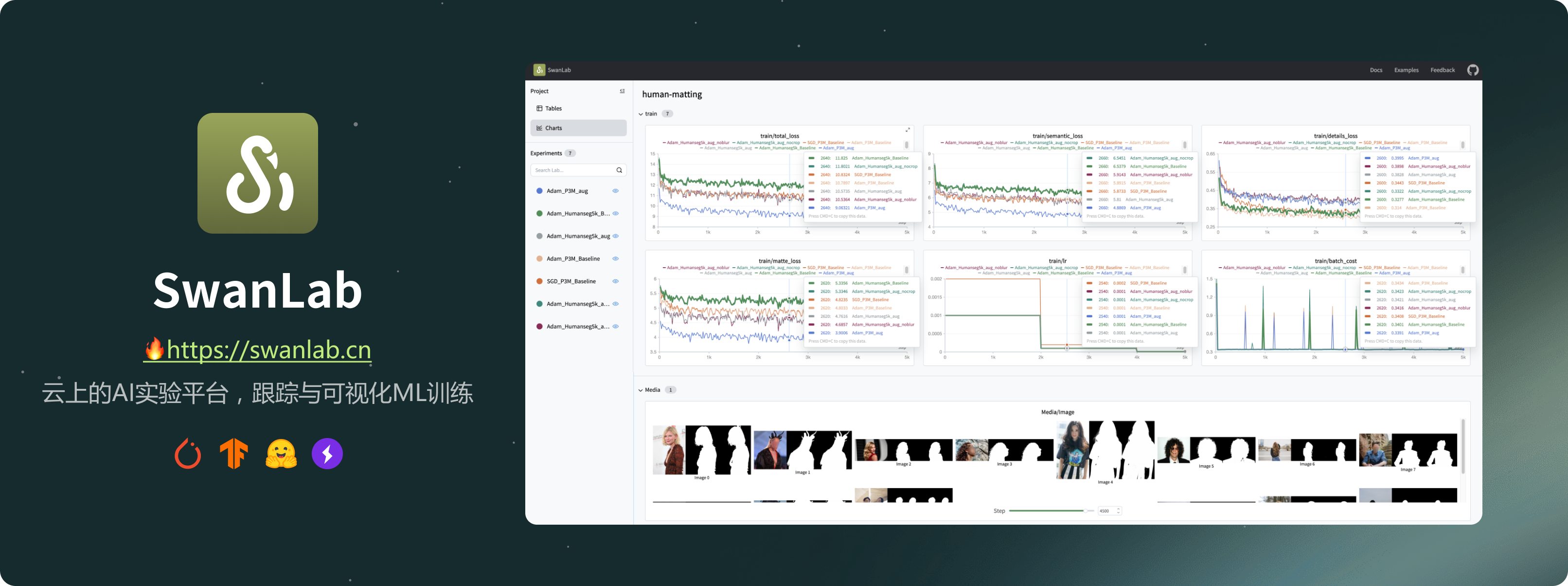
🔥SwanLab Cloud · 📃Document · WeChat · Report Issue · Feedback · Changelog
Table of contents
TOC
👋🏻 Introduction
SwanLab is an open-source, lightweight AI experiment tracking tool that provides a platform for tracking, comparing, and collaborating on experiments.
It offers a user-friendly API and a decent interface, combining features such as tracking hyperparameter, recording metric, online collaboration, and sharing experiment link.
Here is the English version of the core feature list for an AI platform:
1. 📊 Experimental Metrics and Tracking Hyperparameter: Embed your machine learning pipeline with minimalistic code
and track key training metrics.
- Flexible recording of hyperparameters and experiment configurations.
- Supported metadata types: scalar metrics, images, audio, text, etc.
- Supported chart types: line graphs, media charts (images, audio, text), etc.
- Automatic logging: console logging, GPU hardware, Git information, Python interpreter, list of Python libraries,
code directory.
