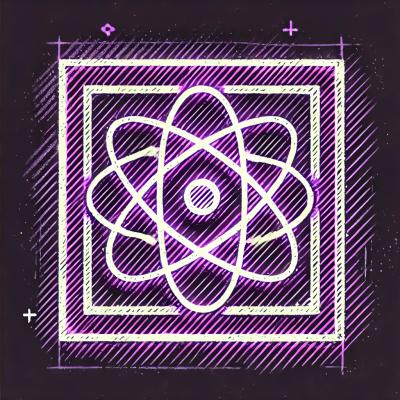
Security News
Create React App Officially Deprecated Amid React 19 Compatibility Issues
Create React App is officially deprecated due to React 19 issues and lack of maintenance—developers should switch to Vite or other modern alternatives.
OS | Status |
---|---|
Linux | |
Mac | |
Windows |
A Python package implementing Structural Causal Models (SCM).
The library uses the CAS library SymPy to allow the user to state arbitrary assignment functions and noise distributions as supported by SymPy and builds the DAG with networkx.
It supports the features:
and by extension all methods on a DAG provided by networkx after accessing the member variable dag
Either install via pip
pip install scmodels
or via cloning the repository and running the installation locally
git clone https://github.com/maichmueller/scmodels
cd scmodels
pip install .
In order to declare the SCM
$X ~ \leftarrow ~ Z ~ \rightarrow ~ V ~ \rightarrow ~ W$
$\downarrow ~ ~ \swarrow$
$Y$
with the assignments
$Z \sim \text{LogLogistic} (\alpha=1,\beta=1)$
$X=3Z^2 \cdot N \quad [N \sim \text{LogNormal}(\mu=1,\sigma=1)]$
$Y = 2Z + \sqrt{X} + N \quad [N \sim \text{Normal}(\mu=2,\sigma=1)]$
$V = Z + N^P \quad [N \sim \text{Normal}(\mu=0,\sigma=1), P \sim \text{Ber}(0.5)]$
$W = V + \exp(T) - \log(M) * N \quad [N \sim \text{Normal}(\mu=0,\sigma=1), T \sim \text{StudentT}(\nu = 0.5), M \sim \text{Exp}(\lambda = 1)]$
there are 2 different ways implemented.
Describe the assignments as strings of the form:
'VAR = FUNC(Noise, parent1, parent2, ...), Noise ~ DistributionXYZ'
Note that in this case, one must not restate the noise symbol string in the distribution (as would otherwise be necessary in constructing sympy distributions).
from scmodels import SCM
assignment_seq = [
"Z = M; M ~ LogLogistic(alpha=1, beta=1)",
"X = N * 3 * Z ** 2; N ~ LogNormal(mean=1, std=1)",
"Y = P + 2 * Z + sqrt(X); P ~ Normal(mean=2, std=1)",
"V = N**P + Z; N ~ Normal(0,1) / P ~ Bernoulli(0.5)",
"W = exp(T) - log(M) * N + V; M ~ Exponential(1) / T ~ StudentT(0.5) / N ~ Normal(0, 2)",
]
myscm = SCM(assignment_seq)
Agreements:
P ~ Normal(mean=2, std=1)
) has to align with the noise variable
name (P
) of the assignment string.W
in the above example.
The noise model string segment must specify all individual noise models separated by a '/'One can construct the SCM via an assignment map with the variables as keys and a tuple defining the assignment and the noise.
To refer to SymPy's string parsing capability (this includes numpy functions) provide a dict entry with 2-tuples as values of the form:
'var': ('assignment string', noise)
from sympy.stats import LogLogistic, LogNormal, Normal, Bernoulli, StudentT, Exponential
assignment_map = {
"Z": (
"M",
LogLogistic("M", alpha=1, beta=1)
),
"X": (
"N * 3 * Z ** 2",
LogNormal("N", mean=1, std=1),
),
"Y": (
"P + 2 * Z + sqrt(X)",
Normal("P", mean=2, std=1),
),
"V": (
"N**P + Z",
[Normal("N", 0, 1), Bernoulli("P", 0.5)]
),
"W": (
"exp(T) - log(M) * N + V",
[Normal("N", 0, 1), StudentT("T", 0.5), Exponential("M", 1)]
)
}
myscm2 = SCM(assignment_map)
Agreements:
Normal("N", mean=2, std=1)
) must align with the noise variable
name (N
) in the assignment string.[]
, or tuple ()
)One can also declare the SCM via specifying the variable assignment in a dictionary with the variables as keys and as values a sequence of length 3 of the form:
'var': (['parent1', 'parent2', ...], Callable, Noise)
This allows the user to supply functions that are not limited to predefined function sets of scmodels
' dependencies.
import numpy as np
def Y_assignment(p, z, x):
return p + 2 * z + np.sqrt(x)
functional_map = {
"Z": (
[],
lambda m: m,
LogLogistic("M", alpha=1, beta=1)
),
"X": (
["Z"],
lambda n, z: n * 3 * z ** 2,
LogNormal("N", mean=1, std=1),
),
"Y": (
["Z", "X"],
Y_assignment,
Normal("P", mean=2, std=1),
),
"V": (
["Z"],
lambda n, p, z: n ** p + z,
[Normal("N", mean=0, std=1), Bernoulli("P", p=0.5)]
),
"W": (
["V"],
lambda n, t, m, v: np.exp(m) - np.log(m) * n + v,
[Normal("N", mean=0, std=1), StudentT("T", nu=0.5), Exponential("M", rate=1)]
)
}
myscm3 = SCM(functional_map)
Agreements:
None
, then they have to be omitted from the parameter list of the callable.The SCM supports a form of informative printing of its current setup, which includes mentioning active interventions and the assignments.
