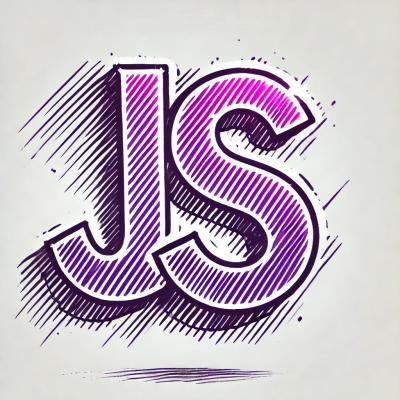
Security News
Oracle Drags Its Feet in the JavaScript Trademark Dispute
Oracle seeks to dismiss fraud claims in the JavaScript trademark dispute, delaying the case and avoiding questions about its right to the name.
The goal is to provide a unified interface to interact with various social analysis tools
The Social Tools library provides a unified interface for interacting with various social analysis tools, including sentiment analysis, toxicity detection, emotion detection, and other natural language processing (NLP) models. With this library, developers can quickly analyze social media text, chat messages, or other forms of unstructured data for toxicity, sentiment, emotions, and more.
To install Social Tools, run:
pip install social-tools
from social_tools import EmotionDetection, SentimentAnalysis, ToxicityDetection
The ToxicityDetection module allows you to analyze text for toxic comments using pre-trained models like HuggingFace's unitary/toxic-bert
.
# Using the unitary/toxic-bert transformer model
tox_detector = ToxicityDetection(tool='transformer', model='unitary/toxic-bert')
result = tox_detector.analyze("I hate you.")
print(result)
This returns:
[{'label': 'toxic', 'score': 0.9475088119506836}]
You can also analyze multiple texts at once:
tox_detector.analyze(["I hate you.", "This is harsh"])
Output:
[
{'label': 'toxic', 'score': 0.9475088119506836},
{'label': 'toxic', 'score': 0.002488125581294298}
]
The SentimentAnalysis module offers several options for analyzing the sentiment of text, including NLTK, SpaCy, and HuggingFace models.
# Using NLTK
sa = SentimentAnalysis(tool='nltk')
result = sa.analyze("This is awesome!")
print(result)
Output:
[{'neg': 0.0, 'neu': 0.313, 'pos': 0.687, 'compound': 0.6588}]
sa = SentimentAnalysis(tool='spacy')
result = sa.analyze("This is awesome!")
print(result)
Output:
[{
'polarity': 1.0,
'subjectivity': 1.0,
'sentiment_assessments': [(['awesome', '!'], 1.0, 1.0, None)]
}]
sa = SentimentAnalysis(tool='huggingface')
result = sa.analyze("This is awesome!")
print(result)
Output:
[{'label': 'POSITIVE', 'score': 0.9998669624328613}]
You can specify a custom HuggingFace transformer model by passing the model name during initialization:
sa = SentimentAnalysis(tool='huggingface', transformer_model="cardiffnlp/twitter-roberta-base-sentiment-latest")
result = sa.analyze("This is awesome!")
print(result)
Output:
[{'label': 'positive', 'score': 0.9813949465751648}]
The EmotionDetection module allows you to detect emotions such as happiness, sadness, and anger. For example, using HuggingFace models:
emotion_detector = EmotionDetection(tool='huggingface')
result = emotion_detector.analyze("I am so happy today!")
print(result)
This will return:
[{'label': 'joy', 'score': 0.95}]
All analyze
functions in each module accept both single strings (str
) and lists of strings (List[str]
) as input:
# Single input
result = sa.analyze("I love this!")
# Multiple inputs
result = sa.analyze(["I love this!", "This is terrible."])
When using a HuggingFace transformer model, you can pass additional parameters during initialization, such as return_all_scores
:
sa = SentimentAnalysis(tool='huggingface', transformer_model="bert-base-uncased", return_all_scores=True)
result = sa.analyze("This is fantastic!")
print(result)
The Social Tools library simplifies the process of analyzing social data by providing multiple sentiment, emotion, and toxicity detection tools in a unified interface. You can integrate popular NLP libraries like NLTK, SpaCy, and HuggingFace models into your workflow seamlessly.
For more information about the supported HuggingFace models and additional parameters, refer to the HuggingFace documentation.
This project would not have been possible without the contributions of the following open-source projects:
transformers
library.spacytextblob
extension for sentiment analysis.A huge thank you to these projects and their respective communities for building the foundational tools that made this library possible.
@misc{socialtool,
title={socialtool},
author={Ridwan Amure},
howpublished={Github. https://github.com/instabaines/social_tools_lib/},
year={2024}
}
if you use the detoxify module in this tool, kindly cite
@misc{Detoxify,
title={Detoxify},
author={Hanu, Laura and {Unitary team}},
howpublished={Github. https://github.com/unitaryai/detoxify},
year={2020}
}
FAQs
The goal is to provide a unified interface to interact with various social analysis tools
We found that social-tools demonstrated a healthy version release cadence and project activity because the last version was released less than a year ago. It has 1 open source maintainer collaborating on the project.
Did you know?
Socket for GitHub automatically highlights issues in each pull request and monitors the health of all your open source dependencies. Discover the contents of your packages and block harmful activity before you install or update your dependencies.
Security News
Oracle seeks to dismiss fraud claims in the JavaScript trademark dispute, delaying the case and avoiding questions about its right to the name.
Security News
The Linux Foundation is warning open source developers that compliance with global sanctions is mandatory, highlighting legal risks and restrictions on contributions.
Security News
Maven Central now validates Sigstore signatures, making it easier for developers to verify the provenance of Java packages.