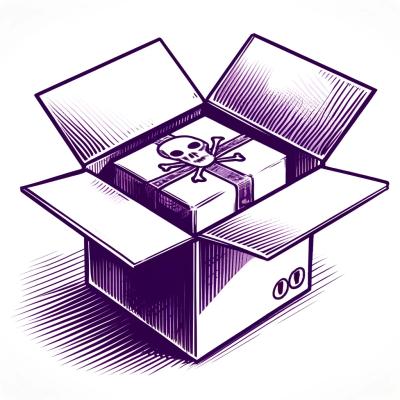
Security News
Research
Data Theft Repackaged: A Case Study in Malicious Wrapper Packages on npm
The Socket Research Team breaks down a malicious wrapper package that uses obfuscation to harvest credentials and exfiltrate sensitive data.
An excuse to play with Rust, but also a neat trick for sklearn!
This package contains some bloom tricks for text pipelines in scikit-learn. To learn more about this trick, check out this blogpost.
You can install it via:
python -m pip install scikit-bloom
And you can import the components via:
from skbloom import BloomVectorizer, BloomishVectorizer, SlowBloomVectorizer
BloomVectorizer().fit(X).transform(X)
BloomishVectorizer().fit(X).transform(X)
The BloomVectorizer
will use rust under the hood for the hashing to construct the bloom representation. The BloomishVectorizer
will just run the HashingVectorizer from scikit-learn multiple times in sequence. The SlowBloomVectorizer
is pretty much the same as the BloomVectizer
in terms of features, but is implemented in Python.
I ran a quick benchmark, which seems to suggest the approach is pretty speedy.
import time
from datasets import load_dataset
from skbloom import BloomVectorizer, BloomishVectorizer, SlowBloomVectorizer
from sklearn.feature_extraction.text import HashingVectorizer
dataset = load_dataset("clinc_oos", "plus")
texts = dataset['train']['text'] * 10
trials = [BloomVectorizer(n_features=10_000),
BloomishVectorizer(n_features=10_000),
SlowBloomVectorizer(n_features=10_000),
HashingVectorizer(n_features=10_000)]
for trial in trials:
tic = time.time()
trial.fit_transform(texts)
toc = time.time()
print(f"{trial.__class_.__name__}: {toc - tic}")
In this benchmark we're creating a
Approach | Time taken | Description |
---|---|---|
BloomVectorizer | 1.562 | The speedy rust implementation |
BloomishVectorizer | 2.111 | Using sklearn's implementation sequentially |
SlowBloomVectorizer | 5.259 | A pure python implementation |
HashingVectorizer | 0.695 | Using sklearn's hashing vectorizer to only hash once |
Note that the HashingVectorizer
is faster here because it only hashes each word once. The other implementations hash it three times.
Just as an extra, you can also choose to run the BloomVectorizer
by just hashing once and when I do that ... it seems to be competative with the HashingVectorizer
.
import time
from datasets import load_dataset
from skbloom import BloomVectorizer, BloomishVectorizer, SlowBloomVectorizer
from sklearn.feature_extraction.text import HashingVectorizer
dataset = load_dataset("clinc_oos", "plus")
texts = dataset['train']['text'] * 10
for feats in [3000, 5000, 10000, 20000, 100_000]:
trials = [BloomVectorizer(n_hash=1, n_features=feats), HashingVectorizer(n_features=feats)]
for trial in trials:
tic = time.time()
trial.fit_transform(texts)
toc = time.time()
print(f"{feats}: {trial.__class__.__name__}: {toc - tic}")
Number of feats | BloomVectorizer | HashingVectorizer |
---|---|---|
3000 | 0.6071 | 0.6864 |
5000 | 0.6092 | 0.6947 |
10000 | 0.6123 | 0.6911 |
20000 | 0.6124 | 0.6918 |
100000 | 0.6108 | 0.6938 |
I want to be careful with suggesting that the BloomVectorizer
is always faster
because the HashingVectorizer
comes with way more features. You can build n-gram representations, just to mention one example, which the BloomVectorizer
does not do. But it does seem like we're in the same ballpark, which is neat consider the implementation was very little effort.
In fairness, while this trick is interesting ... you might be fine just using the HashingVectorizer
that just comes with sklearn. This project works, but it was also an excuse for me to try out rust.
It's a nice motivating example for me to learn a bit of rust, partially because it's a tangible example from a field that I am familiar with. But it's also been a relatively low investment to rewrite an expensive bit of code.
These are mainly some notes for myself.
To install all of this locally;
curl --proto '=https' --tlsv1.2 -sSf https://sh.rustup.rs | sh
python -m pip install uv
uv venv --python 3.12
uv pip install maturin
uv run maturin develop --uv
uv pip install -e .
If you want to make a release, remember to tag before pushing.
git tag v0.2.1
git push origin <branchname>
git push origin --tags
FAQs
Unknown package
We found that scikit-bloom demonstrated a healthy version release cadence and project activity because the last version was released less than a year ago. It has 1 open source maintainer collaborating on the project.
Did you know?
Socket for GitHub automatically highlights issues in each pull request and monitors the health of all your open source dependencies. Discover the contents of your packages and block harmful activity before you install or update your dependencies.
Security News
Research
The Socket Research Team breaks down a malicious wrapper package that uses obfuscation to harvest credentials and exfiltrate sensitive data.
Research
Security News
Attackers used a malicious npm package typosquatting a popular ESLint plugin to steal sensitive data, execute commands, and exploit developer systems.
Security News
The Ultralytics' PyPI Package was compromised four times in one weekend through GitHub Actions cache poisoning and failure to rotate previously compromised API tokens.