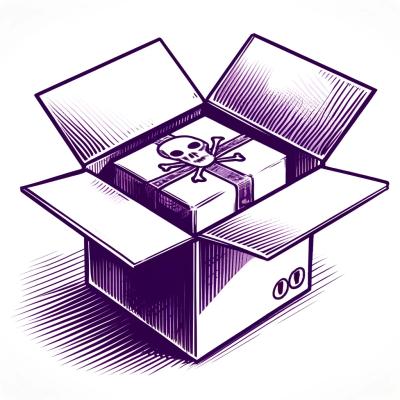
Security News
Research
Data Theft Repackaged: A Case Study in Malicious Wrapper Packages on npm
The Socket Research Team breaks down a malicious wrapper package that uses obfuscation to harvest credentials and exfiltrate sensitive data.
A package for Script Table Operator that applies set theory to machine learning in Python.
tdstone2
PackageThe tdstone2
package is designed to simplify the operationalization of Python code for data analysis and machine learning on the Teradata Vantage system. It leverages the massive parallel processing architecture of Teradata Vantage to run hundreds of Python scripts across hundreds of data partitions. This approach enables the industrialization, lineage, and reproducibility of millions of custom models while minimizing data movement.
To install tdstone2
, use pip:
pip install tdstone2
Ensure you have access to a Teradata Vantage system and the necessary credentials to connect and execute queries.
from sklearn.preprocessing import StandardScaler
from sklearn.ensemble import RandomForestClassifier
# Example usage
steps_classifier = [
('scaler', StandardScaler()),
('classifier', RandomForestClassifier(max_depth=5, n_estimators=95))
]
from tdstone2.tdshypermodel import HyperModel
from tdstone2.tdstone import TDStone
sto = TDStone(schema_name=Param['database'], SEARCHUIFDBPATH=Param['user'])
model_parameters = {
"target": 'Y2',
"column_categorical": ['flag', 'Y2'],
"column_names_X": ['X1', 'X2', 'X3', 'X4', 'X5', 'X6', 'X7', 'X8', 'X9', 'flag']
}
model = HyperModel(
tdstone=sto,
metadata={'project': 'test'},
skl_pipeline_steps=steps_classifier,
model_parameters=model_parameters,
dataset=tdml.in_schema(Param['database'], 'dataset_00'),
id_row='ID',
id_partition='Partition_ID',
id_fold='FOLD',
fold_training='train',
convert_to_onnx=False, # <-- set to True if you want to get the ONNX version of your trained models
store_pickle=True, # <-- to store your full object in pickle format
)
# Model deployment outputs
exec(code_and_data['code'])
local_model = MyModel(**code_and_data['arguments'])
df_local = code_and_data['data']
df_local['flag'] = df_local['flag'].astype('category')
df_local['Y2'] = df_local['Y2'].astype('category')
local_model.fit(code_and_data['data'])
local_model.score(code_and_data['data'])
model.train()
# Outputs: Trained models are inserted into the specified repository
This training operation launches as many training there are data partitions identified by the id_partition column list, and belonging to the training FOLD.
model.score()
# Outputs: Model scores are inserted into the specified scores table
This runs the scoring on all the data, using the latest model available for the corresponding data partition.
sto = TDStone(schema_name=Param['database'], SEARCHUIFDBPATH=Param['user'])
sto.list_hyper_models()
# Outputs: List of hyper models with their metadata
id = '0286d259-ecde-4cd0-ae4a-bcb3191383d1' # Example model ID
existing_model = HyperModel(tdstone=sto)
existing_model.download(id=id)
Note that the model is not actually downloaded, but this just establish a connection between the model hosted in Vantage and the python client.
existing_model.train()
# Outputs: Updated trained models are inserted into the specified repository
existing_model.score()
# Outputs: Updated scores are inserted into the specified scores table
FAQs
A package for Script Table Operator that applies set theory to machine learning in Python.
We found that tdstone2 demonstrated a healthy version release cadence and project activity because the last version was released less than a year ago. It has 1 open source maintainer collaborating on the project.
Did you know?
Socket for GitHub automatically highlights issues in each pull request and monitors the health of all your open source dependencies. Discover the contents of your packages and block harmful activity before you install or update your dependencies.
Security News
Research
The Socket Research Team breaks down a malicious wrapper package that uses obfuscation to harvest credentials and exfiltrate sensitive data.
Research
Security News
Attackers used a malicious npm package typosquatting a popular ESLint plugin to steal sensitive data, execute commands, and exploit developer systems.
Security News
The Ultralytics' PyPI Package was compromised four times in one weekend through GitHub Actions cache poisoning and failure to rotate previously compromised API tokens.