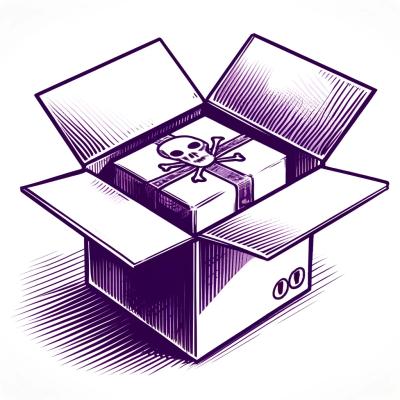
Security News
Research
Data Theft Repackaged: A Case Study in Malicious Wrapper Packages on npm
The Socket Research Team breaks down a malicious wrapper package that uses obfuscation to harvest credentials and exfiltrate sensitive data.
|github| |codecov| |docs|
.. |codecov| image:: https://codecov.io/gh/15five/scim2-filter-parser/branch/master/graph/badge.svg :target: https://codecov.io/gh/15five/scim2-filter-parser
.. |docs| image:: https://readthedocs.org/projects/scim2-filter-parser/badge/?version=latest :target: https://scim2-filter-parser.readthedocs.io/en/latest/?badge=latest :alt: Documentation Status
.. |github| image:: https://github.com/15five/scim2-filter-parser/workflows/CI%2FCD/badge.svg :target: https://github.com/15five/scim2-filter-parser/actions?workflow=CI%2FCD :alt: CI/CD Status
SCIM 2.0 defines queries that look like this::
'emails[type eq "work" and value co "@example.com"] or ims[type eq "xmpp" and value co "@foo.com"]'
These can be hard to work with and covert into SQL to run against a database.
That's where SCIM 2.0 Filter Parser (SFP) can help.
SFP is broken up into four modules, each handling a different part of translating a SCIM call into a SQL query.
The first step is tokenization or lexical analysis where the filter query is broken down into many tokens that make it up.
::
sfp-lexer 'emails[type eq "work" and value co "@example.com"] or ims[type eq "xmpp" and value co "@foo.com"]'
Token(type='ATTRNAME', value='emails', lineno=1, index=0)
Token(type='LBRACKET', value='[', lineno=1, index=6)
Token(type='ATTRNAME', value='type', lineno=1, index=7)
Token(type='EQ', value='eq', lineno=1, index=12)
Token(type='COMP_VALUE', value='work', lineno=1, index=15)
Token(type='AND', value='and', lineno=1, index=22)
Token(type='ATTRNAME', value='value', lineno=1, index=26)
Token(type='CO', value='co', lineno=1, index=32)
Token(type='COMP_VALUE', value='@example.com', lineno=1, index=35)
Token(type='RBRACKET', value=']', lineno=1, index=49)
Token(type='OR', value='or', lineno=1, index=51)
Token(type='ATTRNAME', value='ims', lineno=1, index=54)
Token(type='LBRACKET', value='[', lineno=1, index=57)
Token(type='ATTRNAME', value='type', lineno=1, index=58)
Token(type='EQ', value='eq', lineno=1, index=63)
Token(type='COMP_VALUE', value='xmpp', lineno=1, index=66)
Token(type='AND', value='and', lineno=1, index=73)
Token(type='ATTRNAME', value='value', lineno=1, index=77)
Token(type='CO', value='co', lineno=1, index=83)
Token(type='COMP_VALUE', value='@foo.com', lineno=1, index=86)
Token(type='RBRACKET', value=']', lineno=1, index=96)
The second step is to convert that series of tokens into a abstract syntax tree.
