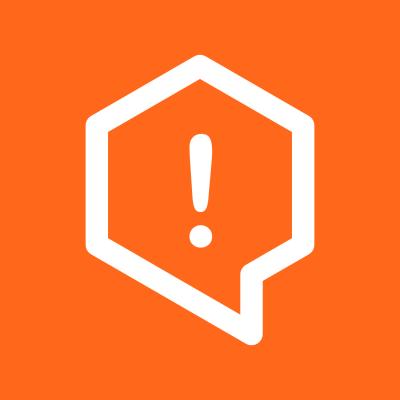
Security News
Fluent Assertions Faces Backlash After Abandoning Open Source Licensing
Fluent Assertions is facing backlash after dropping the Apache license for a commercial model, leaving users blindsided and questioning contributor rights.
spl_transpiler
provides a high-performance, highly portable, convenient tool for adapting common SPL code into PySpark
code when possible, making it easy to migrate from Splunk to other data platforms for log processing.
The project started as a Rust + Python port of Databricks Labs'
spl_transpiler
, but at this point is far more usable and feature-complete than the original.
You can install the basic library with
pip install spl_transpiler
or install all tools using
pip install spl_transpiler[cli,runtime]
You can also use the TUI directly using uvx
or pipx
:
uvx run spl_transpiler
from spl_transpiler import convert_spl_to_pyspark
print(convert_spl_to_pyspark(r"""multisearch
[index=regionA | fields +country, orders]
[index=regionB | fields +country, orders]"""))
# spark.table("regionA").select(
# F.col("country"),
# F.col("orders"),
# ).unionByName(
# spark.table("regionB").select(
# F.col("country"),
# F.col("orders"),
# ),
# allowMissingColumns=True,
# )
For demonstration purposes and ease of use, an interactive CLI is also provided.
pip install spl_transpiler[cli]
python -m spl_transpiler
This provides an in-terminal user interface (using textual
) where you can
type an SPL query and see the converted Pyspark code in real time, alongside a visual representation of how the
transpiler is understanding your query.
The Runtime is a library provided (currently) as part of the SPL Transpiler which can provide more robust implementation as well as an SPL-like authoring experience when writing PySpark code directly.
The runtime is optional and must be installed explicitly:
pip install spl_transpiler[runtime]
For example, the following code snippets are equivalent:
In SPL (which can be transpiled and run on Spark):
sourcetype="cisco"
| eval x=len(raw)
| stats max(x) AS longest BY source
In the SPL Runtime:
from pyspark.sql import functions as F
from spl_transpiler.runtime import commands, functions
df_1 = commands.search(None, sourcetype=F.lit("cisco"))
df_2 = commands.eval(df_1, x=functions.eval.len(F.col("raw")))
df_3 = commands.stats(
df_2, by=[F.col("source")], longest=functions.stats.max(F.col("x"))
)
df_3
In raw PySpark without the Runtime, this is much more verbose and is less obviously equivalent:
from pyspark.sql import functions as F
spark.table(...).where(
(F.col("sourcetype") == F.lit("cisco")),
).withColumn(
"x",
F.length(F.col("raw")),
).groupBy(
[
"source",
]
).agg(
F.max(F.col("x")).alias("longest"),
)
This runtime is a collection of helper functions on top of PySpark, and can be intermingled with other PySpark code. This means you can leverage an SPL-like experience where convenient while still using regular PySpark code where convenient.
In addition to these helper functions, the runtime also provides UDFs (user-defined functions) to provide data
processing functions that aren't natively available in Spark.
For example, in eval is_local=cidrmatch("10.0.0.0/8", ip)
, the cidrmatch
function has no direct equivalent in Spark.
