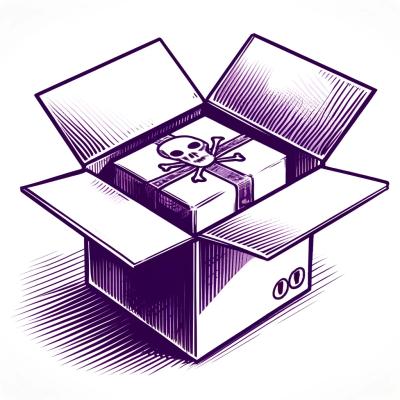
Security News
Research
Data Theft Repackaged: A Case Study in Malicious Wrapper Packages on npm
The Socket Research Team breaks down a malicious wrapper package that uses obfuscation to harvest credentials and exfiltrate sensitive data.
PigeonXT is an extention to the original Pigeon, created by Anastasis Germanidis. PigeonXT is a simple widget that lets you quickly annotate a dataset of unlabeled examples from the comfort of your Jupyter notebook.
PigeonXT currently support the following annotation tasks:
Anything that can be displayed on Jupyter
(text, images, audio, graphs, etc.) can be displayed by pigeon
by providing the appropriate display_fn
argument.
Additionally, custom hooks can be attached to each row update (example_process_fn
),
or when the annotating task is complete(final_process_fn
).
There is a full blog post on the usage of PigeonXT on Towards Data Science.
PigeonXT obviously needs a Jupyter Lab environment. Futhermore, it requires ipywidgets. The widget itself can be installed using pip:
pip install pigeonXT-jupyter
Currently, it is much easier to install due to Jupyterlab 3: To run the provided examples in a new environment using Conda:
conda create --name pigeon python=3.9
conda activate pigeon
pip install numpy pandas jupyterlab ipywidgets pigeonXT-jupyter
For an older Jupyterlab or any other trouble, please try the old method:
conda create --name pigeon python=3.7
conda activate pigeon
conda install nodejs
pip install numpy pandas jupyterlab ipywidgets
jupyter nbextension enable --py widgetsnbextension
jupyter labextension install @jupyter-widgets/jupyterlab-manager
pip install pigeonXT-jupyter
Starting Jupyter Lab environment:
jupyter lab
I have moved the development environment to Poetry. To create an identical environment use:
conda env create -f environment.yml
conda activate pigeonxt
poetry install
pre-commit install
Examples are also provided in the accompanying notebook.
Code:
import pandas as pd
import pigeonXT as pixt
annotations = pixt.annotate(
['I love this movie', 'I was really disappointed by the book'],
options=['positive', 'negative', 'inbetween']
)
Preview:
Code:
import pandas as pd
import pigeonXT as pixt
df = pd.DataFrame([
{'example': 'Star wars'},
{'example': 'The Positively True Adventures of the Alleged Texas Cheerleader-Murdering Mom'},
{'example': 'Eternal Sunshine of the Spotless Mind'},
{'example': 'Dr. Strangelove or: How I Learned to Stop Worrying and Love the Bomb'},
{'example': 'Killer klowns from outer space'},
])
labels = ['Adventure', 'Romance', 'Fantasy', 'Science fiction', 'Horror', 'Thriller']
annotations = pixt.annotate(
df,
options=labels,
task_type='multilabel-classification',
buttons_in_a_row=3,
reset_buttons_after_click=True,
include_next=True,
include_back=True,
)
Preview:
Code:
import pandas as pd
import pigeonXT as pixt
from IPython.display import display, Image
annotations = pixt.annotate(
['assets/img_example1.jpg', 'assets/img_example2.jpg'],
options=['cat', 'dog', 'horse'],
display_fn=lambda filename: display(Image(filename))
)
Preview:
Code:
import pandas as pd
import pigeonXT as pixt
from IPython.display import Audio
annotations = pixt.annotate(
['assets/audio_1.mp3', 'assets/audio_2.mp3'],
task_type='regression',
options=(1,5,1),
display_fn=lambda filename: display(Audio(filename, autoplay=True))
)
annotations
Preview:
Code:
import pandas as pd
import numpy as np
from pathlib import Path
from pigeonXT import annotate
df = pd.DataFrame([
{'example': 'Star wars'},
