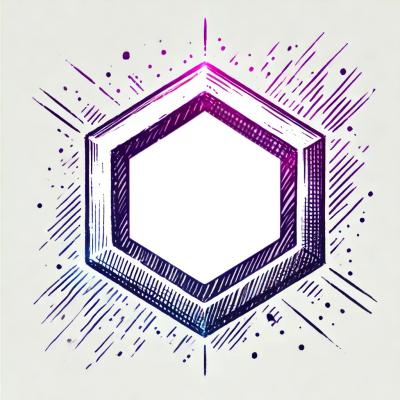
Security News
Maven Central Adds Sigstore Signature Validation
Maven Central now validates Sigstore signatures, making it easier for developers to verify the provenance of Java packages.
A Python module for mixed-methods qualitative studies of large textual or symbolic corpora, providing applications of statistical models accompanied by tailored interactive visualizations. It affords the detection of both textual and metadata structures by employing stochastic block modeling (SBM) from `graph-tool` or (currently deprecated) word embedding from `gensim`.
A Python module for mixed-methods qualitative studies of large textual or
symbolic corpora, providing applications of statistical models accompanied by
tailored interactive visualizations. It affords the detection of both textual
and metadata structures by employing stochastic block modeling (SBM) from
graph-tool
or (currently deprecated) word embedding from gensim
.
Much of the functionality of sashimi
is available as a suite of methods in the Cortext
Manager web service. See their documentation for
SASHIMI, which may also serve users of the Python module as an introduction to the methodology.
You must first sharpen your knife install graph-tool
by following the installation instructions according to your system.
Then:
pip install sashimi-domains
With the exception of graph-tool, dependencies will be automatically installed by pip
.
This project builds mainly on the following others:
from sashimi import GraphModels
import pandas as pd
# Let's instantiate a corpus with an explicit storage dir
corpus = GraphModels(storage_dir="my_project")
# Read a dataframe from a CSV file and load it into the corpus, giving it a name
df = pd.read_csv("example_corpus.csv")
corpus.load_data(df, name='example')
# Take a look at what was loaded
print(corpus.data)
# For autoloading, store the data in JSON format under the project's storage dir
corpus.store_data()
For the typical usage with a textual corpus:
# Set the data column labels for text sources
corpus.text_sources = ["title", "body"]
# Set up how to process text sources, for example:
text_sources_args = dict(ngrams=3, language="en", stop_words=True)
The language
is any valid language for spacy
, where None
will use the English tokenizer with no stop_words. Our English tokenizer is slightly improved from spacy
's original, in order to get ["hot-dog"] out of "hot-dog" (when spacy would split that), ["this", "that"] out of "this/that", and ["citation"] out of "citation[2,3]".
You may also directly use tokens that you have processed yourself, or from token data such as keywords, categories, or anything really, in addition or in place of textual data.
# Set the data column labels for token sources
corpus.token_sources = ["keyword", "category"]
Token data for a document is expected to be in the form of a list, containing strings or lists containing strings: List[str | List[str]]
. If your data differs, you must adjust it before processing.