The runtime provides a UDF that can be used as follows, either directly:
from pyspark.sql import functions as F
from spl_transpiler.runtime import udfs
df = df.withColumn("is_local", udfs.cidr_match("10.0.0.0/8", F.col("ip")))
Or via the runtime:
from pyspark.sql import functions as F
from spl_transpiler.runtime import commands, functions
df_1 = commands.eval(None, is_local=functions.eval.cidrmatch("10.0.0.0/8", F.col("ip")))
df_1
The transpiler, by default, will not assume the presence of the runtime. You need to explicitly allow the runtime to enable these features:
from spl_transpiler import convert_spl_to_pyspark
convert_spl_to_pyspark(
'''eval is_local=cidrmatch("10.0.0.0/8", ip)''',
allow_runtime=True
)
Why transpile SPL into Spark? Because a huge amount of domain knowledge is locked up in the Splunk ecosystem, but Splunk is not always the optimal place to store and analyze data. Transpiling existing queries can make it easier for analysts and analytics to migrate iteratively onto other platforms. SPL is also a very laser-focused language for certain analytics, and in most cases it's far more concise than other languages (PySpark or SQL) at log processing tasks. Therefore, it may be preferable to continue writing queries in SPL and use a transpiler layer to make that syntax viable on various platforms.
Why rewrite the Databricks Lab transpiler? A few reasons:
This project is in early development. While it parses most common SPL queries and can convert a non-trivial variety of queries to PySpark, it's extremely limited and not yet ready for any serious usage. However, it lays a solid foundation for the whole process and is modular enough to easily add incremental features to.
Ways to contribute:
A complete list of built-in Splunk commands is provided on the Splunk documentation page.
It is not the goal of this transpiler to support every feature of every SPL command. Splunk and Spark are two different platforms and not everything that is built in to Splunk makes sense to trivially convert to Spark. What's far more important is supporting most queries (say, 90% of all queries), which only requires supporting a reasonable number of the most commonly used commands.
For reference, however, here is a complete table of Splunk commands and the current and planned support status in this transpiler.
Support status can be one of the following:
None
: This command will result in a syntax error in the SPL parser, and is completely unrecognized.Parser
: This command can be parsed from SPL into a syntax tree, but cannot currently be rendered back as Pyspark
code.Partial
: This command can be parsed and rendered back to functional Pyspark code. Not all features may be supported.Complete
: This command can be parsed and rendered back to functional Pyspark code. All intended features are
supported. This library is still early in its development, and commands might get marked as Complete
while still
having unknown bugs or limitations.Commands that don't yet support the Runtime can still be converted to raw PySpark code. The only difference will be that the PySpark code will be verbose and native, rather than using the SPL-like interface the runtime provides.
Command | Support | Runtime | Target |
---|---|---|---|
High Priority | |||
bin (bucket ) | Partial | Yes | |
convert | Yes | Yes | |
dedup | Parser | Yes | |
eval | Partial | Yes | Yes |
eventstats | Partial | Yes | |
fields | Yes | Yes | |
fillnull | Partial | Yes | Yes |
geom | None | Yes | |
geomfilter | None | Yes | |
geostats | None | Yes | |
head | Partial | Yes | |
history | None | Yes | |
inputlookup | Parser | Yes | |
iplocation | None | Yes | |
join | Partial | Yes | |
lookup | Partial | Yes | |
metadata | None | Yes | |
mstats | None | Yes | |
multisearch | Partial | Yes | |
mvexpand | Parser | Yes | |
outputlookup | None | Yes | |
rare | Yes | Yes | |
regex | Yes | Yes | |
rename | Partial | Yes | |
rex | Partial | Yes | |
search | Partial | Yes | Yes |