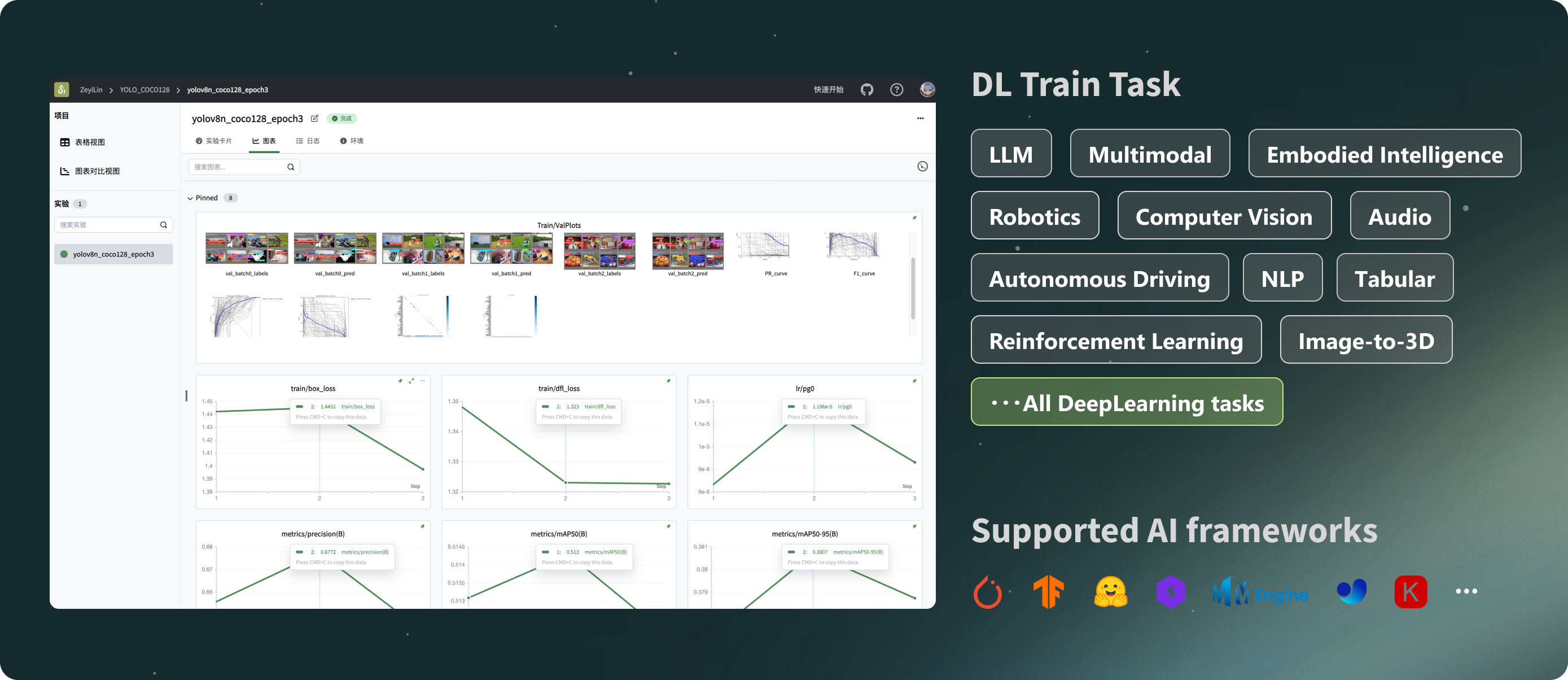
2. ⚡️ Comprehensive Framework Integration: PyTorch、Tensorflow、PyTorch Lightning、🤗HuggingFace、Transformers、MMEngine、Ultralytics、fastai、Tensorboard、OpenAI、ZhipuAI、Hydra、...
3. 📦 Organizing Experiments: Centralized dashboard for efficiently managing multiple projects and experiments,
providing an overview of training at a glance.
4. 🆚 Comparing Results: Use online tables and paired charts to compare the hyperparameters and outcomes of different
experiments, developing iterative inspiration.
5. 👥 Online Collaboration: Collaborate with your team on training projects, supporting real-time synchronization of
experiments under the same project, allowing you to synchronize training records of the team online and share insights
and suggestions based on results.
6. ✉️ Sharing Results: Copy and send persistent URLs to share each experiment, efficiently send them to colleagues,
or embed them in online notes.
7. 💻 Self-hosting Support: Supports offline mode with a self-hosted community version that also allows for dashboard
viewing and experiment management.
