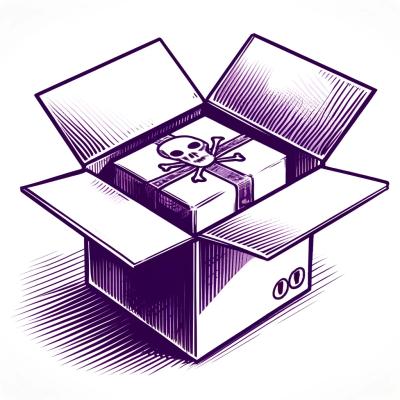
Security News
Research
Data Theft Repackaged: A Case Study in Malicious Wrapper Packages on npm
The Socket Research Team breaks down a malicious wrapper package that uses obfuscation to harvest credentials and exfiltrate sensitive data.
🤗 Optimum Quanto is a pytorch quantization backend for optimum.
It has been designed with versatility and simplicity in mind:
weight_only
and 🤗 safetensors
,Features yet to be implemented:
In a nutshell:
int8
/float8
weights and float8
activations are very close to the full-precision models,The paragraph below is just an example. Please refer to the bench
folder for detailed results per use-case of model.
Optimum Quanto is available as a pip package.
pip install optimum-quanto
optimum-quanto
provides helper classes to quantize, save and reload Hugging Face quantized models.
The first step is to quantize the model
from transformers import AutoModelForCausalLM
from optimum.quanto import QuantizedModelForCausalLM, qint4
model = AutoModelForCausalLM.from_pretrained('meta-llama/Meta-Llama-3-8B')
qmodel = QuantizedModelForCausalLM.quantize(model, weights=qint4, exclude='lm_head')
Note: the model quantized weights will be frozen. If you want to keep them unfrozen to train them you need to use optimum.quanto.quantize
directly.
The quantized model can be saved using save_pretrained
:
qmodel.save_pretrained('./Llama-3-8B-quantized')
It can later be reloaded using from_pretrained
:
from optimum.quanto import QuantizedModelForCausalLM
qmodel = QuantizedModelForCausalLM.from_pretrained('Llama-3-8B-quantized')
You can quantize any of the submodels inside a diffusers pipeline and seamlessly include them later in another pipeline.
Here we quantize the transformer
of a Pixart
pipeline.
from diffusers import PixArtTransformer2DModel
from optimum.quanto import QuantizedPixArtTransformer2DModel, qfloat8
model = PixArtTransformer2DModel.from_pretrained("PixArt-alpha/PixArt-Sigma-XL-2-1024-MS", subfolder="transformer")
qmodel = QuantizedPixArtTransformer2DModel.quantize(model, weights=qfloat8)
qmodel.save_pretrained("./pixart-sigma-fp8")
Later, we can reload the quantized model and recreate the pipeline:
from diffusers import PixArtTransformer2DModel
from optimum.quanto import QuantizedPixArtTransformer2DModel
transformer = QuantizedPixArtTransformer2DModel.from_pretrained("./pixart-sigma-fp8")
transformer.to(device="cuda")
pipe = PixArtSigmaPipeline.from_pretrained(
"PixArt-alpha/PixArt-Sigma-XL-2-1024-MS",
transformer=None,
torch_dtype=torch.float16,
).to("cuda")
pipe.transformer = transformer
One thing to keep in mind when using the low-level quanto API is that by default models weights are dynamically quantized: an explicit call must be made to 'freeze' the quantized weights.
A typical quantization workflow would consist of the following steps:
1. Quantize
The first step converts a standard float model into a dynamically quantized model.
from optimum.quanto import quantize, qint8
quantize(model, weights=qint8, activations=qint8)
At this stage, only the inference of the model is modified to dynamically quantize the weights.
2. Calibrate (optional if activations are not quantized)
Quanto supports a calibration mode that allows to record the activation ranges while passing representative samples through the quantized model.
from optimum.quanto import Calibration
with Calibration(momentum=0.9):
model(samples)
This automatically activates the quantization of the activations in the quantized modules.
3. Tune, aka Quantization-Aware-Training (optional)
If the performance of the model degrades too much, one can tune it for a few epochs to recover the float model performance.
import torch
model.train()
for batch_idx, (data, target) in enumerate(train_loader):
data, target = data.to(device), target.to(device)
optimizer.zero_grad()
output = model(data).dequantize()
loss = torch.nn.functional.nll_loss(output, target)
loss.backward()
optimizer.step()
4. Freeze integer weights
When freezing a model, its float weights are replaced by quantized integer weights.
from optimum.quanto import freeze
freeze(model)
5. Serialize quantized model
Quantized models weights can be serialized to a state_dict
, and saved to a file.