sort | Partial | Yes | |
spath | Partial | Yes | |
stats | Partial | Yes | Yes |
streamstats | Parser | Yes | |
table | Partial | Yes | |
tail | Yes | Yes | |
top | Yes | Yes | |
tstats | Partial | Yes | Yes |
where | Partial | Yes | |
Planned/Supported | |||
addtotals | Partial | Yes | |
anomalydetection | None | Maybe | |
append | None | Maybe | |
appendpipe | None | Maybe | |
chart | None | Maybe | |
collect | Parser | Maybe | |
extract (kv ) | None | Maybe | |
foreach | None | Yes | |
format | Parser | Yes | |
from | None | Yes | |
makecontinuous | None | Maybe | |
makemv | None | Maybe | |
makeresults | Parser | Yes | |
map | Parser | Yes | |
multikv | None | Maybe | |
replace | None | Maybe | |
return | Parser | Yes | |
transaction | None | Yes | |
xmlkv | None | Yes | |
Unsupported | |||
abstract | None | ||
accum | None | ||
addcoltotals | None | ||
addinfo | None | ||
analyzefields | None | ||
anomalies | None | ||
anomalousvalue | None | ||
appendcols | None | ||
arules | None | ||
associate | None | ||
autoregress | None | ||
bucketdir | None | ||
cluster | None | ||
cofilter | None | ||
concurrency | None | ||
contingency | None | ||
correlate | None | ||
datamodel | Partial | Yes | |
dbinspect | None | ||
delete | None | ||
delta | None | ||
diff | None | ||
erex | None | ||
eventcount | None | ||
fieldformat | None | ||
fieldsummary | None | ||
filldown | None | ||
findtypes | None | ||
folderize | None | ||
gauge | None | ||
gentimes | None | ||
highlight | None | ||
iconify | None | ||
inputcsv | None | ||
kmeans | None | ||
kvform | None | ||
loadjob | None | ||
localize | None | ||
localop | None | ||
mcollect | None | ||
metasearch | None | ||
meventcollect | None | ||
mpreview | None | ||
msearch | None | ||
mvcombine | Parser | ||
nomv | None | ||
outlier | None | Maybe | |
outputcsv | None | ||
outputtext | None | ||
overlap | None | ||
pivot | None | ||
predict | None | ||
rangemap | None | ||
redistribute | None | ||
reltime | None | ||
require | None | ||
rest | None | ||
reverse | None | ||
rtorder | None | ||
savedsearch | None | ||
script (run ) | None | ||
scrub | None | ||
searchtxn | None | ||
selfjoin | None | ||
sendalert | None | ||
sendemail | None | ||
set | None | ||
setfields | None | ||
sichart | None | ||
sirare | None | ||
sistats | None | ||
sitimechart | None | ||
sitop | None | ||
strcat | None | ||
tags | None | ||
timechart | None | Maybe | |
timewrap | None | ||
tojson | None | ||
transpose | None | ||
trendline | None | ||
tscollect | None | ||
typeahead | None | ||
typelearner | None | ||
typer | None | ||
union | None | ||
uniq | None | ||
untable | None | ||
walklex | None | ||
x11 | None | ||
xmlunescape | None | ||
xpath | None | ||
xyseries | None |
There are two primary kinds of
functions: Evaluation functions (
primarily for use in eval
)
and Statistical and Charting functions (
primarily for use in stats
).
Like with commands, there are a lot of built-in functions and not all of them may map cleanly to Spark. This transpiler intends to support most queries and will thus support the most common functions. However, there is no goal at this time to support all Splunk functions.
If you are using the runtime, these functions are available as follows:
from spl_transpiler.runtime import functions
x = functions.eval.len(...)
y = functions.stats.max(...)
These functions are all wrappers and provide no new functionality.
In cases where the SPL function's behavior can be matched by a built-in function from the core Spark library, this wrapper simply converts an SPL-like syntax into the Spark equivalent.
In other cases where no Spark function exists (e.g. cidrmatch
), the wrapper calls a custom UDF from spl_transpiler.runtime.udfs
.
Even in these cases, the wrapper might still provide a more SPL-like syntax around the UDF, since the UDF is written and optimized for use apart from the wrapper (e.g. it might only provide part of the functionality of the overall SPL function).
