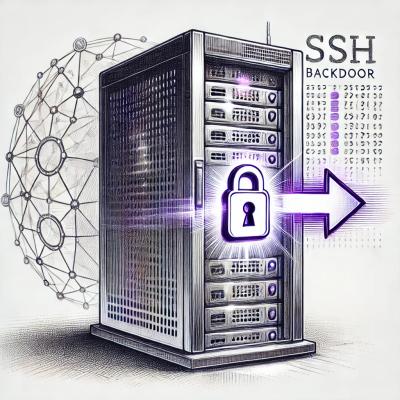
Research
Security News
Malicious npm Packages Inject SSH Backdoors via Typosquatted Libraries
Socket’s threat research team has detected six malicious npm packages typosquatting popular libraries to insert SSH backdoors.
scikit-learn-intelex
Advanced tools
Intel(R) Extension for Scikit-learn is a seamless way to speed up your Scikit-learn application.
With Intel(R) Extension for Scikit-learn you can accelerate your Scikit-learn applications and still have full conformance with all Scikit-Learn APIs and algorithms. This is a free software AI accelerator that brings over 10-100X acceleration across a variety of applications. And you do not even need to change the existing code!
The acceleration is achieved through the use of the Intel(R) oneAPI Data Analytics Library (oneDAL). Patching scikit-learn makes it a well-suited machine learning framework for dealing with real-life problems.
⚠️Intel(R) Extension for Scikit-learn contains scikit-learn patching functionality that was originally available in daal4py package. All future updates for the patches will be available only in Intel(R) Extension for Scikit-learn. We recommend you to use scikit-learn-intelex package instead of daal4py. You can learn more about daal4py in daal4py documentation.
We publish blogs on Medium, so follow us to learn tips and tricks for more efficient data analysis with the help of Intel(R) Extension for Scikit-learn. Here are our latest blogs:
Report issues, ask questions, and provide suggestions using:
You may reach out to project maintainers privately at onedal.maintainers@intel.com
Intel(R) Extension for Scikit-learn is available at the Python Package Index, on Anaconda Cloud in Conda-Forge channel and in Intel channel. Intel(R) Extension for Scikit-learn is also available as a part of Intel® oneAPI AI Analytics Toolkit (AI Kit).
pip install scikit-learn-intelex
conda config --add channels conda-forge
conda config --set channel_priority strict
conda install scikit-learn-intelex
conda config --add channels intel
conda config --set channel_priority strict
conda install scikit-learn-intelex
OS / Python version | Python 3.8 | Python 3.9 | Python 3.10 | Python 3.11 | Python 3.12 |
---|---|---|---|---|---|
Linux | [CPU, GPU] | [CPU, GPU] | [CPU, GPU] | [CPU, GPU] | [CPU, GPU] |
Windows | [CPU, GPU] | [CPU, GPU] | [CPU, GPU] | [CPU, GPU] | [CPU, GPU] |
OS / Python version | Python 3.8 | Python 3.9 | Python 3.10 | Python 3.11 | Python 3.12 |
---|---|---|---|---|---|
Linux | [CPU] | [CPU] | [CPU] | [CPU] | [CPU] |
Windows | [CPU] | [CPU] | [CPU] | [CPU] | [CPU] |
OS / Python version | Python 3.8 | Python 3.9 | Python 3.10 | Python 3.11 | Python 3.12 |
---|---|---|---|---|---|
Linux | [CPU, GPU] | [CPU, GPU] | [CPU, GPU] | [CPU, GPU] | [CPU, GPU] |
Windows | [CPU, GPU] | [CPU, GPU] | [CPU, GPU] | [CPU, GPU] | [CPU, GPU] |
⚠️ Note: GPU support is an optional dependency. Required dependencies for GPU support will not be downloaded. You need to manually install dpcpp_cpp_rt package.
pip install --upgrade dpcpp_cpp_rt
conda install dpcpp_cpp_rt -c intel
You can build the package from sources as well.
Intel CPU optimizations patching
import numpy as np
from sklearnex import patch_sklearn
patch_sklearn()
from sklearn.cluster import DBSCAN
X = np.array([[1., 2.], [2., 2.], [2., 3.],
[8., 7.], [8., 8.], [25., 80.]], dtype=np.float32)
clustering = DBSCAN(eps=3, min_samples=2).fit(X)
Intel GPU optimizations patching
import numpy as np
import dpctl
from sklearnex import patch_sklearn, config_context
patch_sklearn()
from sklearn.cluster import DBSCAN
X = np.array([[1., 2.], [2., 2.], [2., 3.],
[8., 7.], [8., 8.], [25., 80.]], dtype=np.float32)
with config_context(target_offload="gpu:0"):
clustering = DBSCAN(eps=3, min_samples=2).fit(X)
Configurations:
python runner.py --configs configs/blogs/skl_conda_config.json -–report
python runner.py --configs configs/blogs/skl_conda_config.json -–report --no-intel-optimized
Intel(R) Extension for Scikit-learn patching affects performance of specific Scikit-learn functionality. Refer to the list of supported algorithms and parameters for details. In cases when unsupported parameters are used, the package fallbacks into original Scikit-learn. If the patching does not cover your scenarios, submit an issue on GitHub.
⚠️ We support optimizations for the last four versions of scikit-learn. The latest release of scikit-learn-intelex-2024.0.X supports scikit-learn 1.0.X, 1.1.X, 1.2.X and 1.3.X.
To find out which implementation of the algorithm is currently used (Intel(R) Extension for Scikit-learn or original Scikit-learn), set the environment variable:
export SKLEARNEX_VERBOSE=INFO
set SKLEARNEX_VERBOSE=INFO
For example, for DBSCAN you get one of these print statements depending on which implementation is used:
SKLEARNEX INFO: sklearn.cluster.DBSCAN.fit: running accelerated version on CPU
SKLEARNEX INFO: sklearn.cluster.DBSCAN.fit: fallback to original Scikit-learn
FAQs
Intel(R) Extension for Scikit-learn is a seamless way to speed up your Scikit-learn application.
We found that scikit-learn-intelex demonstrated a healthy version release cadence and project activity because the last version was released less than a year ago. It has 2 open source maintainers collaborating on the project.
Did you know?
Socket for GitHub automatically highlights issues in each pull request and monitors the health of all your open source dependencies. Discover the contents of your packages and block harmful activity before you install or update your dependencies.
Research
Security News
Socket’s threat research team has detected six malicious npm packages typosquatting popular libraries to insert SSH backdoors.
Security News
MITRE's 2024 CWE Top 25 highlights critical software vulnerabilities like XSS, SQL Injection, and CSRF, reflecting shifts due to a refined ranking methodology.
Security News
In this segment of the Risky Business podcast, Feross Aboukhadijeh and Patrick Gray discuss the challenges of tracking malware discovered in open source softare.