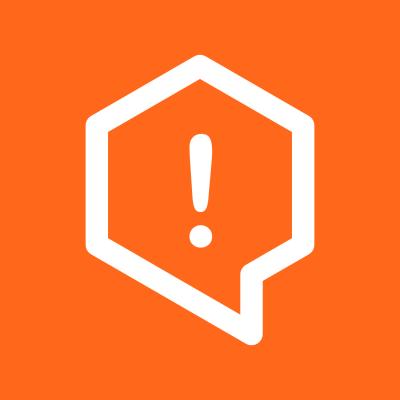
Security News
Fluent Assertions Faces Backlash After Abandoning Open Source Licensing
Fluent Assertions is facing backlash after dropping the Apache license for a commercial model, leaving users blindsided and questioning contributor rights.
A Python client library for communicating with the Private AI API. This document provides information about how to best use the client. For more information, see Private AI's API Documentation.
pip install privateai_client
from privateai_client import PAIClient
from privateai_client import request_objects
client = PAIClient(url="http://localhost:8080")
text_request = request_objects.process_text_obj(text=["My sample name is John Smith"])
response = client.process_text(text_request)
print(text_request.text)
print(response.processed_text)
Output:
['My sample name is John Smith']
['My sample name is [NAME_1]']
We use pytest to run our tests in the tests folder.
To run from command line, ensure you have pytest installed, and then run pytest
from the main project folder.
pip install -r requirements.dev.txt
pytest
Alternatively, you can run automatically run all tests from the Testing window in Visual Studio Code.
The PAI client requires a scheme, host, and optional port to initialize.
Alternatively, a full url can be used.
Once created, the connection can be tested with the client's ping
function
scheme = 'http'
host = 'localhost'
port= '8080'
client = PAIClient(scheme, host, port)
client.ping()
url = "http://localhost:8080"
client = PAIClient(url=url)
client.ping()
Output:
True
True
from privateai_client import PAIClient
# On initialization
client = PAIClient(url="http://localhost:8080", api_key='testkey')
# After initialization
client = PAIClient(url="http://localhost:8080")
client.ping()
client.add_api_key("testkey")
client.ping()
Output:
The request returned with a 401 Unauthorized
True
Once initialized the client can be used to make any request listed in the Private-AI documentation
Available requests:
Client Function | Endpoint |
---|---|
get_version() | / |
ping() | /healthz |
get_metrics() | /metrics |
get_diagnostics() | /diagnostics |
process_text() | /process/text |
process_files_uri() | /process/files/uri |
process_files_base64() | /process/files/base64 |
bleep() | /bleep |
Requests can be made using dictionaries:
sample_text = ["This is John Smith's sample dictionary request"]
text_dict_request = {"text": sample_text}
response = client.process_text(text_dict_request)
print(response.processed_text)
Output:
["This is [NAME_1]'s sample dictionary request"]
or using built-in request objects:
from privateai_client import request_objects
sample_text = "This is John Smith's sample process text object request"
text_request_object = request_objects.process_text_obj(text=[sample_text])
response = client.process_text(text_request_object)
print(response.processed_text)
Output:
["This is [NAME_1]'s sample process text object request"]
Request objects are a simple way of creating request bodies without the tediousness of writing dictionaries. Every post request (as listed in the Private-AI documentation) has its own request own request object.
from privateai_client import request_objects
sample_obj = request_objects.file_uri_obj(uri='path/to/file.jpg')
sample_obj.uri
Output:
'path/to/file.jpg'
Additionally there are request objects for each nested dictionary of a request:
from privateai_client import request_objects
sample_text = "This is John Smith from Sample Company to show a sample process text object request where organizations won't be removed, but John will be recognized as the same entity"
# sub-dictionary of entity_detection
sample_entity_type_selector = request_objects.entity_type_selector_obj(type="DISABLE", value=["ORGANIZATION"])
# sub-dictionary of a process text request
sample_entity_detection = request_objects.entity_detection_obj(entity_types=[sample_entity_type_selector])
# sub-dictionary of a process text request
sample_processed_text = request_objects.processed_text_obj(type="MARKER", pattern="[UNIQUE_NUMBERED_ENTITY_TYPE]", coreference_resolution="model")
# request object created using the sub-dictionaries
sample_request = request_objects.process_text_obj(text=[sample_text], entity_detection=sample_entity_detection, processed_text=sample_processed_text)
response = client.process_text(sample_request)
print(response.processed_text)
Output:
["This is [NAME_1] from Sample Company to show a sample process text object request where organizations won't be removed, but [NAME_1] will be recognized as the same entity"]
Request objects can initialized by passing in all the required values needed for the request as arguments or from a dictionary, using the object's fromdict
function. Any object can be created as per the Private AI documentation.