Category | Subcategory | Function | Support | Runtime | Target |
---|---|---|---|---|---|
Eval | Bitwise | bit_and | Yes | Yes | Yes |
Eval | Bitwise | bit_or | Yes | Yes | Yes |
Eval | Bitwise | bit_not | Yes | Yes | Yes |
Eval | Bitwise | bit_xor | Yes | Yes | Yes |
Eval | Bitwise | bit_shift_left | Yes | Yes | Yes |
Eval | Bitwise | bit_shift_right | Yes | Yes | Yes |
Eval | Comparison and Conditional | case | Yes | Yes | Yes |
Eval | Comparison and Conditional | cidrmatch | Yes* | Yes (UDF) | Yes |
Eval | Comparison and Conditional | coalesce | Yes | Yes | Yes |
Eval | Comparison and Conditional | false | Yes | Yes | Yes |
Eval | Comparison and Conditional | if | Yes | Yes | Yes |
Eval | Comparison and Conditional | in | Yes | Yes | Yes |
Eval | Comparison and Conditional | like | Yes | Yes | Yes |
Eval | Comparison and Conditional | lookup | No | No | |
Eval | Comparison and Conditional | match | Yes | Yes | Yes |
Eval | Comparison and Conditional | null | Yes | Yes | Yes |
Eval | Comparison and Conditional | nullif | Yes | Yes | Yes |
Eval | Comparison and Conditional | searchmatch | No | No | |
Eval | Comparison and Conditional | true | Yes | Yes | Yes |
Eval | Comparison and Conditional | validate | Yes | Yes | Yes |
Eval | Conversion | ipmask | No | Yes (UDF) | |
Eval | Conversion | printf | No | Yes (UDF) | |
Eval | Conversion | tonumber | Partial | Yes (UDF) | Yes |
Eval | Conversion | tostring | Partial | Yes (UDF) | Yes |
Eval | Cryptographic | md5 | Yes | Yes | Yes |
Eval | Cryptographic | sha1 | Yes | Yes | Yes |
Eval | Cryptographic | sha256 | Yes | Yes | Yes |
Eval | Cryptographic | sha512 | Yes | Yes | Yes |
Eval | Date and Time | now | Yes | Yes | Yes |
Eval | Date and Time | relative_time | Yes | Yes | Yes |
Eval | Date and Time | strftime | Partial | Partial | Yes |
Eval | Date and Time | strptime | Partial | Partial | Yes |
Eval | Date and Time | time | Yes | Yes | Yes |
Eval | Informational | isbool | No | No | No |
Eval | Informational | isint | No | No | No |
Eval | Informational | isnotnull | Yes | Yes | Yes |
Eval | Informational | isnull | Yes | Yes | Yes |
Eval | Informational | isnum | No | No | No |
Eval | Informational | isstr | No | No | No |
Eval | Informational | typeof | No | No | No |
Eval | JSON | json_object | No | No | |
Eval | JSON | json_append | No | No | |
Eval | JSON | json_array | No | No | |
Eval | JSON | json_array_to_mv | No | No | |
Eval | JSON | json_extend | No | No | |
Eval | JSON | json_extract | No | No | |
Eval | JSON | json_extract_exact | No | No | |
Eval | JSON | json_keys | Yes | Yes | |
Eval | JSON | json_set | No | No | |
Eval | JSON | json_set_exact | No | No | |
Eval | JSON | json_valid | Yes | Yes | |
Eval | Mathematical | abs | Yes | Yes | Yes |
Eval | Mathematical | ceiling (ceil ) | Yes | Yes | Yes |
Eval | Mathematical | exact | Yes* | Yes* | No |
Eval | Mathematical | exp | Yes | Yes | Yes |
Eval | Mathematical | floor | Yes | Yes | Yes |
Eval | Mathematical | ln | Yes | Yes | Yes |
Eval | Mathematical | log | Yes | Yes | Yes |
Eval | Mathematical | pi | Yes | Yes | Yes |
Eval | Mathematical | pow | Yes | Yes | Yes |
Eval | Mathematical | round | Yes | Yes | Yes |
Eval | Mathematical | sigfig | No | Yes | No |
Eval | Mathematical | sqrt | Yes | Yes | Yes |
Eval | Mathematical | sum | Yes | Yes | Yes |
Eval | Multivalue | commands | No | No | |
Eval | Multivalue | mvappend | Yes | Yes | Yes |
Eval | Multivalue | mvcount | Yes | Yes | Yes |
Eval | Multivalue | mvdedup | No | No | |
Eval | Multivalue | mvfilter | No | No | Yes |
