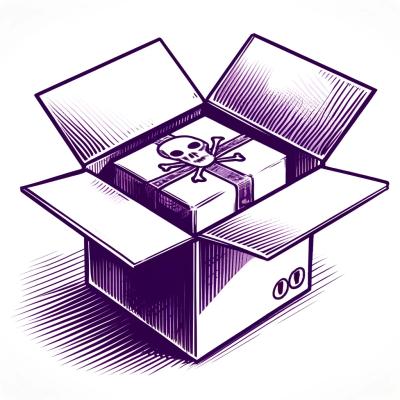
Security News
Research
Data Theft Repackaged: A Case Study in Malicious Wrapper Packages on npm
The Socket Research Team breaks down a malicious wrapper package that uses obfuscation to harvest credentials and exfiltrate sensitive data.
tensorQTL is a GPU-enabled QTL mapper, achieving ~200-300 fold faster cis- and trans-QTL mapping compared to CPU-based implementations.
If you use tensorQTL in your research, please cite the following paper: Taylor-Weiner, Aguet, et al., Genome Biol., 2019.
Empirical beta-approximated p-values are computed as described in Ongen et al., Bioinformatics, 2016.
You can install tensorQTL using pip:
pip3 install tensorqtl
or directly from this repository:
$ git clone git@github.com:broadinstitute/tensorqtl.git
$ cd tensorqtl
# set up virtual environment and install
$ virtualenv venv
$ source venv/bin/activate
(venv)$ pip install -r install/requirements.txt .
To use PLINK 2 binary files (pgen/pvar/psam), pgenlib must be installed:
git clone git@github.com:chrchang/plink-ng.git
cd plink-ng/2.0/Python/
python3 setup.py build_ext
python3 setup.py install
tensorQTL requires an environment configured with a GPU for optimal performance, but can also be run on a CPU. Instructions for setting up a virtual machine on Google Cloud Platform are provided here.
Three inputs are required for QTL analyses with tensorQTL: genotypes, phenotypes, and covariates.
Phenotypes must be provided in BED format, with a single header line starting with #
and the first four columns corresponding to: chr
, start
, end
, phenotype_id
, with the remaining columns corresponding to samples (the identifiers must match those in the genotype input). The BED file should specify the center of the cis-window (usually the TSS), with start == end-1
. A function for generating a BED template from a gene annotation in GTF format is available in pyqtl (io.gtf_to_tss_bed
).
Covariates can be provided as a tab-delimited text file (covariates x samples) or dataframe (samples x covariates), with row and column headers.
Genotypes must be in PLINK format, which can be generated from a VCF as follows:
plink2 --make-bed \
--output-chr chrM \
--vcf ${plink_prefix_path}.vcf.gz \
--out ${plink_prefix_path}
If using PLINK 1.9 or earlier, add the --keep-allele-order
flag.
Alternatively, the genotypes can be provided as a dataframe (genotypes x samples).
The examples notebook below contains examples of all input files. The input formats for phenotypes and covariates are identical to those used by FastQTL.
For examples illustrating cis- and trans-QTL mapping, please see tensorqtl_examples.ipynb.
This section describes how to run the different modes of tensorQTL, both from the command line and within Python. For a full list of options, run
python3 -m tensorqtl --help
This section is only relevant when running tensorQTL in Python. The following imports are required:
import pandas as pd
import tensorqtl
from tensorqtl import genotypeio, cis, trans
Phenotypes and covariates can be loaded as follows:
phenotype_df, phenotype_pos_df = tensorqtl.read_phenotype_bed(phenotype_bed_file)
covariates_df = pd.read_csv(covariates_file, sep='\t', index_col=0).T # samples x covariates
Genotypes can be loaded as follows, where plink_prefix_path
is the path to the VCF in PLINK format (excluding .bed
/.bim
/.fam
extensions):
pr = genotypeio.PlinkReader(plink_prefix_path)
# load genotypes and variants into data frames
genotype_df = pr.load_genotypes()
variant_df = pr.bim.set_index('snp')[['chrom', 'pos']]
To save memory when using genotypes for a subset of samples, a subset of samples can be loaded (this is not strictly necessary, since tensorQTL will select the relevant samples from genotype_df
otherwise):
pr = genotypeio.PlinkReader(plink_prefix_path, select_samples=phenotype_df.columns)
This is the main mode for cis-QTL mapping. It generates phenotype-level summary statistics with empirical p-values, enabling calculation of genome-wide FDR. In Python:
cis_df = cis.map_cis(genotype_df, variant_df, phenotype_df, phenotype_pos_df, covariates_df)
tensorqtl.calculate_qvalues(cis_df, qvalue_lambda=0.85)
Shell command:
python3 -m tensorqtl ${plink_prefix_path} ${expression_bed} ${prefix} \
--covariates ${covariates_file} \
--mode cis
${prefix}
specifies the output file name.
