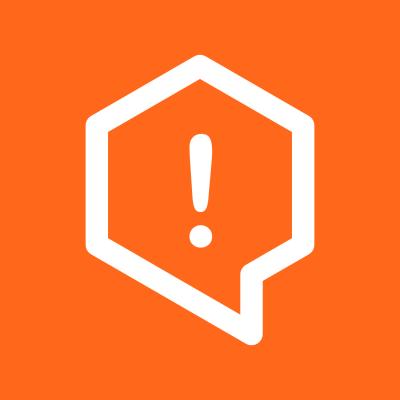
Security News
Fluent Assertions Faces Backlash After Abandoning Open Source Licensing
Fluent Assertions is facing backlash after dropping the Apache license for a commercial model, leaving users blindsided and questioning contributor rights.
PyPMML is a Python PMML scoring library, it really is the Python API for PMML4S.
pip install pypmml
Or install the latest version from github:
pip install --upgrade git+https://github.com/autodeployai/pypmml.git
Load model from various sources, e.g. readable, file path, string, or an array of bytes.
from pypmml import Model
# The model is from http://dmg.org/pmml/pmml_examples/KNIME_PMML_4.1_Examples/single_iris_dectree.xml
model = Model.load('single_iris_dectree.xml')
Call predict(data)
to predict new values that can be in different types, e.g. dict, list, json, ndarray of NumPy, Series or DataFrame of Pandas.
data
in dict:>>> model.predict({'sepal_length': 5.1, 'sepal_width': 3.5, 'petal_length': 1.4, 'petal_width': 0.2})
{'probability_Iris-setosa': 1.0, 'probability_Iris-versicolor': 0.0, 'probability': 1.0, 'predicted_class': 'Iris-setosa', 'probability_Iris-virginica': 0.0, 'node_id': '1'}
data
in list:NOTE: the order of values must match the input names, and the order of results always matches the output names.
>>> model.inputNames
['sepal_length', 'sepal_width', 'petal_length', 'petal_width']
>>> model.predict([5.1, 3.5, 1.4, 0.2])
['Iris-setosa', 1.0, 1.0, 0.0, 0.0, '1']
>>> model.outputNames
['predicted_class', 'probability', 'probability_Iris-setosa', 'probability_Iris-versicolor', 'probability_Iris-virginica', 'node_id']
data
in records
json:>>> model.predict('[{"sepal_length": 5.1, "sepal_width": 3.5, "petal_length": 1.4, "petal_width": 0.2}]')
[{"probability":1.0,"probability_Iris-versicolor":0.0,"probability_Iris-setosa":1.0,"probability_Iris-virginica":0.0,"predicted_class":"Iris-setosa","node_id":"1"}]
data
in split
json:>>> model.predict('{"columns": ["sepal_length", "sepal_width", "petal_length", "petal_width"], "data": [[5.1, 3.5, 1.4, 0.2]]}')
{"columns":["predicted_class","probability","probability_Iris-setosa","probability_Iris-versicolor","probability_Iris-virginica","node_id"],"data":[["Iris-setosa",1.0,1.0,0.0,0.0,"1"]]}
data
in ndarray of NumPy:NOTE: as the list above, the order of ndarray values must match the input names, and the order of results always matches the output names.
>>> import numpy as np
>>> model.predict(np.array([5.1, 3.5, 1.4, 0.2]))
['Iris-setosa', 1.0, 1.0, 0.0, 0.0, '1']
>>>
>>> model.predict(np.array([[5.1, 3.5, 1.4, 0.2], [7, 3.2, 4.7, 1.4]]))
[['Iris-setosa', 1.0, 1.0, 0.0, 0.0, '1'], ['Iris-versicolor', 0.9074074074074074, 0.0, 0.9074074074074074, 0.09259259259259259, '3']]
data
in Series of Pandas:>>> import pandas as pd
>>> model.predict(pd.Series({'sepal_length': 5.1, 'sepal_width': 3.5, 'petal_length': 1.4, 'petal_width': 0.2}))
node_id 1
predicted_class Iris-setosa
probability 1
probability_Iris-setosa 1
probability_Iris-versicolor 0
probability_Iris-virginica 0
Name: 0, dtype: object
data
in DataFrame of Pandas:>>> import pandas as pd
>>> data = pd.read_csv('Iris.csv') # The data is from here: http://dmg.org/pmml/pmml_examples/Iris.csv
>>> model.predict(data)
node_id predicted_class probability probability_Iris-setosa probability_Iris-versicolor probability_Iris-virginica
0 1 Iris-setosa 1.000000 1.0 0.000000 0.000000
1 1 Iris-setosa 1.000000 1.0 0.000000 0.000000
2 1 Iris-setosa 1.000000 1.0 0.000000 0.000000
3 1 Iris-setosa 1.000000 1.0 0.000000 0.000000
4 1 Iris-setosa 1.000000 1.0 0.000000 0.000000
.. ... ... ... ... ... ...
145 10 Iris-virginica 0.978261 0.0 0.021739 0.978261
146 10 Iris-virginica 0.978261 0.0 0.021739 0.978261
147 10 Iris-virginica 0.978261 0.0 0.021739 0.978261
148 10 Iris-virginica 0.978261 0.0 0.021739 0.978261
149 10 Iris-virginica 0.978261 0.0 0.021739 0.978261
PyPMML supports both backends access to Java from Python: "py4j" and "jpype", Py4j
is used by default, you can call the following code to switch to jpype
before loading models:
from pypmml import PMMLContext
PMMLContext.getOrCreate(gateway="jpype")
See the PMML4S project. PMML4S is a PMML scoring library for Scala. It provides both Scala and Java Evaluator API for PMML.
See the PMML4S-Spark project. PMML4S-Spark is a PMML scoring library for Spark as SparkML Transformer.
See the PyPMML-Spark project. PyPMML-Spark is a Python PMML scoring library for PySpark as SparkML Transformer, it really is the Python API for PMML4s-Spark.
See the AI-Serving project. AI-Serving is serving AI/ML models in the open standard formats PMML and ONNX with both HTTP (REST API) and gRPC endpoints.
If you have any questions about the PyPMML library, please open issues on this repository.
Feedback and contributions to the project, no matter what kind, are always very welcome.
PyPMML is licensed under APL 2.0.
FAQs
Python PMML scoring library
We found that pypmml demonstrated a healthy version release cadence and project activity because the last version was released less than a year ago. It has 1 open source maintainer collaborating on the project.
Did you know?
Socket for GitHub automatically highlights issues in each pull request and monitors the health of all your open source dependencies. Discover the contents of your packages and block harmful activity before you install or update your dependencies.
Security News
Fluent Assertions is facing backlash after dropping the Apache license for a commercial model, leaving users blindsided and questioning contributor rights.
Research
Security News
Socket researchers uncover the risks of a malicious Python package targeting Discord developers.
Security News
The UK is proposing a bold ban on ransomware payments by public entities to disrupt cybercrime, protect critical services, and lead global cybersecurity efforts.