Eval | Multivalue | mvfind | No | No | |
Eval | Multivalue | mvindex | Yes | Yes | Yes |
Eval | Multivalue | mvjoin | No | No | Yes |
Eval | Multivalue | mvmap | No | No | |
Eval | Multivalue | mvrange | No | No | |
Eval | Multivalue | mvsort | No | No | |
Eval | Multivalue | mvzip | Yes | Yes | |
Eval | Multivalue | mv_to_json_array | No | No | |
Eval | Multivalue | split | Yes | Yes | Yes |
Eval | Statistical | avg | Yes | Yes | Yes |
Eval | Statistical | max | Yes | Yes | Yes |
Eval | Statistical | min | Yes | Yes | Yes |
Eval | Statistical | random | Yes | Yes | Yes |
Eval | Text | len | Yes | Yes | Yes |
Eval | Text | lower | Yes | Yes | Yes |
Eval | Text | ltrim | Yes | Yes | Yes |
Eval | Text | replace | Yes | Yes | Yes |
Eval | Text | rtrim | Yes | Yes | Yes |
Eval | Text | spath | No | No | |
Eval | Text | substr | Yes | Yes | Yes |
Eval | Text | trim | Yes | Yes | Yes |
Eval | Text | upper | Yes | Yes | Yes |
Eval | Text | urldecode | Yes | Yes | Yes |
Eval | Trigonometry and Hyperbolic | acos | Yes | Yes | Yes |
Eval | Trigonometry and Hyperbolic | acosh | Yes | Yes | Yes |
Eval | Trigonometry and Hyperbolic | asin | Yes | Yes | Yes |
Eval | Trigonometry and Hyperbolic | asinh | Yes | Yes | Yes |
Eval | Trigonometry and Hyperbolic | atan | Yes | Yes | Yes |
Eval | Trigonometry and Hyperbolic | atan2 | Yes | Yes | Yes |
Eval | Trigonometry and Hyperbolic | atanh | Yes | Yes | Yes |
Eval | Trigonometry and Hyperbolic | cos | Yes | Yes | Yes |
Eval | Trigonometry and Hyperbolic | cosh | Yes | Yes | Yes |
Eval | Trigonometry and Hyperbolic | hypot | Yes | Yes | Yes |
Eval | Trigonometry and Hyperbolic | sin | Yes | Yes | Yes |
Eval | Trigonometry and Hyperbolic | sinh | Yes | Yes | Yes |
Eval | Trigonometry and Hyperbolic | tan | Yes | Yes | Yes |
Eval | Trigonometry and Hyperbolic | tanh | Yes | Yes | Yes |
Stats | Aggregate | avg | Yes | Yes | Yes |
Stats | Aggregate | count | Yes | Yes | Yes |
Stats | Aggregate | distinct_count (dc ) | Yes | Yes | Yes |
Stats | Aggregate | estdc | Yes | Yes | |
Stats | Aggregate | estdc_error | No | No | |
Stats | Aggregate | exactperc | Yes | Yes | |
Stats | Aggregate | max | Yes | Yes | Yes |
Stats | Aggregate | mean | Yes | Yes | Yes |
Stats | Aggregate | median | Yes | Yes | Yes |
Stats | Aggregate | min | Yes | Yes | Yes |
Stats | Aggregate | mode | Yes | Yes | Yes |
Stats | Aggregate | percentile | Yes | Yes | Yes |
Stats | Aggregate | range | Yes | Yes | Yes |
Stats | Aggregate | stdev | Yes | Yes | Yes |
Stats | Aggregate | stdevp | Yes | Yes | Yes |
Stats | Aggregate | sum | Yes | Yes | Yes |
Stats | Aggregate | sumsq | Yes | Yes | Yes |
Stats | Aggregate | upperperc | Yes | Yes | Yes |
Stats | Aggregate | var | Yes | Yes | Yes |
Stats | Aggregate | varp | Yes | Yes | Yes |
Stats | Event order | first | Yes | Yes | |
Stats | Event order | last | Yes | Yes | |
Stats | Multivalue stats and chart | list | Yes | Yes | |
Stats | Multivalue stats and chart | values | Yes | Yes | Yes |
Stats | Time | earliest | Yes | Yes | Yes |
Stats | Time | earliest_time | Yes? | Yes? | |
Stats | Time | latest | Yes | Yes | Yes |
Stats | Time | latest_time | Yes? | Yes? | |
Stats | Time | per_day | No | No | |
Stats | Time | per_hour | No | No | |
Stats | Time | per_minute | No | No | |
Stats | Time | per_second | No | No | |
Stats | Time | rate | Yes? | Yes? | |
Stats | Time | rate_avg | No | Yes? | |
Stats | Time | rate_sum | No | Yes? |
* Pyspark output depends on custom UDFs instead of native Spark functions. Some of these may be provided by this package, some may be provided by Databricks Sirens.