::
sfp-parser 'emails[type eq "work" and value co "@example.com"] or ims[type eq "xmpp" and value co "@foo.com"]'
Filter(expr=LogExpr, negated=False, namespace=None)
LogExpr(op='or', expr1=Filter, expr2=Filter)
Filter(expr=Filter, negated=False, namespace=None)
Filter(expr=Filter, negated=False, namespace=AttrPath)
Filter(expr=LogExpr, negated=False, namespace=None)
LogExpr(op='and', expr1=Filter, expr2=Filter)
Filter(expr=AttrExpr, negated=False, namespace=None)
AttrExpr(value='eq', attr_path=AttrPath, comp_value=CompValue)
AttrPath(attr_name='type', sub_attr=None, uri=None)
CompValue(value='work')
Filter(expr=AttrExpr, negated=False, namespace=None)
AttrExpr(value='co', attr_path=AttrPath, comp_value=CompValue)
AttrPath(attr_name='value', sub_attr=None, uri=None)
CompValue(value='@example.com')
AttrPath(attr_name='emails', sub_attr=None, uri=None)
Filter(expr=Filter, negated=False, namespace=None)
Filter(expr=Filter, negated=False, namespace=AttrPath)
Filter(expr=LogExpr, negated=False, namespace=None)
LogExpr(op='and', expr1=Filter, expr2=Filter)
Filter(expr=AttrExpr, negated=False, namespace=None)
AttrExpr(value='eq', attr_path=AttrPath, comp_value=CompValue)
AttrPath(attr_name='type', sub_attr=None, uri=None)
CompValue(value='xmpp')
Filter(expr=AttrExpr, negated=False, namespace=None)
AttrExpr(value='co', attr_path=AttrPath, comp_value=CompValue)
AttrPath(attr_name='value', sub_attr=None, uri=None)
CompValue(value='@foo.com')
AttrPath(attr_name='ims', sub_attr=None, uri=None)
The third step is to transpile this AST into a language of our choice. The above query is transpiled to SQL below.
::
sfp-transpiler 'emails[type eq "work" and value co "@example.com"] or ims[type eq "xmpp" and value co "@foo.com"]'
((emails.type = {0}) AND (emails.value LIKE {1})) OR ((ims.type = {2}) AND (ims.value LIKE {3}))
{0: 'work', 1: '%@example.com%', 2: 'xmpp', 3: '%@foo.com%'}
The fourth step is to take what is a segment of a SQL WHERE clause and complete the rest of the SQL query.
::
sfp-query 'emails[type eq "work" and value co "@example.com"] or ims[type eq "xmpp" and value co "@foo.com"]'
>>> DO NOT USE THIS OUTPUT DIRECTLY
>>> SQL INJECTION ATTACK RISK
>>> SQL PREVIEW:
SELECT DISTINCT users.*
FROM users
LEFT JOIN emails ON emails.user_id = users.id
LEFT JOIN schemas ON schemas.user_id = users.id
WHERE ((emails.type = work) AND (emails.value LIKE %@example.com%)) OR ((ims.type = xmpp) AND (ims.value LIKE %@foo.com%));
Please note that SFP does not build SQL queries with parameters pre-injected.
That would create a SQL injection attack vulnerability. Instead a SQLQuery
object is created and can be forced to display itself as seen above
by print
ing the query object.
::
pip install scim2-filter-parser
# Or ...
pip install scim2-filter-parser[django-query]
Although command line shims are provided, the library is intended to be used
programmatically. Users of the library should instantiate the
scim2_filter_parser.queries.SQLQuery
class with an attribute map and optionally
any joins necessary to make all required fields accessible in the query.
For example, if user information is stored in the users
table and email
information is stored in a different table emails
, then the attribute map
and the joins might be defined as so::
from scim2_filter_parser.queries import SQLQuery
attr_map = {
('userName', None, None): 'users.username',
('name', 'familyName', None): 'users.family_name',
('meta', 'lastModified', None): 'users.update_ts',
('emails', None, None): 'emails.address',
('emails', 'value', None): 'emails.address',
}
joins = (
'LEFT JOIN emails ON emails.user_id = users.id',
)
filter_ = 'name.familyName co "Simpson" or emails.value eq "lisa@example.com"'
q = SQLQuery(filter_, 'users', attr_map, joins)
q.sql # Will be...