print(myscm)
Structural Causal Model of 5 variables: Z, X, V, Y, W
Variables with active interventions: []
Assignments:
Z := f(M) = M [ M ~ LogLogistic(alpha=1, beta=1) ]
X := f(N, Z) = N * 3 * Z ** 2 [ N ~ LogNormal(mean=1, std=1) ]
Y := f(P, Z, X) = P + 2 * Z + sqrt(X) [ P ~ Normal(mean=2, std=1) ]
V := f(N, P, Z) = N**P + Z [ N ~ Normal(mean=0, std=1), P ~ Bernoulli(p=0.5, succ=1, fail=0) ]
W := f(M, T, N, V) = exp(T) - log(M) * N + V [ M ~ Exponential(rate=1), T ~ StudentT(nu=0.5), N ~ Normal(mean=0, std=2) ]
In the case of custom callable assignments, the output is less informative
print(myscm3)
Structural Causal Model of 5 variables: Z, X, V, Y, W
Variables with active interventions: []
Assignments:
Z := f(M) = __unknown__ [ M ~ LogLogistic(alpha=1, beta=1) ]
X := f(N, Z) = __unknown__ [ N ~ LogNormal(mean=1, std=1) ]
Y := f(P, Z, X) = __unknown__ [ P ~ Normal(mean=2, std=1) ]
V := f(N, P, Z) = __unknown__ [ N ~ Normal(mean=0, std=1), P ~ Bernoulli(p=0.5, succ=1, fail=0) ]
W := f(N, T, M, V) = __unknown__ [ N ~ Normal(mean=0, std=1), T ~ StudentT(nu=0.5), M ~ Exponential(rate=1) ]
One can easily perform interventions on the variables, e.g. a Do-intervention or also general interventions, which remodel the connections, assignments, and noise distributions. For general interventions, the passing structure is dict of the following form:
{var: (New Parents (Optional), New Assignment (optional), New Noise (optional))}
Any part of the original variable state, that is meant to be left unchanged, has to be passed as None
.
E.g. to assign a new callable assignment to variable X
without changing parents or noise, one would call:
my_new_callable = lambda n, z: n + z
myscm.intervention({"X": (None, my_new_callable, None)})
For the example of a do-intervention $\text{do}(X=1)$, the helper method do_intervention
is provided. Its counterpart for noise interventions is soft_intervention
:
myscm.do_intervention([("X", 1)])
from sympy.stats import FiniteRV
myscm.soft_intervention([("X", FiniteRV(myscm["X"].noise[0].name, density={-1: .5, 1: .5}))])
Refer to the sympy
docs for more information on building random variables.
Calling undo_intervention
restores the original state of all variables.
If variables are specified (variables=['X', 'Y']
), any interventions on only those variables are undone.
myscm.undo_intervention(variables=["X"])
The SCM allows drawing as many samples as needed through the method myscm.sample(n)
.
n = 5
samples = myscm.sample(n)
display_data(samples)
Z | V | X | W | Y | |
---|---|---|---|---|---|
0 | 0.854202 | 0.193336 | 15.9145 | 1.60315 | 7.55694 |
1 | 0.634422 | 0.951119 | 2.43486 | 5.59541 | 3.6478 |
2 | 1.02354 | 2.02354 | 4.11357 | 2.12862 | 6.23834 |
3 | 0.376173 | 2.2962 | 0.252316 | 2.83333 | 3.64392 |
4 | 4.19568 | 2.894 | 36.2844 | 3.07636 | 16.4083 |
If infinite sampling is desired, one can also receive a sampling generator through
container = {var: [] for var in myscm}
sampler = myscm.sample_iter(container)
container
is an optional target dictionary to store the computed samples in.
for i in range(n):
next(sampler)
display_data(container)
Z | V | X | W | Y | |
---|---|---|---|---|---|
0 | 0.245579 | 0.814717 | 1.59715 | 2.10267 | 2.26984 |
1 | 7.88344 | 8.88344 | 122.197 | 1.90523e+13 | 29.1743 |
2 | 1.26534 | 1.29397 | 12.5345 | 0.523827 | 8.77073 |
3 | 1.37904 | 2.37904 | 13.327 | 2.08063 | 9.14935 |
4 | 0.230563 | -0.966714 | 0.354393 | -1.22201 | 3.09432 |
If the target container is not provided, the generator returns a new dict
for every sample.
sample = next(myscm.sample_iter())
display_data(sample)
Z | V | X | W | Y | |
---|---|---|---|---|---|
0 | 2.02308 | 3.1259 | 59.8718 | 4.2209 | 13.7312 |
If you have graphviz installed, you can plot the DAG by calling
myscm.plot(node_size=1000, alpha=1)
FAQs
Structural Causal Models
We found that scmodels demonstrated a healthy version release cadence and project activity because the last version was released less than a year ago. It has 1 open source maintainer collaborating on the project.
Did you know?
Socket for GitHub automatically highlights issues in each pull request and monitors the health of all your open source dependencies. Discover the contents of your packages and block harmful activity before you install or update your dependencies.
Security News
Create React App is officially deprecated due to React 19 issues and lack of maintenance—developers should switch to Vite or other modern alternatives.
Security News
Oracle seeks to dismiss fraud claims in the JavaScript trademark dispute, delaying the case and avoiding questions about its right to the name.
Security News
The Linux Foundation is warning open source developers that compliance with global sanctions is mandatory, highlighting legal risks and restrictions on contributions.