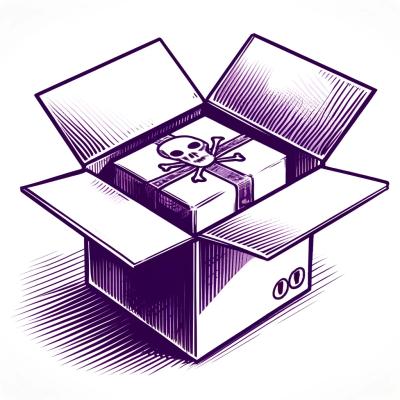
Security News
Research
Data Theft Repackaged: A Case Study in Malicious Wrapper Packages on npm
The Socket Research Team breaks down a malicious wrapper package that uses obfuscation to harvest credentials and exfiltrate sensitive data.
Parallel processing wrapper for rasterio
|PyPI| |Build Status| |Coverage Status|
From pypi:
pip install rio-mucho
From github (usually for a branch / dev):
pip install pip install git+ssh://git@github.com/mapbox/rio-mucho.git@<branch>#egg=riomucho
Development:
::
git clone git@github.com:mapbox/rio-mucho.git
cd rio-mucho
pip install -e .
.. code:: python
with riomucho.RioMucho([{inputs}], {output}, {run function},
windows={windows},
global_args={global arguments},
options={options to write}) as rios:
rios.run({processes})
Arguments
``inputs``
^^^^^^^^^^
An list of file paths to open and read.
``output``
^^^^^^^^^^
What file to write to.
``run_function``
^^^^^^^^^^^^^^^^
A function to be applied to each window chunk. This should have input
arguments of:
1. A data input, which can be one of:
- A list of numpy arrays of shape (x,y,z), one for each file as
specified in input file list ``mode="simple_read" [default]``
- A numpy array of shape ({*n* input files x *n* band count}, {window
rows}, {window cols}) ``mode=array_read"``
- A list of open sources for reading ``mode="manual_read"``
2. A ``rasterio`` window tuple
3. A ``rasterio`` window index (``ij``)
4. A global arguments object that you can use to pass in global
arguments
This should return:
1. An output array of ({count}, {window rows}, {window cols}) shape, and
of the correct data type for writing
.. code:: python
def basic_run({data}, {window}, {ij}, {global args}):
## do something
return {out}
Keyword arguments
windows={windows}
^^^^^^^^^^^^^^^^^^^^^
A list of rasterio
(window, ij) tuples to operate on.
[Default = src[0].block_windows()]
global_args={global arguments}
^^^^^^^^^^^^^^^^^^^^^^^^^^^^^^^^^^
Since this is working in parallel, any other objects / values that you
want to be accessible in the run_function
. [Default = {}]
.. code:: python
global_args = {
'divide_value': 2
}
options={keyword args}
^^^^^^^^^^^^^^^^^^^^^^^^^^
The options to pass to the writing output. [Default = srcs[0].meta
.. code:: python
import riomucho, rasterio, numpy
def basic_run(data, window, ij, g_args):
## do something
out = np.array(
[d[0] /= global_args['divide'] for d in data]
)
return out
# get windows from an input
with rasterio.open('/tmp/test_1.tif') as src:
## grabbing the windows as an example. Default behavior is identical.
windows = [[window, ij] for ij, window in src.block_windows()]
options = src.meta
# since we are only writing to 2 bands
options.update(count=2)
global_args = {
'divide': 2
}
processes = 4
# run it
with riomucho.RioMucho(['input1.tif','input2.tif'], 'output.tif', basic_run,
windows=windows,
global_args=global_args,
options=options) as rm:
rm.run(processes)
`riomucho.utils.array_stack([array, array, array,...])
Given a list of ({depth}, {rows}, {cols}) numpy arrays, stack into a
single (l{list length \* each image depth}, {rows}, {cols}) array. This
is useful for handling variation between ``rgb`` inputs of a single
file, or separate files for each.
One RGB file
^^^^^^^^^^^^
.. code:: python
files = ['rgb.tif']
open_files = [rasterio.open(f) for f in files]
rgb = `riomucho.utils.array_stack([src.read() for src in open_files])
Separate RGB files
^^^^^^^^^^^^^^^^^^
.. code:: python
files = ['r.tif', 'g.tif', 'b.tif']
open_files = [rasterio.open(f) for f in files]
rgb = `riomucho.utils.array_stack([src.read() for src in open_files])
.. |PyPI| image:: https://img.shields.io/pypi/v/rio-mucho.svg?maxAge=2592000?style=plastic
:target:
.. |Build Status| image:: https://travis-ci.org/mapbox/rio-mucho.svg?branch=master
:target: https://travis-ci.org/mapbox/rio-mucho
.. |Coverage Status| image:: https://coveralls.io/repos/mapbox/rio-mucho/badge.svg?branch=master&service=github
:target: https://coveralls.io/github/mapbox/rio-mucho?branch=master
FAQs
Windowed multiprocessing wrapper for rasterio
We found that rio-mucho demonstrated a healthy version release cadence and project activity because the last version was released less than a year ago. It has 1 open source maintainer collaborating on the project.
Did you know?
Socket for GitHub automatically highlights issues in each pull request and monitors the health of all your open source dependencies. Discover the contents of your packages and block harmful activity before you install or update your dependencies.
Security News
Research
The Socket Research Team breaks down a malicious wrapper package that uses obfuscation to harvest credentials and exfiltrate sensitive data.
Research
Security News
Attackers used a malicious npm package typosquatting a popular ESLint plugin to steal sensitive data, execute commands, and exploit developer systems.
Security News
The Ultralytics' PyPI Package was compromised four times in one weekend through GitHub Actions cache poisoning and failure to rotate previously compromised API tokens.