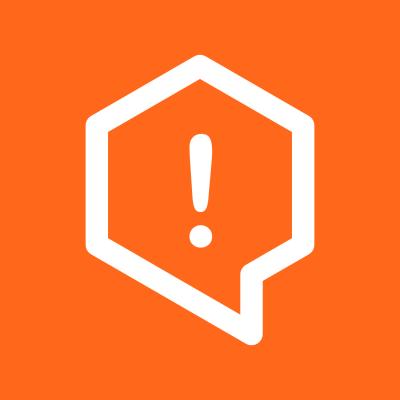
Security News
Fluent Assertions Faces Backlash After Abandoning Open Source Licensing
Fluent Assertions is facing backlash after dropping the Apache license for a commercial model, leaving users blindsided and questioning contributor rights.
nutpie can be installed using Conda or Mamba from conda-forge with
mamba install -c conda-forge nutpie
Or using pip:
pip install nutpie
To install it from source, install a Rust compiler and maturin and then
maturin develop --release
If you want to use the nightly SIMD implementation for some of the math functions,
switch to Rust nightly and then install with the simd_support
feature in then
nutpie directory:
rustup override set nightly
maturin develop --release --features=simd_support
First, PyMC and Numba need to be installed, for example using
mamba install -c conda-forge pymc numba
We need to create a model:
import pymc as pm
import numpy as np
import nutpie
import pandas as pd
import seaborn as sns
# Load the radon dataset
data = pd.read_csv(pm.get_data("radon.csv"))
data["log_radon"] = data["log_radon"].astype(np.float64)
county_idx, counties = pd.factorize(data.county)
coords = {"county": counties, "obs_id": np.arange(len(county_idx))}
# Create a simple hierarchical model for the radon dataset
with pm.Model(coords=coords, check_bounds=False) as pymc_model:
intercept = pm.Normal("intercept", sigma=10)
# County effects
raw = pm.ZeroSumNormal("county_raw", dims="county")
sd = pm.HalfNormal("county_sd")
county_effect = pm.Deterministic("county_effect", raw * sd, dims="county")
# Global floor effect
floor_effect = pm.Normal("floor_effect", sigma=2)
# County:floor interaction
raw = pm.ZeroSumNormal("county_floor_raw", dims="county")
sd = pm.HalfNormal("county_floor_sd")
county_floor_effect = pm.Deterministic(
"county_floor_effect", raw * sd, dims="county"
)
mu = (
intercept
+ county_effect[county_idx]
+ floor_effect * data.floor.values
+ county_floor_effect[county_idx] * data.floor.values
)
sigma = pm.HalfNormal("sigma", sigma=1.5)
pm.Normal(
"log_radon", mu=mu, sigma=sigma, observed=data.log_radon.values, dims="obs_id"
)
We then compile this model and sample form the posterior:
compiled_model = nutpie.compile_pymc_model(pymc_model)
trace_pymc = nutpie.sample(compiled_model)
trace_pymc
now contains an ArviZ InferenceData
object, including sampling
statistics and the posterior of the variables defined above.
We can also control the sampler in a non-blocking way:
# The sampler will now run the the background
sampler = nutpie.sample(compiled_model, blocking=False)
# Pause and resume the sampling
sampler.pause()
sampler.resume()
# Wait for the sampler to finish (up to timeout seconds)
# sampler.wait(timeout=0.1)
# or we can also abort the sampler (and return the incomplete trace)
incomplete_trace = sampler.abort()
# or cancel and discard all progress:
sampler.cancel()
In order to sample from Stan model, bridgestan
needs to be installed.
A pip package is available, but right now this can not be installed using Conda.
pip install bridgestan
When we install nutpie with pip, we can also specify that we want optional dependencies for Stan models using
pip install 'nutpie[stan]'
In addition, a C++ compiler needs to be available. For details see the Stan docs.
We can then compile a Stan model, and sample using nutpie:
import nutpie
code = """
data {
real mu;
}
parameters {
real x;
}
model {
x ~ normal(mu, 1);
}
"""
compiled = nutpie.compile_stan_model(code=code)
# Provide data
compiled = compiled.with_data(mu=3.)
trace = nutpie.sample(compiled)
nutpie uses nuts-rs
, a library written in Rust, that implements NUTS as in
PyMC and Stan, but with a slightly different mass matrix tuning method as
those. It often produces a higher effective sample size per gradient
evaluation, and tends to converge faster and with fewer gradient evaluation.
FAQs
Sample Stan or PyMC models
We found that nutpie demonstrated a healthy version release cadence and project activity because the last version was released less than a year ago. It has 2 open source maintainers collaborating on the project.
Did you know?
Socket for GitHub automatically highlights issues in each pull request and monitors the health of all your open source dependencies. Discover the contents of your packages and block harmful activity before you install or update your dependencies.
Security News
Fluent Assertions is facing backlash after dropping the Apache license for a commercial model, leaving users blindsided and questioning contributor rights.
Research
Security News
Socket researchers uncover the risks of a malicious Python package targeting Discord developers.
Security News
The UK is proposing a bold ban on ransomware payments by public entities to disrupt cybercrime, protect critical services, and lead global cybersecurity efforts.