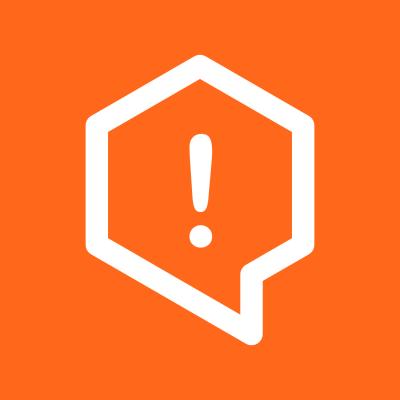
Security News
Fluent Assertions Faces Backlash After Abandoning Open Source Licensing
Fluent Assertions is facing backlash after dropping the Apache license for a commercial model, leaving users blindsided and questioning contributor rights.
The Python Performance Analysis Library (py-pal) is a profiling tool for the Python programming language. With py-pal one can approximate the time complexity (big O notation) of Python functions in an empirical way. The arguments of the function and the executed opcodes serve as a basis for the analysis.
To the docs <https://py-pal.readthedocs.io>
_.
This project requires CPython and a C compiler to run. Install CPython >= 3.7, then install py-pal by running:
pip install py-pal
or
python -m pip install py-pal
python -m py_pal <target-module/file>
or
py-pal <target-module/file>
There are multiple aliases to the same command: py-pal
, py_pal
and pypal
. If py-pal is executed this way, all
functions called in the code are captured and analyzed. The output is in the form of a pandas data frame.
See the help message:
py-pal -h
py-pal can perform cost analysis on a line-by-line basis:
py-pal <file> -l/--line
The --separate flag can be used to examine the cost function of individual arguments (caution: this function assumes the independence of the arguments):
py-pal <file> -s/--separate
The output of the results can be noisy, to limit this you can use --filter-function to filter the desired functions from the result. Regular expressions are supported:
py-pal <file> -ff/--filter-function .*_sort
Similarly, the result can also be filtered by modules with --filter-module, e.g. to exclude importlib modules
py-pal <file> -fm/--filter-module "^(?!<frozen.*>).*"
To save the results in a specified folder use --out:
py-pal <file> -o/--out results
The output format can be changed with --format:
py-pal <file> -o/--out results --format json
With the additional specification of the --plot flag, the cost functions of the result set are stored as images:
py-pal <file> -o/--out results -p/--plot
For the --log-level flag see the development <https://py-pal.readthedocs.io/en/latest/development.html#logging>
_ docs.
Example, creating plots for selected functions:
py-pal tests/examples/sort.py -o results -p -f sort
To profile a single function and get the complexity estimate there is profile_function.
.. sourcecode:: python
from py_pal.core import profile_function
from py_pal.data_collection.opcode_metric import OpcodeMetric
from py_pal.datagen import gen_random_growing_lists
from algorithms.sort import bubble_sort
profile_function(OpcodeMetric(), gen_random_growing_lists(), bubble_sort)
The profile decorator:
.. sourcecode:: python
from py_pal.core import profile, DecoratorStore
@profile
def test():
pass
# Must be called at some point
test()
estimator = AllArgumentEstimator(DecoratorStore.get_call_stats(), DecoratorStore.get_opcode_stats())
res = estimator.export()
By using the profile decorator, it is possible to annotate Python functions such that only the annotated Python functions will be profiled. It acts similar to a whitelist filter.
Another possibility is to use the context-manager protocol:
.. sourcecode:: python
from py_pal.analysis.estimator import AllArgumentEstimator
from py_pal.data_collection.tracer import Tracer
with Tracer() as t:
pass
estimator = AllArgumentEstimator(t.get_call_stats(), t.get_opcode_stats())
res = estimator.export()
# Do something with the resulting DataFrame
print(res)
The most verbose way to use the py-pal API:
.. sourcecode:: python
from py_pal.analysis.estimator import AllArgumentEstimator
from py_pal.data_collection.tracer import Tracer
t = Tracer()
t.trace()
# Your function
pass
t.stop()
estimator = AllArgumentEstimator(t.get_call_stats(), t.get_opcode_stats())
res = estimator.export()
# Do something with the resulting DataFrame
print(res)
All examples instantiate a tracer object that is responsible for collecting the data. After execution, the collected data is passed to the analysis module. Finally, an estimate of the asymptotic runtime of the functions contained in the code is obtained.
In the current version py-pal offers only the profiling mode. Although py_pal.datagen
offers some functions for
generating inputs, py-pal must be combined with appropriate test cases to realize a performance testing mode. An
automatic detection and generation of appropriate test inputs does not exist at the moment.
The profiling approach implemented by the py-pal modules does not distinguish between different threads executing a Python function. Actually it is a major problem to profile a Python script which makes use of threads. The bytecode counting strategy will increase all counters of Python functions on the current call stack no matter what threads is executing it. Thus, the data points will not be accurate to what really happened during the profiled execution of the script.
This work integrates some code from the big_O <https://github.com/pberkes/big_O>
_ project. More specifically, most
code in py_pal.analysis.complexity
, py_pal.datagen
and py_pal.analysis.estimator.Estimator.infer_complexity
is adapted from bigO.
Command line interface changes: """""""""""""""""""""""""""""""
Command line interface changes: """""""""""""""""""""""""""""""
--save
flag in favor of -o/--out
-V/--visualize
to -p/--plot
-f/--function
from executing and profiling a specific function inside a python file to applying the analysis to a selected function. Regular expressions are suported.py_pal.datagen
Refactoring """"""""""" Project structure changes, overall CLI interface is unchanged. API changes:
py_pal.tracer
moved to py_pal.data_collection.tracer
py_pal.complexity
and py_pal.estimator
moved to the py_pal.analysis
package.py_pal.analysis.estimator.Estimator
now takes call and opcode stats as arguments.Refactoring """""""""""
The estimator
module was refactored which introduces a slight change to the API.
Classes inheriting from Estimator
now only specify how to transform the collected data with respect to the arguments
of the function.
Instead of ComplexityEstimator
you should use the AllArgumentEstimator
class. Additionally there is the
SeparateArgumentEstimator
which is experimental.
More accurate Data Collection """""""""""""""""""""""""""""
The Tracer
is enhanced by measuring builtin function calls with AdvancedOpcodeMetric
.
Opcodes resembling a function call .e.g FUNCTION_CALL
are filtered for built in function calls.
If the called function is found in the complexity mapping a synthetic Opcode weight gets assigned.
A builtin function call is evaluated using its argument and a pre-defined runtime complexity e.g. O(n log n) for
sort()
.
OpcodeMetric
instance to the Tracer
AdvancedOpcodeMetric
instance assigned to the Tracer
provides statistics about how many builtin function calls were observed and how many were found in the complexity mapBugfixes """"""""
FAQs
Estimate Asymptotic Runtime Complexity from Bytecode executions
We found that py-pal demonstrated a healthy version release cadence and project activity because the last version was released less than a year ago. It has 1 open source maintainer collaborating on the project.
Did you know?
Socket for GitHub automatically highlights issues in each pull request and monitors the health of all your open source dependencies. Discover the contents of your packages and block harmful activity before you install or update your dependencies.
Security News
Fluent Assertions is facing backlash after dropping the Apache license for a commercial model, leaving users blindsided and questioning contributor rights.
Research
Security News
Socket researchers uncover the risks of a malicious Python package targeting Discord developers.
Security News
The UK is proposing a bold ban on ransomware payments by public entities to disrupt cybercrime, protect critical services, and lead global cybersecurity efforts.