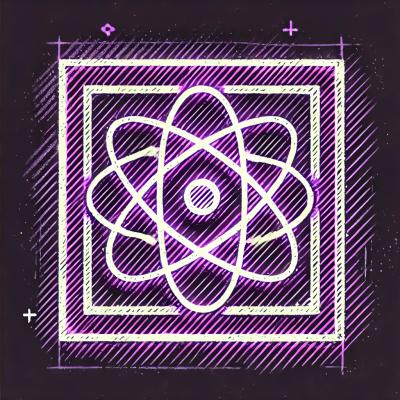
Security News
Create React App Officially Deprecated Amid React 19 Compatibility Issues
Create React App is officially deprecated due to React 19 issues and lack of maintenance—developers should switch to Vite or other modern alternatives.
A library for compressing large language models utilizing the latest techniques and research in the field for both training aware and post training techniques. The library is designed to be flexible and easy to use on top of PyTorch and HuggingFace Transformers, allowing for quick experimentation.
llmcompressor
is an easy-to-use library for optimizing models for deployment with vllm
, including:
safetensors
-based file format compatible with vllm
accelerate
✨ Read the announcement blog here! ✨
pip install llmcompressor
Applying quantization with llmcompressor
:
int8
fp8
int4
Deep dives into advanced usage of llmcompressor
:
Let's quantize TinyLlama
with 8 bit weights and activations using the GPTQ
and SmoothQuant
algorithms.
Note that the model can be swapped for a local or remote HF-compatible checkpoint and the recipe
may be changed to target different quantization algorithms or formats.
Quantization is applied by selecting an algorithm and calling the oneshot
API.
from llmcompressor.modifiers.quantization import GPTQModifier
from llmcompressor.modifiers.smoothquant import SmoothQuantModifier
from llmcompressor.transformers import oneshot
from transformers import AutoModelForCausalLM
# Select quantization algorithm. In this case, we:
# * apply SmoothQuant to make the activations easier to quantize
# * quantize the weights to int8 with GPTQ (static per channel)
# * quantize the activations to int8 (dynamic per token)
recipe = [
SmoothQuantModifier(smoothing_strength=0.8),
GPTQModifier(scheme="W8A8", targets="Linear", ignore=["lm_head"]),
]
# Apply quantization using the built in open_platypus dataset.
# * See examples for demos showing how to pass a custom calibration set
oneshot(
model="TinyLlama/TinyLlama-1.1B-Chat-v1.0",
dataset="open_platypus",
recipe=recipe,
output_dir="TinyLlama-1.1B-Chat-v1.0-INT8",
max_seq_length=2048,
num_calibration_samples=512,
)
The checkpoints created by llmcompressor
can be loaded and run in vllm
:
Install:
pip install vllm
Run:
from vllm import LLM
model = LLM("TinyLlama-1.1B-Chat-v1.0-INT8")
output = model.generate("My name is")
FAQs
A library for compressing large language models utilizing the latest techniques and research in the field for both training aware and post training techniques. The library is designed to be flexible and easy to use on top of PyTorch and HuggingFace Transformers, allowing for quick experimentation.
We found that llmcompressor demonstrated a healthy version release cadence and project activity because the last version was released less than a year ago. It has 1 open source maintainer collaborating on the project.
Did you know?
Socket for GitHub automatically highlights issues in each pull request and monitors the health of all your open source dependencies. Discover the contents of your packages and block harmful activity before you install or update your dependencies.
Security News
Create React App is officially deprecated due to React 19 issues and lack of maintenance—developers should switch to Vite or other modern alternatives.
Security News
Oracle seeks to dismiss fraud claims in the JavaScript trademark dispute, delaying the case and avoiding questions about its right to the name.
Security News
The Linux Foundation is warning open source developers that compliance with global sanctions is mandatory, highlighting legal risks and restrictions on contributions.