# Passing arguments
sample_data = "JVBERi0xLjQKJdPr6eEKMSAwIG9iago8PC9UaXRsZSAoc2FtcGxlKQovUHJvZHVj..."
sample_content_type = "application/pdf"
sample_file_obj = request_objects.file_obj(data=sample_data, content_type=sample_content_type)
# Passing a dictionary using .fromdict()
sample_dict = {"data": "JVBERi0xLjQKJdPr6eEKMSAwIG9iago8PC9UaXRsZSAoc2FtcGxlKQovUHJvZHVj...",
"content_type": "application/pdf"}
sample_file_obj2 = request_objects.file_obj.fromdict(sample_dict)
Request objects also can be formatted as dictionaries, using the request object's to_dict()
function:
from privateai_client import request_objects
sample_text = "Sample text."
sample_accuracy = "standard"
# Create the nested request objects
sample_entity_type_selector = request_objects.entity_type_selector_obj(type="DISABLE", value=['HIPAA_SAFE_HARBOR'])
sample_entity_detection = request_objects.entity_detection_obj(
entity_types=[sample_entity_type_selector],
accuracy=sample_accuracy
)
# Create the request object
sample_request = request_objects.process_text_obj(text=[sample_text], entity_detection=sample_entity_detection)
# All nested request objects are also formatted
print(sample_request.to_dict())
Output:
{
'text': ['Sample text.'],
'link_batch': False,
'entity_detection': {'accuracy': 'standard', 'entity_types': [{'type': 'DISABLE', 'value': ['HIPAA_SAFE_HARBOR']}], 'filter': [], 'return_entity': True},
'processed_text': {'type': 'MARKER', 'pattern': '[UNIQUE_NUMBERED_ENTITY_TYPE]'}
}
from privateai_client import PAIClient
from privateai_client.objects import request_objects
import os
import logging
file_dir = "/path/to/file/directory"
client = PAIClient(url="http://localhost:8080")
for file_name in os.listdir(file_dir):
filepath = os.path.join(file_dir, file_name)
if not os.path.isfile(filepath):
continue
req_obj = request_objects.file_uri_obj(uri=filepath)
# NOTE this method of file processing requires the container to have an the input and output directories mounted
resp = client.process_files_uri(req_obj)
from privateai_client import PAIClient
from privateai_client.objects import request_objects
import base64
import os
import logging
file_dir = "/path/to/your/file"
file_name = 'sample_file.pdf'
filepath = os.path.join(file_dir,file_name)
file_type= "type/of_file" #eg. application/pdf
client = PAIClient(url="http://localhost:8080")
# Read from file
with open(filepath, "rb") as b64_file:
file_data = base64.b64encode(b64_file.read())
file_data = file_data.decode("ascii")
# Make the request
file_obj = request_objects.file_obj(data=file_data, content_type=file_type)
request_obj = request_objects.file_base64_obj(file=file_obj)
resp = client.process_files_base64(request_object=request_obj)
# Write to file
with open(os.path.join(file_dir,f"redacted-{file_name}"), 'wb') as redacted_file:
processed_file = resp.processed_file.encode("ascii")
processed_file = base64.b64decode(processed_file, validate=True)
redacted_file.write(processed_file)
from privateai_client import PAIClient
from privateai_client.objects import request_objects
import base64
import os
import logging
file_dir = "/path/to/your/file"
file_name = 'sample_file.pdf'
filepath = os.path.join(file_dir,file_name)
file_type= "type/of_file" #eg. audio/mp3 or audio/wav
client = PAIClient(url="http://localhost:8080")
file_dir = "/home/adam/workstation/file_processing/test_audio"
file_name = "test_audio.mp3"
filepath = os.path.join(file_dir,file_name)
file_type = "audio/mp3"
with open(filepath, "rb") as b64_file:
file_data = base64.b64encode(b64_file.read())
file_data = file_data.decode("ascii")
file_obj = request_objects.file_obj(data=file_data, content_type=file_type)
