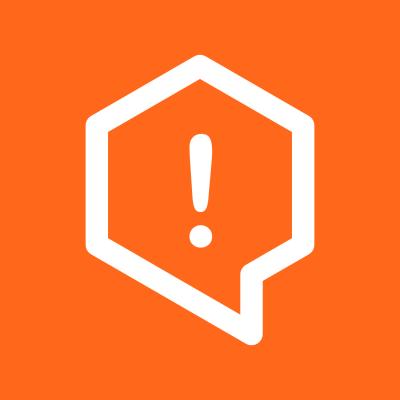
Security News
Fluent Assertions Faces Backlash After Abandoning Open Source Licensing
Fluent Assertions is facing backlash after dropping the Apache license for a commercial model, leaving users blindsided and questioning contributor rights.
Use TiDB Vector Search with Python.
TiDB is a SQL database so that this package introduces Vector Search capability for Python ORMs:
Pick one that you are familiar with to get started. If you are not using any of them, we recommend #SQLAlchemy.
We also provide a Vector Search client for simple usage:
Install:
pip install tidb-vector sqlalchemy pymysql
Usage:
from sqlalchemy import Integer, Column
from sqlalchemy import create_engine, select
from sqlalchemy.dialects.mysql import LONGTEXT
from sqlalchemy.orm import Session, declarative_base
import tidb_vector
from tidb_vector.sqlalchemy import VectorType, VectorAdaptor
engine = create_engine("mysql+pymysql://root@127.0.0.1:4000/test")
Base = declarative_base()
# Define table schema
class Doc(Base):
__tablename__ = "doc"
id = Column(Integer, primary_key=True)
embedding = Column(VectorType(dim=3))
content = Column(LONGTEXT)
# Create empty table
Base.metadata.drop_all(engine) # clean data from last run
Base.metadata.create_all(engine)
# Create index for L2 distance
VectorAdaptor(engine).create_vector_index(
Doc.embedding, tidb_vector.DistanceMetric.L2, skip_existing=True
# For cosine distance, use tidb_vector.DistanceMetric.COSINE
)
# Insert content with vectors
with Session(engine) as session:
session.add(Doc(id=1, content="dog", embedding=[1, 2, 1]))
session.add(Doc(id=2, content="fish", embedding=[1, 2, 4]))
session.add(Doc(id=3, content="tree", embedding=[1, 0, 0]))
session.commit()
# Perform Vector Search for Top K=1
with Session(engine) as session:
results = session.execute(
select(Doc.id, Doc.content)
.order_by(Doc.embedding.l2_distance([1, 2, 3]))
# For cosine distance, use Doc.embedding.cosine_distance(...)
.limit(1)
).all()
print(results)
# Perform filtered Vector Search by adding a Where Clause:
with Session(engine) as session:
results = session.execute(
select(Doc.id, Doc.content)
.where(Doc.content == "dog")
.order_by(Doc.embedding.l2_distance([1, 2, 3]))
.limit(1)
).all()
print(results)
Install:
pip install tidb-vector peewee pymysql
Usage:
import tidb_vector
from peewee import Model, MySQLDatabase, IntegerField, TextField
from tidb_vector.peewee import VectorField, VectorAdaptor
db = MySQLDatabase(
database="test",
user="root",
password="",
host="127.0.0.1",
port=4000,
)
# Define table schema
class Doc(Model):
class Meta:
database = db
table_name = "peewee_test"
id = IntegerField(primary_key=True)
embedding = VectorField(3)
content = TextField()
# Create empty table and index for L2 distance
db.drop_tables([Doc]) # clean data from last run
db.create_tables([Doc])
# For cosine distance, use tidb_vector.DistanceMetric.COSINE
VectorAdaptor(db).create_vector_index(Doc.embedding, tidb_vector.DistanceMetric.L2)
# Insert content with vectors
Doc.insert_many(
[
{"id": 1, "content": "dog", "embedding": [1, 2, 1]},
{"id": 2, "content": "fish", "embedding": [1, 2, 4]},
{"id": 3, "content": "tree", "embedding": [1, 0, 0]},
]
).execute()
# Perform Vector Search for Top K=1
cursor = (
Doc.select(Doc.id, Doc.content)
# For cosine distance, use Doc.embedding.cosine_distance(...)
.order_by(Doc.embedding.l2_distance([1, 2, 3]))
.limit(1)
)
for row in cursor:
print(row.id, row.content)
# Perform filtered Vector Search by adding a Where Clause:
cursor = (
Doc.select(Doc.id, Doc.content)
.where(Doc.content == "dog")
.order_by(Doc.embedding.l2_distance([1, 2, 3]))
.limit(1)
)
for row in cursor:
print(row.id, row.content)
[!TIP]
Django is a full-featured web framework, not just an ORM. The following usage introducutions are provided for existing Django users.
