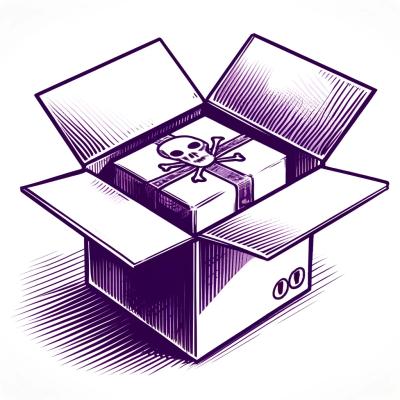
Security News
Research
Data Theft Repackaged: A Case Study in Malicious Wrapper Packages on npm
The Socket Research Team breaks down a malicious wrapper package that uses obfuscation to harvest credentials and exfiltrate sensitive data.
RAGBooster improves the performance of retrieval-based large language models by learning which data sources are important to retrieve high quality data.
We provide an example notebook that shows how we boost RedPajama-INCITE-Instruct-3B-v1, a small LLM with 3 billion parameters to be on par with OpenAI's GPT3.5 (175 billion parameters) in a question answering task by using Bing websearch and ragbooster:
Furthermore, we have an additional example notebook, where we demonstrate how to boost a tiny qa model to get within 5% accuracy on GPT3.5 on a data imputation task:
At the core of RAGBooster are RetrievalAugmentedModels, which fetch external data to improve prediction quality. Retrieval augmentation requires two components:
Once you defined your retrieval-augmented model, you can leverage RAGBooster to boost its performance by learning the data importance of retrieval sources (e.g., domains in the web). This often increases accuracy by a few percent.
Have a look at our paper on Improving Retrieval-Augmented Large Language Models with Data-Centric Refinement for detailed algorithms, proofs and experimental results.
RAGBooster is available as pip package, and can be installed as follows:
pip install ragbooster
git clone git@github.com:amsterdata/ragbooster.git
cd ragbooster
python3.9 -m venv venv
source venv/bin/activate
pip install ".[dev]"
maturin develop --release
cargo test --release
RUSTFLAGS="-C target-cpu=native" cargo bench
flake8 python
jupyter notebook
and run the example notebooksFAQs
Unknown package
We found that ragbooster demonstrated a healthy version release cadence and project activity because the last version was released less than a year ago. It has 1 open source maintainer collaborating on the project.
Did you know?
Socket for GitHub automatically highlights issues in each pull request and monitors the health of all your open source dependencies. Discover the contents of your packages and block harmful activity before you install or update your dependencies.
Security News
Research
The Socket Research Team breaks down a malicious wrapper package that uses obfuscation to harvest credentials and exfiltrate sensitive data.
Research
Security News
Attackers used a malicious npm package typosquatting a popular ESLint plugin to steal sensitive data, execute commands, and exploit developer systems.
Security News
The Ultralytics' PyPI Package was compromised four times in one weekend through GitHub Actions cache poisoning and failure to rotate previously compromised API tokens.