{'example': 'The Positively True Adventures of the Alleged Texas Cheerleader-Murdering Mom'},
{'example': 'Eternal Sunshine of the Spotless Mind'},
{'example': 'Dr. Strangelove or: How I Learned to Stop Worrying and Love the Bomb'},
{'example': 'Killer klowns from outer space'},
])
labels = ['Adventure', 'Romance', 'Fantasy', 'Science fiction', 'Horror', 'Thriller']
shortLabels = ['A', 'R', 'F', 'SF', 'H', 'T']
df.to_csv('inputtestdata.csv', index=False)
def setLabels(labels, numClasses):
row = np.zeros([numClasses], dtype=np.uint8)
row[labels] = 1
return row
def labelPortion(
inputFile,
labels = ['yes', 'no'],
outputFile='output.csv',
portionSize=2,
textColumn='example',
shortLabels=None,
):
if shortLabels == None:
shortLabels = labels
out = Path(outputFile)
if out.exists():
outdf = pd.read_csv(out)
currentId = outdf.index.max() + 1
else:
currentId = 0
indf = pd.read_csv(inputFile)
examplesInFile = len(indf)
indf = indf.loc[currentId:currentId + portionSize - 1]
actualPortionSize = len(indf)
print(f'{currentId + 1} - {currentId + actualPortionSize} of {examplesInFile}')
sentences = indf[textColumn].tolist()
for label in shortLabels:
indf[label] = None
def updateRow(example, selectedLabels):
print(example, selectedLabels)
labs = setLabels([labels.index(y) for y in selectedLabels], len(labels))
indf.loc[indf[textColumn] == example, shortLabels] = labs
def finalProcessing(annotations):
if out.exists():
prevdata = pd.read_csv(out)
outdata = pd.concat([prevdata, indf]).reset_index(drop=True)
else:
outdata = indf.copy()
outdata.to_csv(out, index=False)
annotated = annotate(
sentences,
options=labels,
task_type='multilabel-classification',
buttons_in_a_row=3,
reset_buttons_after_click=True,
include_next=False,
example_process_fn=updateRow,
final_process_fn=finalProcessing
)
return indf
def getAnnotationsCountPerlabel(annotations, shortLabels):
countPerLabel = pd.DataFrame(columns=shortLabels, index=['count'])
for label in shortLabels:
countPerLabel.loc['count', label] = len(annotations.loc[annotations[label] == 1.0])
return countPerLabel
def getAnnotationsCountPerlabel(annotations, shortLabels):
countPerLabel = pd.DataFrame(columns=shortLabels, index=['count'])
for label in shortLabels:
countPerLabel.loc['count', label] = len(annotations.loc[annotations[label] == 1.0])
return countPerLabel
annotations = labelPortion('inputtestdata.csv',
labels=labels,
shortLabels= shortLabels)
# counts per label
getAnnotationsCountPerlabel(annotations, shortLabels)
Preview:
The complete and runnable examples are available in the provided Notebook.
FAQs
Quickly annotate data in Jupyter notebooks.
We found that pigeonxt-jupyter demonstrated a healthy version release cadence and project activity because the last version was released less than a year ago. It has 1 open source maintainer collaborating on the project.
Did you know?
Socket for GitHub automatically highlights issues in each pull request and monitors the health of all your open source dependencies. Discover the contents of your packages and block harmful activity before you install or update your dependencies.
Security News
Research
The Socket Research Team breaks down a malicious wrapper package that uses obfuscation to harvest credentials and exfiltrate sensitive data.
Research
Security News
Attackers used a malicious npm package typosquatting a popular ESLint plugin to steal sensitive data, execute commands, and exploit developer systems.
Security News
The Ultralytics' PyPI Package was compromised four times in one weekend through GitHub Actions cache poisoning and failure to rotate previously compromised API tokens.