.cache()
){}
and @
in field namesYou'll need cargo
(Rust) and python
installed.
I recommend using uv
for managing the Python environment,
dependencies, and tools needed for this project.
Note that PySpark is currently only compatible with Python 3.11 and older, 3.12 and 3.13 are not yet supported.
E.g., you can use uv venv --python 3.11
to create a .venv
virtual environment with the appropriate Python
interpreter.
spl_transpiler
is developed against Python 3.10 and likely requires at least that.
This project uses maturin
and pyo3
for the Rust <-> Python interfacing, you'll need to install
maturin
, e.g. using uvx maturin
commands which will auto-install the tool
on first use.
This project uses pre-commit
to automate linting and formatting.
E.g. you can use uvx pre-commit install
to install pre-commit and set up its git hooks.
You can then build and install spl_transpiler
and all dependencies. First, make sure you have your virtual environment
activated (uv
commands will detect the venv by default if you use that, else follow activation instructions for your
virtual environment tool), then run uv pip install -e .[cli,test,runtime]
.
You can test the core transpiler using cargo test
.
The Rust test suites include full end-to-end tests of query conversions, ensuring that the transpiler compiles and
converts a wide range of known inputs into expected outputs.
The Python-side tests can be run with pytest
and primarily ensure that the Rust <-> Python interface is behaving as
expected.
It also includes runtime tests, which validate that hand-written and transpiled runtime code does what is expected using
known input/output data pairs running in an ephemeral Spark cluster.
There is also a large-scale Python test that can be run using pytest tests/test_sample_files_parse.py
.
By default, this test is ignored because it is slow and currently does not pass.
It runs the transpiler on >1,800 sample SPL queries and ensure that the transpiler doesn't crash, generating detailed
logs and error summaries along the way.
This test is useful when expanding the transpiler to support new syntax, command, functions, etc. to see if the changes
cause more commands/queries to transpile successfully.
It's also useful for identifying what elements of SPL should be prioritized next to support more real-world use cases.
This project is deeply indebted to several other projects:
FAQs
Convert Splunk SPL queries into PySpark code
We found that spl-transpiler demonstrated a healthy version release cadence and project activity because the last version was released less than a year ago. It has 2 open source maintainers collaborating on the project.
Did you know?
Socket for GitHub automatically highlights issues in each pull request and monitors the health of all your open source dependencies. Discover the contents of your packages and block harmful activity before you install or update your dependencies.
Security News
Fluent Assertions is facing backlash after dropping the Apache license for a commercial model, leaving users blindsided and questioning contributor rights.
Research
Security News
Socket researchers uncover the risks of a malicious Python package targeting Discord developers.
Security News
The UK is proposing a bold ban on ransomware payments by public entities to disrupt cybercrime, protect critical services, and lead global cybersecurity efforts.