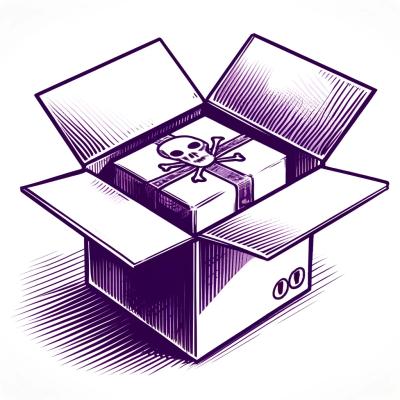
Security News
Research
Data Theft Repackaged: A Case Study in Malicious Wrapper Packages on npm
The Socket Research Team breaks down a malicious wrapper package that uses obfuscation to harvest credentials and exfiltrate sensitive data.
smallab (Small Lab) is an experiment framework designed to be easy to use with your experiment
:small_blue_diamond: :microscope: :small_blue_diamond:
smallab (Small Lab) is an experiment framework designed to be easy to use with your experiment.
The code in this repo should be understandable as a whole in at most 10 minutes.
pip install smallab
or clone here from source
https://smallab.readthedocs.io/en/latest/
Check out
the examples folder
or
demo.py
(Copied here)
import typing
import random
import os
import dill
#What you need to import from smallab
from smallab.experiment_types.experiment import Experiment
from smallab.runner.runner import ExperimentRunner
#Write a simple experiment
class SimpleExperiment(Experiment):
#Need to implement this method, will be passed the specification
#Return a dictionary of results
def main(self, specification: typing.Dict) -> typing.Dict:
random.seed(specification["seed"])
for i in range(specification["num_calls"]): #Advance the random number generator some amount
random.random()
if "fail" in specification and specification["fail"]:
raise Exception()
return {"number":random.random()}
runner = ExperimentRunner()
#Optional: Email yourself when the whole batch is done
#Read https://stackoverflow.com/questions/5619914/sendmail-errno61-connection-refused about how to start an stmp serevr
from smallab.utilities.email_hooks import EmailCallbackBatchOnly
runner.attach_callbacks([EmailCallbackBatchOnly("test@test.com",40)])
#Take it back off since we don't actually want to bother Mr. Test
runner.attach_callbacks([])
#Set the specifications for our experiments, the author reccomends reading this from a json file!
specifications = [{"seed": 1,"num_calls":1}, {"seed":2,"num_calls":1}]
#Fire off the experiment
runner.run("random_number",specifications,SimpleExperiment())
#Read back our results
for root,_,files in os.walk(runner.get_save_directory("random_number")):
for fname in files:
if ".pkl" in fname:
with open(os.path.join(root, fname), "rb") as f:
results = dill.load(f)
print(results["specification"]["seed"])
print(results["result"]["number"])
from smallab.specification_generator import SpecificationGenerator
#If you want to run a lot of experiments but not manual write out each one, use the specification generator.
#Note: This is also JSON serializable, so you could store this in a json file
generation_specification = {"seed":[1,2,3,4,5,6,7,8],"num_calls":[1,2,3]}
#Call the generate method. Will create the cross product.
specifications = SpecificationGenerator().generate(generation_specification)
print(specifications)
runner.run("random_number_from_generator",specifications,SimpleExperiment(),continue_from_last_run=True)
#Read back our results
for root,_,files in os.walk(runner.get_save_directory("random_number_from_generator")):
for fname in files:
if ".pkl" in fname:
with open(os.path.join(root, fname), "rb") as f:
results = dill.load(f)
print(results["specification"]["seed"])
print(results["result"]["number"])
#If you have an experiment you want run on a lot of computers you can use the MultiComputerGenerator
#You assign each computer a number from 0..number_of_computers-1 and it gives each computer every number_of_computerth specification
from smallab.specification_generator import MultiComputerGenerator
all_specifications = SpecificationGenerator().from_json_file('test.json')
g1 = MultiComputerGenerator(0,2)
g2 = MultiComputerGenerator(1,2)
specifications_1 = g1.from_json_file("test.json")
specifications_2 = g2.from_json_file("test.json")
assert len(specifications_1) + len(specifications_2) == len(all_specifications)
#Need to freeze the sets in order to do set manipulation on dictionaries
specifications_1 = set([frozenset(sorted(x.items())) for x in specifications_1])
specifications_2 = set([frozenset(sorted(x.items())) for x in specifications_2])
all_specifications = set([frozenset(sorted(x.items())) for x in all_specifications])
#This will generate two disjoint sets of specifications
assert specifications_1.isdisjoint(specifications_2)
#That together make the whole specification
assert specifications_1.union(specifications_2) == all_specifications
#You can use the provided logging callbacks to log completion and failure of specific specifcations
from smallab.utilities import logger_callbacks
runner.attach_callbacks([LoggingCallback()])
runner.run('with_logging',SpecificationGenerator().from_json_file("test.json"),SimpleExperiment(),continue_from_last_run=True)
The ExperimentRunner
class is passed a list of dictionaries of specifications.
These dictionaries need to be json serializable.
The ExperimentRunner
looks at the completed.json
in the folder for the batch name (the name parameter of the .run
method) and computes which experiments need to be run.
The experiments that need to run are the specifications not in the completed.json
.
The ExperimentRunner
begins runnning the batch either in parallel or single threaded.
If the parallel implementation is used each specification is joblib
's threaded backend.
Once all experiments are either completed or failed (they threw an exception) the results are saved as a pickle
file.
The results are saved in a dictionary that looks like
{
"specification": <the specification the experiment was passed>,
"result": <what the experiment .main returned>
}
The return value of the experiment .main
function must be pickle
serializable.
The runner has several hooks which are called at different times.
on_specification_complete
called whenever a specification completes running (Ususually a single experiment)on_specification_falure
called whenver a specification fails running (Throws an exception)on_batch_complete
called after runner .run
has finished running, passed all the succesfully completed specificationson_batch_failure
called after runner .run
has finished running, passed all the failed specificationsEach experiment is saved in the following structure
experiment_runs/
<name>/ # The name you provide to runner.run
<specification_hash>/ # A hash of the dictionary you provide as the specification
specification.json # The specification.json
<specification_hash>.pkl # The results dictionary
FAQs
smallab (Small Lab) is an experiment framework designed to be easy to use with your experiment
We found that smallab demonstrated a healthy version release cadence and project activity because the last version was released less than a year ago. It has 1 open source maintainer collaborating on the project.
Did you know?
Socket for GitHub automatically highlights issues in each pull request and monitors the health of all your open source dependencies. Discover the contents of your packages and block harmful activity before you install or update your dependencies.
Security News
Research
The Socket Research Team breaks down a malicious wrapper package that uses obfuscation to harvest credentials and exfiltrate sensitive data.
Research
Security News
Attackers used a malicious npm package typosquatting a popular ESLint plugin to steal sensitive data, execute commands, and exploit developer systems.
Security News
The Ultralytics' PyPI Package was compromised four times in one weekend through GitHub Actions cache poisoning and failure to rotate previously compromised API tokens.