[!IMPORTANT]
Star Us, You will receive all release notifications from GitHub without any delay ~ ⭐️

📃 Demo
Check out SwanLab's online demo:
ResNet50 Cats vs Dogs | Yolov8-COCO128 |
---|
| |
Track the image classification task of training a simple ResNet50 model on the cats and dogs dataset. | Perform object detection tasks using Yolov8 on the COCO128 dataset, tracking training hyperparameters and metrics. |
More examples
🏁 Quick Start
1.Installation
pip install swanlab
2.Log in and get the API Key
-
Free Sign Up
-
Log in to your account, go to User Settings > API Key and copy your API Key.
-
Open your terminal and enter:
swanlab login
When prompted, enter your API Key and press Enter to complete the login.
3. Integrate SwanLab with Your Code
import swanlab
swanlab.init(
project="my-first-ml",
config={'learning-rate': 0.003}
)
for i in range(10):
swanlab.log({"loss": i})
All set! Visit SwanLab to see your first SwanLab experiment.
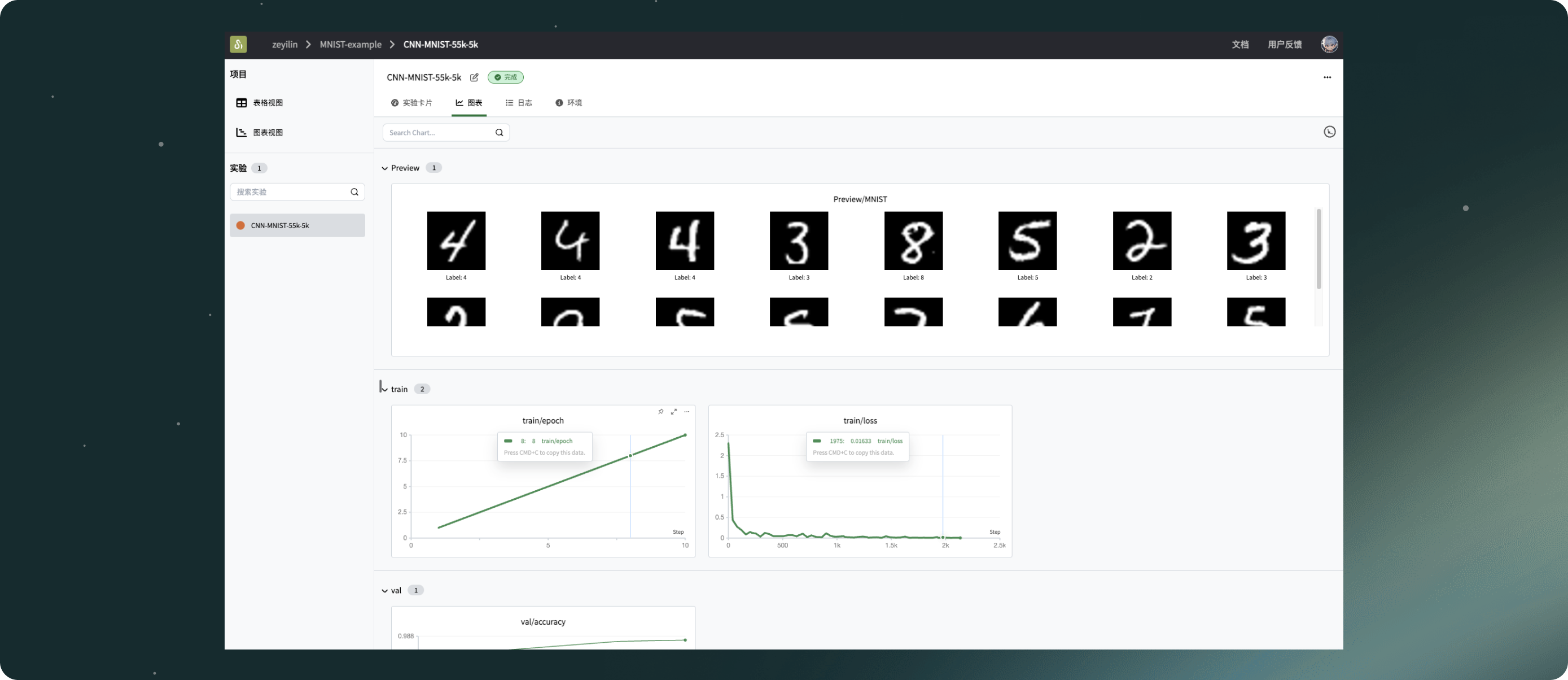
💻 Self-hosted
The community edition supports offline viewing of SwanLab dashboards.