In Python:
cis.map_nominal(genotype_df, variant_df, phenotype_df, phenotype_pos_df,
prefix, covariates_df, output_dir='.')
Shell command:
python3 -m tensorqtl ${plink_prefix_path} ${expression_bed} ${prefix} \
--covariates ${covariates_file} \
--mode cis_nominal
The results are written to a parquet file for each chromosome. These files can be read using pandas
:
df = pd.read_parquet(file_name)
This mode maps conditionally independent cis-QTLs using the stepwise regression procedure described in GTEx Consortium, 2017. The output from the permutation step (see map_cis
above) is required.
In Python:
indep_df = cis.map_independent(genotype_df, variant_df, cis_df,
phenotype_df, phenotype_pos_df, covariates_df)
Shell command:
python3 -m tensorqtl ${plink_prefix_path} ${expression_bed} ${prefix} \
--covariates ${covariates_file} \
--cis_output ${prefix}.cis_qtl.txt.gz \
--mode cis_independent
Instead of mapping the standard linear model (p ~ g), this mode includes an interaction term (p ~ g + i + gi) and returns full summary statistics for the model. The interaction term is a tab-delimited text file or dataframe mapping sample ID to interaction value(s) (if multiple interactions are used, the file must include a header with variable names). With the run_eigenmt=True
option, eigenMT-adjusted p-values are computed.
In Python:
cis.map_nominal(genotype_df, variant_df, phenotype_df, phenotype_pos_df, prefix,
covariates_df=covariates_df,
interaction_df=interaction_df, maf_threshold_interaction=0.05,
run_eigenmt=True, output_dir='.', write_top=True, write_stats=True)
The input options write_top
and write_stats
control whether the top association per phenotype and full summary statistics, respectively, are written to file.
Shell command:
python3 -m tensorqtl ${plink_prefix_path} ${expression_bed} ${prefix} \
--covariates ${covariates_file} \
--interaction ${interactions_file} \
--best_only \
--mode cis_nominal
The option --best_only
disables output of full summary statistics.
Full summary statistics are saved as parquet files for each chromosome, in ${output_dir}/${prefix}.cis_qtl_pairs.${chr}.parquet
, and the top association for each phenotype is saved to ${output_dir}/${prefix}.cis_qtl_top_assoc.txt.gz
. In these files, the columns b_g
, b_g_se
, pval_g
are the effect size, standard error, and p-value of g in the model, with matching columns for i and gi. In the *.cis_qtl_top_assoc.txt.gz
file, tests_emt
is the effective number of independent variants in the cis-window estimated with eigenMT, i.e., based on the eigenvalue decomposition of the regularized genotype correlation matrix (Davis et al., AJHG, 2016). pval_emt = pval_gi * tests_emt
, and pval_adj_bh
are the Benjamini-Hochberg adjusted p-values corresponding to pval_emt
.
This mode computes nominal associations between all phenotypes and genotypes. tensorQTL generates sparse output by default (associations with p-value < 1e-5). cis-associations are filtered out. The output is in parquet format, with four columns: phenotype_id, variant_id, pval, maf. In Python:
trans_df = trans.map_trans(genotype_df, phenotype_df, covariates_df,
return_sparse=True, pval_threshold=1e-5, maf_threshold=0.05,
batch_size=20000)
# remove cis-associations
trans_df = trans.filter_cis(trans_df, phenotype_pos_df.T.to_dict(), variant_df, window=5000000)
Shell command:
python3 -m tensorqtl ${plink_prefix_path} ${expression_bed} ${prefix} \
--covariates ${covariates_file} \
--mode trans
FAQs
GPU-accelerated QTL mapper
We found that tensorqtl demonstrated a healthy version release cadence and project activity because the last version was released less than a year ago. It has 1 open source maintainer collaborating on the project.
Did you know?
Socket for GitHub automatically highlights issues in each pull request and monitors the health of all your open source dependencies. Discover the contents of your packages and block harmful activity before you install or update your dependencies.
Security News
Research
The Socket Research Team breaks down a malicious wrapper package that uses obfuscation to harvest credentials and exfiltrate sensitive data.
Research
Security News
Attackers used a malicious npm package typosquatting a popular ESLint plugin to steal sensitive data, execute commands, and exploit developer systems.
Security News
The Ultralytics' PyPI Package was compromised four times in one weekend through GitHub Actions cache poisoning and failure to rotate previously compromised API tokens.