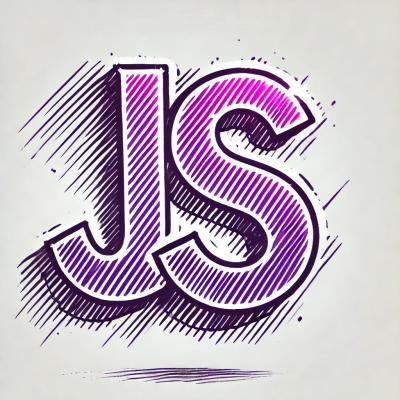
Security News
Oracle Drags Its Feet in the JavaScript Trademark Dispute
Oracle seeks to dismiss fraud claims in the JavaScript trademark dispute, delaying the case and avoiding questions about its right to the name.
Python Implementation of Parameter-exploring Policy Gradients [3] Evolution Strategy
pip3 install pepg-es
git clone https://github.com/goktug97/PEPG-ES
cd PEPG-ES
python3 setup.py install --user
I implemented several things differently from the original paper;
Refer to PEPG-ES/examples folder for more complete examples.
from pepg import PEPG, NeuralNetwork, Adam, sigmoid
import numpy as np
network = NeuralNetwork(input_size = 2, output_size = 1, hidden_sizes = [2],
hidden_activation = sigmoid,
output_activation = sigmoid)
# Adam Optimizer is the default optimizer, it is written for the example
optimizer_kwargs = {'beta_1': 0.9, 'beta_2': 0.999, 'epsilon': 1e-08} # Adam Parameters
es = PEPG(population_size = 100, theta_size = network.number_of_parameters,
mu_init = 0, sigma_init = 2.0,
mu_lr = 0.3, sigma_lr = 0.2, optimizer = Adam,
optimizer_kwargs = optimizer_kwargs)
truth_table = [[0, 1],[1, 0]]
solution_found = False
while True:
print(f'Step: {es.step}')
solutions = es.get_parameters()
rewards = []
for solution in solutions:
network.weights = solution
error = 0
for input_1 in range(len(truth_table)):
for input_2 in range(len(truth_table[0])):
output = int(round(network([input_1, input_2])[0]))
error += abs(truth_table[input_1][input_2] - output)
reward = (4 - error) ** 2
rewards.append(reward)
es.update(rewards)
if es.best_fitness == 16:
print('Solution Found')
print(f'Parameters: {es.best_theta}')
break
Step: 233
Step: 234
Step: 235
Step: 236
Step: 237
Solution Found
Parameters: [ 1.25863047 -0.73151503 -2.53377723 1.01802355 3.02723507 1.23112726
-2.00288859 -3.66789242 4.56593794]
es = PEPG(self, population_size, theta_size,
mu_init, sigma_init, mu_lr,
sigma_lr, l2_coeff = 0.005,
optimizer = Adam, optimizer_kwargs = {})
solutions = self.get_parameters(self)
self.update(self, rewards)
self.save_checkpoint(self)
es = PEPG.load_checkpoint(cls, filename)
self.save_best(self, filename)
theta, mu, sigma = PEPG.load_best(cls, filename)
NeuralNetwork(self, input_size, output_size, hidden_sizes = [],
hidden_activation = lambda x: x,
output_activation = lambda x: x,
bias = True):
self.save_network(self, filename)
network = NeuralNetwork.load_network(cls, filename)
from pepg import PEPG, Optimizer, NeuralNetwork
class CustomOptimizer(Optimizer):
def __init__(self, alpha, parameter, another_parameter):
self.alpha = alpha
self.parameter = parameter
self.another_parameter = another_parameter
def __call__(self, gradients):
gradients = (gradients + self.parameter) * self.another_parameter
return -self.alpha * gradients
network = NeuralNetwork(input_size = 2, output_size = 1)
optimizer_kwargs = {'parameter': 0.3, 'another_parameter': 0.2}
es = PEPG(population_size = 100, theta_size = network.number_of_parameters,
mu_init = 0.0, sigma_init = 2.0,
mu_lr = 0.3, sigma_lr = 0.2, optimizer = CustomOptimizer,
optimizer_kwargs = optimizer_kwargs)
FAQs
Python Implementation of Parameter-exploring Policy Gradients Evolution Strategy
We found that pepg-es demonstrated a healthy version release cadence and project activity because the last version was released less than a year ago. It has 1 open source maintainer collaborating on the project.
Did you know?
Socket for GitHub automatically highlights issues in each pull request and monitors the health of all your open source dependencies. Discover the contents of your packages and block harmful activity before you install or update your dependencies.
Security News
Oracle seeks to dismiss fraud claims in the JavaScript trademark dispute, delaying the case and avoiding questions about its right to the name.
Security News
The Linux Foundation is warning open source developers that compliance with global sanctions is mandatory, highlighting legal risks and restrictions on contributions.
Security News
Maven Central now validates Sigstore signatures, making it easier for developers to verify the provenance of Java packages.