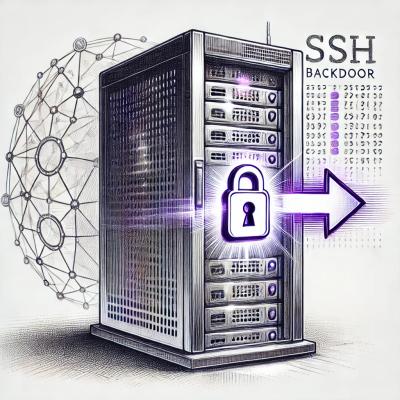
Research
Security News
Malicious npm Packages Inject SSH Backdoors via Typosquatted Libraries
Socket’s threat research team has detected six malicious npm packages typosquatting popular libraries to insert SSH backdoors.
perennityai-mp-extract
Advanced tools
A tool for extracting gesture landmarks and metadata from videos and saving them to disk using MediaPipe.
perennityai-mp-extract
is a tool designed to help developers and researchers easily extract hand, face, and pose landmarks from video files. By leveraging state-of-the-art MediaPipe models, this tool simplifies the process of obtaining precise gesture data, enabling the development of advanced touchless human-computer interaction (HCI) systems, gesture recognition models, and more.
With a user-friendly interface, perennityai-mp-extract allows users to process videos at a specified frame rate, providing flexibility to extract the data in various formats, including CSV, JSON, TFRecord, or Parquet. Whether you're working on sign language recognition, gesture-based controls, or any other HCI project, this tool streamlines the extraction of key visual data points, making it easier to integrate gesture analysis into your applications.
To use perennityai-mp-extract for extracting hand, face, and pose landmarks, the following data is required:
perennity-mp-extract/
│
├── src/ # All source code in the 'src' folder
│ └── perennity_mp_extract/ # Your main package/module
│ ├── __init__.py # Initializes the package/module
│ ├── main.py # Entry point for running the program
│ │
│ ├── utils/ # Utilities folder (for helpers, etc.)
│ │ ├── __init__.py # Initializes utils module
│ │ ├── csv_handler.py # Handles CSV file operations
│ │ ├── tfrecord_processor.py # Handles TFRecord file operations
│ │ ├── feature_header.py # The points labels for all landmarks
│ │ └── logger.py # Logger configuration and functions
│ │
│ └── data_extraction/ # Folder for data extraction related code
│ ├── __init__.py # Initializes data_extraction module
│ └── data_extractor.py # Main code for data extraction logic
│
├── tests/ # Unit tests and test files
│
├── MANIFEST.in # Specifies additional files for packaging
├── pyproject.toml # Build system configuration
├── setup.py # Package setup file
├── README.md # Project documentation
├── LICENSE # License file
└── requirements.txt # Dependencies for development
You can run the extraction tool directly from the command line. Use the following syntax:
pip install perennityai-mp-extract
# Ensure virtual environment path its in system path
perennityai-mp-extract --input_file https://www.example.com/23/23781.mp4 --output_dir ./path/to/storage --output_format csv --label gift --verbose DEBUG
Options:
--input_file: Specifies the path to the input video file. The tool supports multiple formats, including .mp4, .avi, .mov, .ogg, etc.
--output_dir: Indicates the directory where the processed output files (landmarks and metadata) will be saved.
--label: Allows the user to assign a label to the landmarks from the video, useful for classification or organization.
--output_format: Selects the output file format. Options include csv, tfrecord, or parquet, with csv as the default.
--frame_rate: Defines the frequency of frame processing. This can be adjusted for faster processing or more detailed frame-by-frame extraction.
--verbose: Controls the logging level for output, providing flexibility in logging output for debugging or streamlined logging.
Clone the repository and install required packages:
git clone https://github.com/perennityai/perennityai-mp-extract.git
cd perennityai-mp-extract
pip install -r requirements.txt
python main.py --input_file https://www.example.com/23/23781.mp4 --output_dir ./path/to/storage --output_format csv --label gift --verbose DEBUG
conda create --name mp-extract-env python=3.8
conda activate mp-extract-env
pip install perennityai-mp-extract
from perennityai_mp_extract.data_extraction.data_extractor import DataExtractor
# Usage Example: Load from a pretrained configuration file
try:
# Example of config file data:
# This configuration dictionary specifies the output directory, format, and verbosity level.
config = {
'output_dir': 'path/to/output_directory', # Directory to save extracted data
'output_format': 'tfrecord', # Format of output files (csv, tfrecord, or parquet)
'verbose': 'INFO', # Logging level to control output verbosity
}
# Initialize the DataExtractor with the configuration settings
extractor = DataExtractor(config=config)
# Alternatively, load configuration from a pretrained JSON config file
# extractor = DataExtractor.from_pretrained('path/to/config.json')
# Extract landmarks from a given video file
# The 'extract' method processes the video and saves the output based on config settings
animation = extractor.extract('your_video_url', 'label') # 'label' is a custom tag for the video
except FileNotFoundError as e:
# Handle cases where the configuration file is missing
print(f"Configuration loading failed: {e}")
perennity_mp_extract/
- __init__.py: Initializes the main perennity_mp_extract package for import.
main.py
- parse_arguments(): Parses command-line arguments for data extraction options.
- main(): Runs the DataExtractor with provided arguments to perform data extraction.
utils/
- __init__.py: Initializes the utils module for import.
- csv_handler.py: Manages CSV file read and write operations for landmarks data.
- tfrecord_processor.py: Handles saving and loading of TFRecord files.
- feature_header.py: Stores label names for all landmark points.
- logger.py: Configures and provides logging functionality for the package.
data_extraction/
- __init__.py: Initializes the data_extraction module for import.
- data_extractor.py: Core logic for extracting landmarks from video and saving in various formats.
This project is licensed under the MIT License. See the LICENSE file for details.
FAQs
A tool for extracting gesture landmarks and metadata from videos and saving them to disk using MediaPipe.
We found that perennityai-mp-extract demonstrated a healthy version release cadence and project activity because the last version was released less than a year ago. It has 1 open source maintainer collaborating on the project.
Did you know?
Socket for GitHub automatically highlights issues in each pull request and monitors the health of all your open source dependencies. Discover the contents of your packages and block harmful activity before you install or update your dependencies.
Research
Security News
Socket’s threat research team has detected six malicious npm packages typosquatting popular libraries to insert SSH backdoors.
Security News
MITRE's 2024 CWE Top 25 highlights critical software vulnerabilities like XSS, SQL Injection, and CSRF, reflecting shifts due to a refined ranking methodology.
Security News
In this segment of the Risky Business podcast, Feross Aboukhadijeh and Patrick Gray discuss the challenges of tracking malware discovered in open source softare.