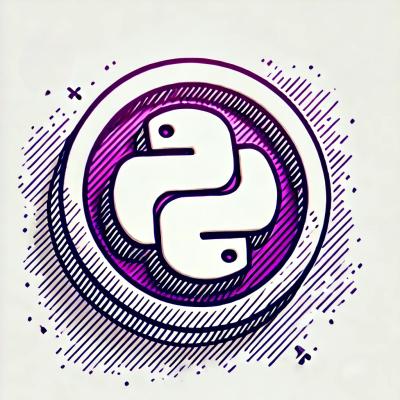
Security News
PyPI Now Supports iOS and Android Wheels for Mobile Python Development
PyPI now supports iOS and Android wheels, making it easier for Python developers to distribute mobile packages.
A fork of OpenAI Baselines, implementations of reinforcement learning algorithms.
WARNING: This package is in maintenance mode, please use Stable-Baselines3 (SB3) for an up-to-date version. You can find a migration guide in SB3 documentation.
Stable Baselines is a set of improved implementations of reinforcement learning algorithms based on OpenAI Baselines.
These algorithms will make it easier for the research community and industry to replicate, refine, and identify new ideas, and will create good baselines to build projects on top of. We expect these tools will be used as a base around which new ideas can be added, and as a tool for comparing a new approach against existing ones. We also hope that the simplicity of these tools will allow beginners to experiment with a more advanced toolset, without being buried in implementation details.
This toolset is a fork of OpenAI Baselines, with a major structural refactoring, and code cleanups:
Repository: https://github.com/hill-a/stable-baselines
Medium article: https://medium.com/@araffin/df87c4b2fc82
Documentation: https://stable-baselines.readthedocs.io/en/master/
RL Baselines Zoo: https://github.com/araffin/rl-baselines-zoo
Most of the library tries to follow a sklearn-like syntax for the Reinforcement Learning algorithms using Gym.
Here is a quick example of how to train and run PPO2 on a cartpole environment:
import gym
from stable_baselines.common.policies import MlpPolicy
from stable_baselines.common.vec_env import DummyVecEnv
from stable_baselines import PPO2
env = gym.make('CartPole-v1')
# Optional: PPO2 requires a vectorized environment to run
# the env is now wrapped automatically when passing it to the constructor
# env = DummyVecEnv([lambda: env])
model = PPO2(MlpPolicy, env, verbose=1)
model.learn(total_timesteps=10000)
obs = env.reset()
for i in range(1000):
action, _states = model.predict(obs)
obs, rewards, dones, info = env.step(action)
env.render()
Or just train a model with a one liner if the environment is registered in Gym and if the policy is registered:
from stable_baselines import PPO2
model = PPO2('MlpPolicy', 'CartPole-v1').learn(10000)
FAQs
A fork of OpenAI Baselines, implementations of reinforcement learning algorithms.
We found that stable-baselines demonstrated a healthy version release cadence and project activity because the last version was released less than a year ago. It has 4 open source maintainers collaborating on the project.
Did you know?
Socket for GitHub automatically highlights issues in each pull request and monitors the health of all your open source dependencies. Discover the contents of your packages and block harmful activity before you install or update your dependencies.
Security News
PyPI now supports iOS and Android wheels, making it easier for Python developers to distribute mobile packages.
Security News
Create React App is officially deprecated due to React 19 issues and lack of maintenance—developers should switch to Vite or other modern alternatives.
Security News
Oracle seeks to dismiss fraud claims in the JavaScript trademark dispute, delaying the case and avoiding questions about its right to the name.