Shmessy

If your data is messy - Use Shmessy!
Shmessy designed to deal with messy pandas dataframes.
We all knows the frustrating times when we as analysts or data-engineers should handle messy dataframe and analyze them by ourselves.
The goal of this tiny tool is to identify the physical / logical data type for each Dataframe column.
It based on fast validators that will validate the data (Based on a sample) against regex / pydantic types or any additional validation function that you want to implement.
As you understand, this tool was designed to deal with dirty data,
ideally developed for Dataframes generated from CSV / Flat files or any source that doesn't contain strict schema.
Installation
pip install shmessy
Usage
You have two ways to use this tool
Identify the Dataframe schema
import pandas as pd
from shmessy import Shmessy
df = pd.read_csv('/tmp/file.csv')
inferred_schema = Shmessy().infer_schema(df)
Output (inferred_schema dump):
{
"infer_duration_ms": 12,
"columns": [
{
"field_name": "id",
"source_type": "Integer",
"inferred_type": "Integer"
},
{
"field_name": "email_value",
"source_type": "String",
"inferred_type": "Email"
},
{
"field_name": "date_value",
"source_type": "String",
"inferred_type": "Date",
"inferred_pattern": "%d-%m-%Y"
},
{
"field_name": "datetime_value",
"source_type": "String",
"inferred_type": "Datetime",
"inferred_pattern": "%Y/%m/%d %H:%M:%S"
},
{
"field_name": "yes_no_data",
"source_type": "String",
"inferred_type": "Boolean",
"inferred_pattern": [
"YES",
"NO"
]
},
{
"field_name": "unix_value",
"source_type": "Integer",
"inferred_type": "UnixTimestamp",
"inferred_pattern": "ms"
},
{
"field_name": "ip_value",
"source_type": "String",
"inferred_type": "IPv4"
}
]
}
Identify and fix Pandas Dataframe
This piece of code will change the column types of the input Dataframe according to Messy infer.
import pandas as pd
from shmessy import Shmessy
df = pd.read_csv('/tmp/file.csv')
fixed_df = Shmessy().fix_schema(df)
Original Dataframe
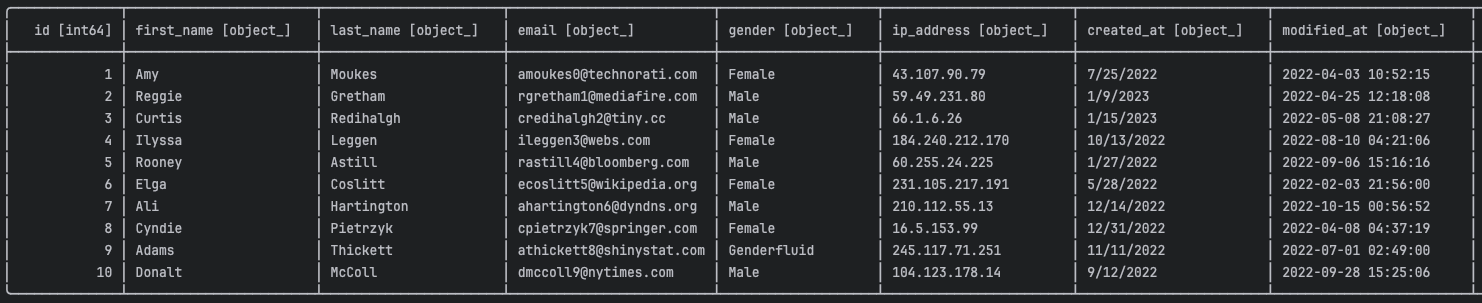
Fixed Dataframe
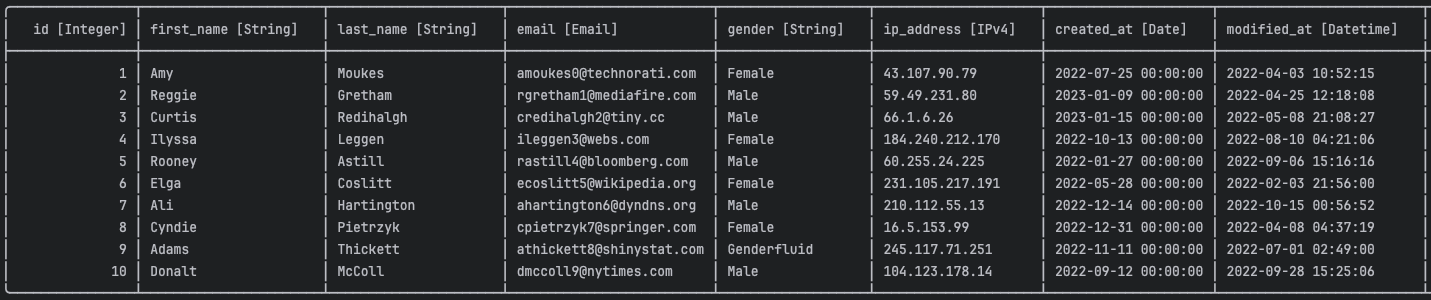
Read Messy CSV file
from shmessy import Shmessy
df = Shmessy().read_csv('/tmp/file.csv')
Original file

Fixed Dataframe

API
Constructor
shmessy = Shmessy(
sample_size: Optional[int] = 1000,
reader_encoding: Optional[str] = "UTF-8",
locale_formatter: Optional[str] = "en_US",
use_random_sample: Optional[bool] = True,
types_to_ignore: Optional[List[str]] = None,
max_columns_num: Optional[int] = 500,
fallback_to_string: Optional[bool] = False,
fallback_to_null: Optional[bool] = False,
use_csv_sniffer: Optional[bool] = True,
fix_column_names: Optional[bool] = False,
)
read_csv
shmessy.read_csv(filepath_or_buffer: Union[str, TextIO, BinaryIO]) -> DataFrame
infer_schema
shmessy.infer_schema(df: Dataframe) -> ShmessySchema
fix_schema
shmessy.fix_schema(df: Dataframe) -> DataFrame
get_inferred_schema
shmessy.get_inferred_schema() -> ShmessySchema