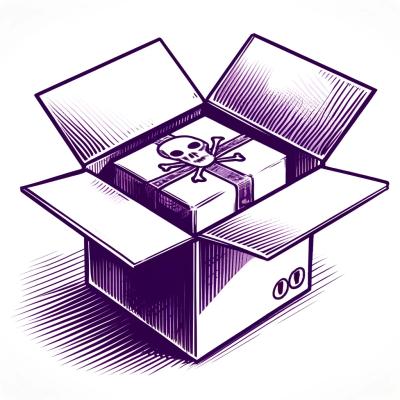
Security News
Research
Data Theft Repackaged: A Case Study in Malicious Wrapper Packages on npm
The Socket Research Team breaks down a malicious wrapper package that uses obfuscation to harvest credentials and exfiltrate sensitive data.
minisom2onnx
is a Python library for converting MiniSom models to ONNX (Open Neural Network Exchange) format, allowing for deployment in various environments. It provides flexibility to include additional information, such as quantization error thresholds and label mappings.
You can install the library using pip
:
pip install minisom2onnx
The to_onnx
function converts a trained MiniSom model to an ONNX format.
to_onnx(
model,
name: Optional[str] = None,
description: Optional[str] = None,
threshold: Optional[float] = None,
labels: Optional[np.ndarray] = None,
outputs: Optional[List[str]] = ['winner'],
properties: Optional[Dict[str, str]] = {},
opset: Optional[int] = 18,
) -> ModelProto
By default, the following outputs are available:
weights
: The original weights of the MiniSom model.distance
: The distance between each input sample and the weights vector of the winning neuron.quantization
: The code book BMU (weights vector of the winning neuron) for each sample in the data.quantization_error
: The quantization error, calculated as the distance between each input sample and its best matching unit.winner
: The coordinates of the BMU on the SOM grid.Additional outputs are available based on the optional parameters:
outlier
: A binary indicator of whether the quantization error exceeds the provided threshold. This output is only available if the threshold parameter is specified.class
: The label of the BMU. This output is only available if the labels parameter is provided.NOTE: The MiniSom model supports several distance functions, including
euclidean
,cosine
,manhattan
, andchebyshev
. However, the ONNX operatorCDist
currently has an implementation only foreuclidean
distance. As a result, while the model can be exported to ONNX successfully, onnxruntime will fail if a distance function other thaneuclidean
(default) is used.
Additionally, MiniSom allows for custom distance functions. If a custom distance function is employed in the model, theto_onnx
with throw an ValueError: Unsupported activation_distance
For reliable inference, it is recommended to use theeuclidean
distance function with your MiniSom model when exporting to ONNX.
Here’s a basic example of how to use minisom2onnx
to convert a trained MiniSom model to ONNX format:
from minisom import MiniSom
import numpy as np
import random
from minisom2onnx import to_onnx
data = np.random.rand(100, 4)
# Create and train a MiniSom model
som = MiniSom(10, 10, data.shape[1], sigma=0.3, learning_rate=0.5)
som.random_weights_init(data)
som.train_random(data, 100)
# Convert the model to ONNX
onnx_model = to_onnx(som, name="SOMModel")
# Save the model
import onnx
onnx.save(onnx_model, 'som_model.onnx')
To include label information in your ONNX model, you can provide labels
during conversion. Here’s an example:
from minisom import MiniSom
import numpy as np
import random
from minisom2onnx import to_onnx
dim = 10
data = np.random.rand(100, 4)
target = [random.randint(1, 2) for i in range(100)]
# Create and train a MiniSom model
som = MiniSom(dim, dim, data.shape[1], sigma=3, learning_rate=0.5, neighborhood_function='triangle', random_seed=10)
som.pca_weights_init(data)
som.train(data, 1000, random_order=True, use_epochs=True)
default_label = 0
labels = np.full((dim, dim), fill_value=default_label, dtype=int)
for position, counter in som.labels_map(data, target).items():
labels[position] = max(counter, key=counter.get)
# Convert the model to ONNX
onnx_model = to_onnx(som, name="SOMClassifier", labels=labels, outputs=["class"])
# Save the model
import onnx
onnx.save(onnx_model, 'som_model.onnx')
If you want to include threshold-based outlier detection in your ONNX model, you can specify a threshold
. Here’s how:
from minisom import MiniSom
import numpy as np
import random
from minisom2onnx import to_onnx
dim = 10
data = np.random.rand(100, 4)
# Create and train a MiniSom model
som = MiniSom(dim, dim, data.shape[1], sigma=3, learning_rate=0.5, neighborhood_function='triangle', random_seed=10)
som.train(data, 1000, random_order=True, use_epochs=True)
quantization_errors = np.array([som.quantization_error([x]) for x in data])
threshold = np.percentile(quantization_errors, 95)
# Convert the model to ONNX
onnx_model = to_onnx(som, name="SOMOutlier", threshold=threshold, outputs=["outlier"])
# Save the model
import onnx
onnx.save(onnx_model, 'som_model.onnx')
FAQs
A library to convert MiniSom models to ONNX format
We found that minisom2onnx demonstrated a healthy version release cadence and project activity because the last version was released less than a year ago. It has 1 open source maintainer collaborating on the project.
Did you know?
Socket for GitHub automatically highlights issues in each pull request and monitors the health of all your open source dependencies. Discover the contents of your packages and block harmful activity before you install or update your dependencies.
Security News
Research
The Socket Research Team breaks down a malicious wrapper package that uses obfuscation to harvest credentials and exfiltrate sensitive data.
Research
Security News
Attackers used a malicious npm package typosquatting a popular ESLint plugin to steal sensitive data, execute commands, and exploit developer systems.
Security News
The Ultralytics' PyPI Package was compromised four times in one weekend through GitHub Actions cache poisoning and failure to rotate previously compromised API tokens.