Offline Experiment Tracking
Set the parameters logir
and mode
in swanlab.init to track experiments offline:
...
swanlab.init(
logdir='./logs',
mode='local',
)
...
-
The parameter mode
is set to local
, which disables synchronizing the experiment to the cloud.
-
The setting of the parameter logdir
is optional, and it specifies the location for saving SwanLab log files (by
default saved in the swanlog
folder).
-
Log files will be created and updated during tracking of experiments, and launching offline dashboards will also be
based on these log files.
Other parts are completely consistent with cloud usage.
Open Offline Board
Open the terminal and use the following command to open a SwanLab dashboard:
swanlab watch ./logs
After the operation is completed, SwanLab will provide you with a local URL link (default
is http://127.0.0.1:5092).
Visit this link to view the experiment offline in the browser dashboard.
🚗 Integration
Combine your favorite framework with
SwanLab, More Integration.
⚡️ PyTorch Lightning
Create an instance using SwanLabLogger
and pass it into the logger
parameter of Trainer
to enable SwanLab to
record training metrics.
from swanlab.integration.pytorch_lightning import SwanLabLogger
import importlib.util
import os
import pytorch_lightning as pl
from torch import nn, optim, utils
from torchvision.datasets import MNIST
from torchvision.transforms import ToTensor
encoder = nn.Sequential(nn.Linear(28 * 28, 128), nn.ReLU(), nn.Linear(128, 3))
decoder = nn.Sequential(nn.Linear(3, 128), nn.ReLU(), nn.Linear(128, 28 * 28))
class LitAutoEncoder(pl.LightningModule):
def __init__(self, encoder, decoder):
super().__init__()
self.encoder = encoder
self.decoder = decoder
def training_step(self, batch, batch_idx):
x, y = batch
x = x.view(x.size(0), -1)
z = self.encoder(x)
x_hat = self.decoder(z)
loss = nn.functional.mse_loss(x_hat, x)
self.log("train_loss", loss)
return loss
def test_step(self, batch, batch_idx):
x, y = batch
x = x.view(x.size(0), -1)
z = self.encoder(x)
x_hat = self.decoder(z)
loss = nn.functional.mse_loss(x_hat, x)
self.log("test_loss", loss)
return loss
def configure_optimizers(self):
optimizer = optim.Adam(self.parameters(), lr=1e-3)
return optimizer
autoencoder = LitAutoEncoder(encoder, decoder)
dataset = MNIST(os.getcwd(), train=True, download=True, transform=ToTensor())
train_dataset, val_dataset = utils.data.random_split(dataset, [55000, 5000])
test_dataset = MNIST(os.getcwd(), train=False, download=True, transform=ToTensor())
train_loader = utils.data.DataLoader(train_dataset)
val_loader = utils.data.DataLoader(val_dataset)
test_loader = utils.data.DataLoader(test_dataset)
swanlab_logger = SwanLabLogger(
project="swanlab_example",
experiment_name="example_experiment",
cloud=False,
)
trainer = pl.Trainer(limit_train_batches=100, max_epochs=5, logger=swanlab_logger)
trainer.fit(model=autoencoder, train_dataloaders=train_loader, val_dataloaders=val_loader)
trainer.test(dataloaders=test_loader)
🤗HuggingFace Transformers
Create an instance using SwanLabCallback
and pass it into the callbacks
parameter of Trainer
to enable SwanLab to
record training metrics.