SELECT DISTINCT users.*
FROM users
LEFT JOIN emails ON emails.user_id = users.id
WHERE (users.family_name LIKE %s) OR (emails.address = %s);
q.params # Will be...
['%Simpson%', 'lisa@example.com']
The attribute_map (attr_map
) is a mapping of SCIM attribute, subattribute,
and schema uri to a table field. You will need to customize this to your
particular database schema.
The SQLQuery.sql
method returns SQL that can be used as the first
argument in a call to cursor.execute()
with your favorite DB engine.
If you are using a database that requires a replacement character other than '%s',
then you can subclass the SQLQuery
class and override the placeholder
class
level variable. See the query module and unit tests for an example of this subclassing
with SQLite.
The SQLQuery.params
method returns a list of items that can be used as the
second argument in a call to cursor.execute()
.
If you would like to produce a Django Q
_ object instead of a raw SQL query, you can pass
a SCIM filter query and attribute map to the get_query
function from the module
scim2_filter_parser.transpilers.django_q_object
. For example::
get_query(scim_query: str, attr_map: Mapping)
This Q object can then be passed to a Django filter query like so::
query = get_query(scim_query, attr_map)
User.objects.filter(query)
Please note that you will need to install the Django Query extra like for this feature to be available::
pip install scim2-filter-parser[django-query]
.. _Django Q
: https://docs.djangoproject.com/en/3.1/topics/db/queries/#complex-lookups-with-q-objects
SFP is pretty fast. Check out the speed_test.py script for details on the long and short filter queries tested. SFP transpiled a short filter query into SQL in under 54 microseconds. For a longer query, SFP only took 273 microseconds.
::
➜ scim2-filter-parser git:(master) ✗ python -m timeit -s "import speed_test" "speed_test.short()"
10000 loops, best of 3: 53.8 usec per loop
➜ scim2-filter-parser git:(master) ✗ python -m timeit -s "import speed_test" "speed_test.long()"
1000 loops, best of 3: 273 usec per loop
Since this project is relatively stable, time is only dedicated to it on Fridays. Thus if you issue a PR, bug, etc, please note that it may take a week before we get back to you. Thanks you for your patience.
This project uses Poetry to manage dependencies, etc. Thus to install the necessary tools when developing, run:
::
poetry install -v --extras "django-query"
.. |tests| image:: https://github.com/15five/scim2-filter-parser/workflows/CI%2FCD/badge.svg :target: https://github.com/15five/scim2-filter-parser/actions
https://github.com/15five/scim2-filter-parser/actions
Tests are typically run locally with tox
(https://tox.wiki/). Tox will test
all supported versions of Python.
tox
To run the test suite with a single version of Python (the version you created the virtualenv with), run:
::
poetry run pytest tests/
.. |coverage| image:: https://codecov.io/gh/15five/scim2-filter-parser/graph/badge.svg :target: https://codecov.io/gh/15five/scim2-filter-parser
https://codecov.io/gh/15five/scim2-filter-parser
::
tox -e coverage
https://pypi.org/project/scim2-filter-parser/
To deploy this package to PyPI, run:
::
poetry build
poetry publish
FAQs
A customizable parser/transpiler for SCIM2.0 filters.
We found that scim2-filter-parser demonstrated a healthy version release cadence and project activity because the last version was released less than a year ago. It has 2 open source maintainers collaborating on the project.
Did you know?
Socket for GitHub automatically highlights issues in each pull request and monitors the health of all your open source dependencies. Discover the contents of your packages and block harmful activity before you install or update your dependencies.
Security News
Research
The Socket Research Team breaks down a malicious wrapper package that uses obfuscation to harvest credentials and exfiltrate sensitive data.
Research
Security News
Attackers used a malicious npm package typosquatting a popular ESLint plugin to steal sensitive data, execute commands, and exploit developer systems.
Security News
The Ultralytics' PyPI Package was compromised four times in one weekend through GitHub Actions cache poisoning and failure to rotate previously compromised API tokens.