timestamp = request_objects.timestamp_obj(start=1.12, end=2.14)
request_obj = request_objects.bleep_obj(file=file_obj, timestamps=[timestamp])
resp = client.bleep(request_object=request_obj)
with open(os.path.join(file_dir,f"redacted-{file_name}"), 'wb') as redacted_file:
processed_file = resp.bleeped_file.encode("ascii")
processed_file = base64.b64decode(processed_file, validate=True)
redacted_file.write(processed_file)
Redacting a data frame column by column
# Working with data frames
import pandas as pd
from privateai_client import PAIClient
from privateai_client.objects import request_objects
client = PAIClient(url="http://localhost:8080")
data_frame = pd.DataFrame(
{
"Name": [
"Braund, Mr. Owen Harris",
"Allen, Mr. William Henry",
"Bonnell, Miss. Elizabeth",
],
"Age": [22, 35, 58],
"Sex": ["male", "male", "female"],
}
)
print(data_frame)
text_req = request_objects.process_text_obj(text=[])
for column in data_frame.columns:
text_req.text.append(f"{column}:{' | '.join([str(row) for row in data_frame[column]])}")
resp = client.process_text(text_req)
redacted_data = dict()
for row in resp.processed_text:
data = row.split(':',1)
redacted_data[data[0]] = data[1].split(' | ')
redacted_data_frame = pd.DataFrame(redacted_data)
print(redacted_data_frame)
Redacting cell by cell for columns with large text content
# Working with data frames
import pandas as pd
from privateai_client import PAIClient
from privateai_client.objects import request_objects
client = PAIClient(url="http://localhost:8080")
data_frame = pd.DataFrame(
{
"Book": [
"Treasure Island",
"Moby Dick",
],
"chapter": [1,1],
"paragraph": [1,1],
"text": ["The Old Sea-dog at the Admiral Benbow\nSquire Trelawney, Dr. Livesey, and the rest of...",
"Call me Ishmael. Some years ago—never mind how long precisely—having little or no money in my purse..."
]
}
)
obj = request_objects.process_text_obj
func = client.process_text
data_frame['text'] = [(lambda x: func(obj(text=[x])).processed_text[0])(row) for row in data_frame['text']]
Reidentifying Text
from privateai_client import PAIClient
from privateai_client import request_objects
client = PAIClient(url="http://localhost:8080")
# Deidentify the text
initial_text = 'My name is John. I work for Private AI'
request_obj = request_objects.process_text_obj(text=[initial_text])
response_obj = client.process_text(request_obj)
# Build reidentify request object from the deidentified response
new_request_obj = response_obj.get_reidentify_request()
# Call the reidentify Route
new_response_obj = client.reidentify_text(new_request_obj)
print(new_response_obj.body)
FAQs
A thin client for communicating with the Private AI de-identication API.
We found that privateai-client demonstrated a healthy version release cadence and project activity because the last version was released less than a year ago. It has 2 open source maintainers collaborating on the project.
Did you know?
Socket for GitHub automatically highlights issues in each pull request and monitors the health of all your open source dependencies. Discover the contents of your packages and block harmful activity before you install or update your dependencies.
Security News
Fluent Assertions is facing backlash after dropping the Apache license for a commercial model, leaving users blindsided and questioning contributor rights.
Research
Security News
Socket researchers uncover the risks of a malicious Python package targeting Discord developers.
Security News
The UK is proposing a bold ban on ransomware payments by public entities to disrupt cybercrime, protect critical services, and lead global cybersecurity efforts.