Once token and text sources are set up, you can process them all with:
corpus.process_sources(**text_sources_args)
# Set data column labels
corpus.col_title = "titles" # document titles (required; if your corpus doesn't have canonical titles, be creative)
corpus.col_time = "years" # dates (optional)
corpus.col_urls = "urls" # may also be a list of columns, for multiple urls (optional)
# Load a domain-topic model
corpus.load_domain_topic_model()
print(corpus.state)
print(corpus.dblocks)
print(corpus.tblocks)
# Create an interactive hierarchical map
# Output is a self-contained html+css+js+data document
corpus.domain_map()
# Create network representations for the first domain and topic levels, as pdf, svg and graphml documents
corpus.domain_network(doc_level=1, ter_level=1)
corpus.domain_network(doc_level=2, ter_level=1)
# The default representation shows edges from a domain to its "specific" topics, with the previous call being equivalent to the following
corpus.domain_network(doc_level=2, ter_level=1, edges="specific")
# For domains of level higher than 1, setting `edges="common"` will show edges towards topics that are common to all of its subdomains
corpus.domain_network(doc_level=2, ter_level=1, edges="common")
# Create domain-topic tables for all domains at level 3
if 3 in corpus.dblocks:
corpus.subxblocks_tables(xbtype="doc", xlevel=3, xb=None, ybtype="ter")
# Store the current choices of corpus and model
corpus.register_config("my_config.json")
# To reload them in a future session
from sashimi import GraphModels
corpus = GraphModels('my_config.json')
corpus.load_domain_topic_model() # will load the previously calculated model
corpus.load_domain_topic_model(load=False) # will fit a new model
print(corpus.list_blockstates())
# Load a domain-chained model over the column "metadata_A"
corpus.set_chain(prop='metadata_A'}
corpus.load_domain_chained_model()
print(corpus.list_chainedbstates())
# Create interactive instruments for the chained dimension
corpus.domain_map(chained=True)
corpus.domain_network(doc_level=1, ter_level=None, ext_level=1)
corpus.domain_network(doc_level=1, ter_level=1, ext_level=1)
# The `edges` parameter applies to both topics and the chained dimension
corpus.domain_network(doc_level=2, ter_level=None, ext_level=1, edges="specific")
corpus.domain_network(doc_level=2, ter_level=1, ext_level=1, edges="common")
if 3 in corpus.dblocks:
corpus.subxblocks_tables(xbtype="doc", xlevel=3, xb=None, ybtype="ext")
Help: the domain map document provides a "Help" tab explaining how to navigate and read it, which is also useful to understand the other interfaces.
Filters: Create filtered visualizations to show only a selected group of domains in maps and networks, with Corpus.set_sample()
or with the xb_selection
parameter to domain_network()
.
Filtered chaining: With Corpus.set_sample()
, perform selective chaining, whereby a domain-chained model is fit by considering only a selected group of domains, instead of the entire corpus, in order to understand the local associations of metadata dimensions.
Complex chaining: Calls to Corpus.set_chain()
may pass in the matcher
parameter a path to a .json
file containing a dictionary. Nodes of the chained dimension will then correspond to the keys in the dictionary, and links will be established by searching for a key's value, as a regular expression, in the column passed as the first parameter.
Better network map: An entirely new network map function with many improvements and new features is under development and can be tried from from sashimi.blocks.better_network_map import network_map
. It will eventually replace the current network map.
This module provides four main classes:
class Corpus
Methods to load and preprocess corpora, plus some descriptive statistics.
class GraphModels
(user-facing class, inherits Corpus and Blocks)
Methods to fit Stochastic Block Models to corpora through either document-term or document-metadata graphs, yielding domain-topic and domain-chained models, respectively.
class Blocks
Methods to build interactive domain maps, networks, tables, and other interfaces.
class VectorModels
[currently deprecated]
Methods to fit word embedding models to corpora and produce reports, statistics and visualizations.
FAQs
A Python module for mixed-methods qualitative studies of large textual or symbolic corpora, providing applications of statistical models accompanied by tailored interactive visualizations. It affords the detection of both textual and metadata structures by employing stochastic block modeling (SBM) from `graph-tool` or (currently deprecated) word embedding from `gensim`.
We found that sashimi-domains demonstrated a healthy version release cadence and project activity because the last version was released less than a year ago. It has 1 open source maintainer collaborating on the project.
Did you know?
Socket for GitHub automatically highlights issues in each pull request and monitors the health of all your open source dependencies. Discover the contents of your packages and block harmful activity before you install or update your dependencies.
Security News
Maven Central now validates Sigstore signatures, making it easier for developers to verify the provenance of Java packages.
Security News
CISOs are racing to adopt AI for cybersecurity, but hurdles in budgets and governance may leave some falling behind in the fight against cyber threats.
Research
Security News
Socket researchers uncovered a backdoored typosquat of BoltDB in the Go ecosystem, exploiting Go Module Proxy caching to persist undetected for years.