Both pickle
and safetensors
(recommended) are supported.
from safetensors.torch import save_file
save_file(model.state_dict(), 'model.safetensors')
In order to be able to reload these weights, you also need to store the quantized model quantization map.
import json
from optimum.quanto import quantization_map
with open('quantization_map.json', 'w') as f:
json.dump(quantization_map(model), f)
5. Reload a quantized model
A serialized quantized model can be reloaded from a state_dict
and a quantization_map
using the requantize
helper.
Note that you need first to instantiate an empty model.
import json
from safetensors.torch import load_file
from optimum.quanto import requantize
state_dict = load_file('model.safetensors')
with open('quantization_map.json', 'r') as f:
quantization_map = json.load(f)
# Create an empty model from your modeling code and requantize it
with torch.device('meta'):
new_model = ...
requantize(new_model, state_dict, quantization_map, device=torch.device('cuda'))
Please refer to the examples for instantiations of that workflow.
At the heart of quanto is a Tensor subclass that corresponds to:
For floating-point destination types, the mapping is done by the native pytorch cast (i.e. Tensor.to()
).
For integer destination types, the mapping is a simple rounding operation (i.e. torch.round()
).
The goal of the projection is to increase the accuracy of the conversion by minimizing the number of:
The projection is symmetric per-tensor or per-channel for int8
and float8
, and group-wise affine (with a shift or 'zero-point') for lower bitwidth.
One of the benefits of using a lower-bitwidth representation is that you will be able to take advantage of accelerated operations for the destination type, which is typically faster than their higher precision equivalents.
Quanto does not support the conversion of a Tensor using mixed destination types.
Quanto provides a generic mechanism to replace torch
modules by optimum-quanto
modules that are able to process quanto tensors.
optimum-quanto
modules dynamically convert their weights until a model is frozen, which slows down inference a bit but is
required if the model needs to be tuned.
Weights are usually quantized per-channel along the first dimension (output features).
Biases are not converted to preserve the accuracy of a typical addmm
operation.
Explanation: to be consistent with the unquantized arithmetic operations, biases would need to be quantized with a scale that
is equal to the product of the input and weight scales, which leads to a ridiculously small scale, and conversely
requires a very high bitwidth to avoid clipping. Typically, with int8
inputs and weights, biases would need to be quantized
with at least 12
bits, i.e. in int16
. Since most biases are today float16
, this is a waste of time.
Activations are dynamically quantized per-tensor using static scales (defaults to the range [-1, 1]
).
To preserve accuracy, the model needs to be calibrated to evaluate the best activation scales (using a momentum).
The following modules can be quantized:
Activations are always quantized per-tensor because most linear algebra operations in a model graph are not compatible
with per-axis inputs: you simply cannot add numbers that are not expressed in the same base (you cannot add apples and oranges
).
Weights involved in matrix multiplications are, on the contrary, always quantized along their first axis, because all output features are evaluated independently from one another.
The outputs of a quantized matrix multiplication will anyway always be dequantized, even if activations are quantized, because:
int32
or float32
) than the activation bitwidth (typically int8
or float8
),float
bias.Quantizing activations per-tensor to int8
can lead to serious quantization errors if the corresponding tensors contain large outlier values.
Typically, this will lead to quantized tensors with most values set to zero (except the outliers).
A possible solution to work around that issue is to 'smooth' the activations statically as illustrated by SmoothQuant. You can find a script to smooth some model architectures under external/smoothquant.
A better option is to represent activations using float8
.
FAQs
A pytorch quantization backend for optimum.
We found that optimum-quanto demonstrated a healthy version release cadence and project activity because the last version was released less than a year ago. It has 1 open source maintainer collaborating on the project.
Did you know?
Socket for GitHub automatically highlights issues in each pull request and monitors the health of all your open source dependencies. Discover the contents of your packages and block harmful activity before you install or update your dependencies.
Security News
Research
The Socket Research Team breaks down a malicious wrapper package that uses obfuscation to harvest credentials and exfiltrate sensitive data.
Research
Security News
Attackers used a malicious npm package typosquatting a popular ESLint plugin to steal sensitive data, execute commands, and exploit developer systems.
Security News
The Ultralytics' PyPI Package was compromised four times in one weekend through GitHub Actions cache poisoning and failure to rotate previously compromised API tokens.