import evaluate
import numpy as np
import swanlab
from swanlab.integration.huggingface import SwanLabCallback
from datasets import load_dataset
from transformers import AutoModelForSequenceClassification, AutoTokenizer, Trainer, TrainingArguments
def tokenize_function(examples):
return tokenizer(examples["text"], padding="max_length", truncation=True)
def compute_metrics(eval_pred):
logits, labels = eval_pred
predictions = np.argmax(logits, axis=-1)
return metric.compute(predictions=predictions, references=labels)
dataset = load_dataset("yelp_review_full")
tokenizer = AutoTokenizer.from_pretrained("bert-base-cased")
tokenized_datasets = dataset.map(tokenize_function, batched=True)
small_train_dataset = tokenized_datasets["train"].shuffle(seed=42).select(range(1000))
small_eval_dataset = tokenized_datasets["test"].shuffle(seed=42).select(range(1000))
metric = evaluate.load("accuracy")
model = AutoModelForSequenceClassification.from_pretrained("bert-base-cased", num_labels=5)
training_args = TrainingArguments(
output_dir="test_trainer",
report_to="none",
num_train_epochs=3,
logging_steps=50,
)
swanlab_callback = SwanLabCallback(experiment_name="TransformersTest", cloud=False)
trainer = Trainer(
model=model,
args=training_args,
train_dataset=small_train_dataset,
eval_dataset=small_eval_dataset,
compute_metrics=compute_metrics,
callbacks=[swanlab_callback],
)
trainer.train()
MMEngine(MMDetection etc.)
Integrate SwanlabVisBackend
into MMEngine to enable automatic logging of training metrics by SwanLab.
Add the following code snippet to your MM config file to start training:
custom_imports = dict(imports=["swanlab.integration.mmengine"], allow_failed_imports=False)
vis_backends = [
dict(
type="SwanlabVisBackend",
save_dir="runs/swanlab",
init_kwargs={
"project": "swanlab-mmengine",
},
),
]
visualizer = dict(
type="Visualizer",
vis_backends=vis_backends,
)
Ultralytics
Integrating SwanLab into Ultralytics is very simple; you can use the add_swanlab_callback
function:
from ultralytics import YOLO
from swanlab.integration.ultralytics import add_swanlab_callback
model = YOLO("yolov8n.yaml")
model.load()
add_swanlab_callback(model)
model.train(
data="./coco.yaml",
epochs=50,
imgsz=320,
)
🆚 Comparison with familiar tools
Tensorboard vs SwanLab
-
☁️ Online Usage Support:
With SwanLab, training experiments can be conveniently synchronized and saved in the cloud, allowing for remote
monitoring of training progress, managing historical projects, sharing experiment links, sending real-time
notification messages, and viewing experiments across multiple devices. In contrast, TensorBoard is an offline
experiment tracking tool.
-
👥 Collaborative Multi-user Environment:
SwanLab facilitates easy management of multi-person training projects and sharing of experiment links for
collaborative machine learning across teams. It also enables cross-space communication and discussion. On the other
hand, TensorBoard is primarily designed for individual use, making it difficult to collaborate and share experiments
with multiple users.
-
💻 Persistent, Centralized Dashboard:
Regardless of where you are training your models, be it on a local computer, a lab cluster, or on public cloud GPU
instances, your results are logged to the same centralized dashboard. Using TensorBoard, on the other hand, requires
spending time copying and managing TFEvent files from different machines.
-
💪 More Powerful Tables:
SwanLab tables allow you to view, search, and filter results from various experiments, making it easy to review
thousands of model versions to find the best-performing models for different tasks. TensorBoard is not well-suited for
large-scale projects.
Weights and Biases vs SwanLab
-
Weights and Biases is an online-only, proprietary MLOps platform.
-
Not only does SwanLab support online usage, but it also offers an open-source, free, and self-hosted version.
Community and support
- GitHub Issues:Errors and issues encountered when using SwanLab
- Email support:Feedback on issues with using SwanLab
- WeChat:Discuss issues using SwanLab,
share the latest AI technology.
SwanLab README Badge
If you like to use SwanLab in your work, please add the SwanLab badge to your README:

[](https://github.com/swanhubx/swanlab)
Citing SwanLab in the paper
If you find SwanLab helpful for your research journey, please consider citing in the following format:
@software{Zeyilin_SwanLab_2023,
author = {Zeyi Lin, Shaohong Chen, Kang Li, Qiushan Jiang, Zirui Cai, Kaifang Ji and {The SwanLab team}},
doi = {10.5281/zenodo.11100550},
license = {Apache-2.0},
title = {{SwanLab}},
url = {https://github.com/swanhubx/swanlab},
year = {2023}
}
Contribute to SwanLab
Considering contributing to SwanLab? First, please take some time to read
the Contribution Guidelines.
At the same time, we warmly welcome support for SwanLab through social media, events, and conference sharing. Thank you!
Contributors
Download Icon
SwanLab-Icon-SVG
📃 License
This repository follows the Apache 2.0 License open source
license.