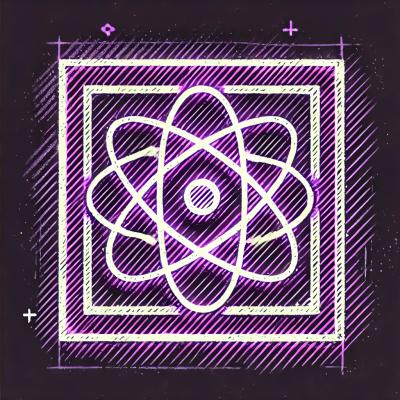
Security News
Create React App Officially Deprecated Amid React 19 Compatibility Issues
Create React App is officially deprecated due to React 19 issues and lack of maintenance—developers should switch to Vite or other modern alternatives.
numba-kdtree
provides a fast KDTree implementation for numba
CPU functions based on scipys ckdtree
.
The provided KDTree
class is usable from both python and numba nopython functions calling directly into C code for performance critical sections utilizing a ctypes-like interface.
Once the KDTree
class is compiled by numba
, it is just as fast as the original scipy version.
Note: Currently only a basic subset of the original ckdtree
interface is implemented.
pip install numba-kdtree
git clone https://github.com/mortacious/numba-kdtree.git
cd numba-kdtree
python -m pip install
import numpy as np
from numba_kdtree import KDTree
data = np.random.random(3_000_000).reshape(-1, 3)
kdtree = KDTree(data, leafsize=10)
# compute the nearest neighbors of the first 100 points
distances, indices = kdtree.query(data[:100], k=30)
# compute all points in a 0.5 radius around the first 100 points and return it's sorted indices
indices = kdtree.query_radius(data[:100], r=0.5, return_sorted=True)
The KDTree
can be freely passed into a numba
nopython function or constructed directly within it:
import numpy as np
from numba import njit
from numba_kdtree import KDTree
def numba_function_with_kdtree(kdtree, data):
for i in range(data.shape[0]):
distances, indices = kdtree.query(data[i], k=30)
#<Use the computed neighbors
data = np.random.random(3_000_000).reshape(-1, 3)
kdtree = KDTree(data, leafsize=10)
numba_function_with_kdtree(kdtree)
import numpy as np
from numba import njit
from numba_kdtree import KDTree
def numba_function_constructing_kdtree(kdtree, data):
kdtree = KDTree(data, leafsize=10)
for i in range(data.shape[0]):
distances, indices = kdtree.query(data[i], k=30)
#<Use the computed neighbors
data = np.random.random(10_000_000).reshape(-1, 10)
numba_function_constructing_kdtree(data)
Additionally, the KDTree
object can also be serialized via pickle:
import pickle
data = np.random.random(3_000_000).reshape(-1, 3)
kdtree = KDTree(data, leafsize=10)
# pass the tree through pickle
# Note: This also copies the data array preserving it's integrity
serialized_tree = pickle.dumps(kdtree)
# The copied data array is now owned by the restored tree
restored_kdtree = pickle.loads(serialized_tree)
k = 10
# query the old tree
dd, ii, nn = kdtree.query_parallel(data[:100], k=k)
# query the new tree
dd_r, ii_r, nn_r = restored_kdtree.query_parallel(data[:100], k=k)
ckdtree
interfaceFAQs
A kdtree implementation for numba.
We found that numba-kdtree demonstrated a healthy version release cadence and project activity because the last version was released less than a year ago. It has 1 open source maintainer collaborating on the project.
Did you know?
Socket for GitHub automatically highlights issues in each pull request and monitors the health of all your open source dependencies. Discover the contents of your packages and block harmful activity before you install or update your dependencies.
Security News
Create React App is officially deprecated due to React 19 issues and lack of maintenance—developers should switch to Vite or other modern alternatives.
Security News
Oracle seeks to dismiss fraud claims in the JavaScript trademark dispute, delaying the case and avoiding questions about its right to the name.
Security News
The Linux Foundation is warning open source developers that compliance with global sanctions is mandatory, highlighting legal risks and restrictions on contributions.