For new users to get started, consider using SQLAlchemy or Peewee.
Install:
pip install 'django-tidb[vector]~=5.0.0' 'django~=5.0.0' mysqlclient
Usage:
1. Configure django_tidb
as engine, like:
DATABASES = {
'default': {
'ENGINE': 'django_tidb',
'NAME': 'django',
'USER': 'root',
'PASSWORD': '',
'HOST': '127.0.0.1',
'PORT': 4000,
},
}
2. Define a model with a vector field and vector index:
from django.db import models
from django_tidb.fields.vector import VectorField, VectorIndex, L2Distance
class Doc(models.Model):
id = models.IntegerField(primary_key=True)
embedding = VectorField(dimensions=3)
content = models.TextField()
class Meta:
indexes = [VectorIndex(L2Distance("embedding"), name="idx")]
3. Insert data:
Doc.objects.create(id=1, content="dog", embedding=[1, 2, 1])
Doc.objects.create(id=2, content="fish", embedding=[1, 2, 4])
Doc.objects.create(id=3, content="tree", embedding=[1, 0, 0])
4. Perform Vector Search for Top K=1:
queryset = (
Doc.objects
.order_by(L2Distance("embedding", [1, 2, 3]))
.values("id", "content")[:1]
)
print(queryset)
5. Perform filtered Vector Search by adding a Where Clause:
queryset = (
Doc.objects
.filter(content="dog")
.order_by(L2Distance("embedding", [1, 2, 3]))
.values("id", "content")[:1]
)
print(queryset)
For more details, see django-tidb.
Within the framework, you can directly utilize the built-in TiDBVectorClient
, as demonstrated by integrations like Langchain and Llama index, to seamlessly interact with TiDB Vector. This approach abstracts away the need to manage the underlying ORM, simplifying your interaction with the vector store.
We provide TiDBVectorClient
which is based on sqlalchemy, you need to use pip install tidb-vector[client]
to install it.
Create a TiDBVectorClient
instance:
from tidb_vector.integrations import TiDBVectorClient
TABLE_NAME = 'vector_test'
CONNECTION_STRING = 'mysql+pymysql://<USER>:<PASSWORD>@<HOST>:4000/<DB>?ssl_verify_cert=true&ssl_verify_identity=true'
tidb_vs = TiDBVectorClient(
# the table which will store the vector data
table_name=TABLE_NAME,
# tidb connection string
connection_string=CONNECTION_STRING,
# the dimension of the vector, in this example, we use the ada model, which has 1536 dimensions
vector_dimension=1536,
# if recreate the table if it already exists
drop_existing_table=True,
)
Bulk insert:
ids = [
"f8e7dee2-63b6-42f1-8b60-2d46710c1971",
"8dde1fbc-2522-4ca2-aedf-5dcb2966d1c6",
"e4991349-d00b-485c-a481-f61695f2b5ae",
]
documents = ["foo", "bar", "baz"]
embeddings = [
text_to_embedding("foo"),
text_to_embedding("bar"),
text_to_embedding("baz"),
]
metadatas = [
{"page": 1, "category": "P1"},
{"page": 2, "category": "P1"},
{"page": 3, "category": "P2"},
]
tidb_vs.insert(
ids=ids,
texts=documents,
embeddings=embeddings,
metadatas=metadatas,
)
Query:
tidb_vs.query(text_to_embedding("foo"), k=3)
# query with filter
tidb_vs.query(text_to_embedding("foo"), k=3, filter={"category": "P1"})
Bulk delete:
tidb_vs.delete(["f8e7dee2-63b6-42f1-8b60-2d46710c1971"])
# delete with filter
tidb_vs.delete(["f8e7dee2-63b6-42f1-8b60-2d46710c1971"], filter={"category": "P1"})
There are some examples to show how to use the tidb-vector-python to interact with TiDB Vector in different scenarios.
for more examples, see the examples directory.
Please feel free to reach out to the maintainers if you have any questions or need help with the project. Before contributing, please read the CONTRIBUTING.md file.
FAQs
A Python client for TiDB Vector
We found that tidb-vector demonstrated a healthy version release cadence and project activity because the last version was released less than a year ago. It has 2 open source maintainers collaborating on the project.
Did you know?
Socket for GitHub automatically highlights issues in each pull request and monitors the health of all your open source dependencies. Discover the contents of your packages and block harmful activity before you install or update your dependencies.
Security News
Fluent Assertions is facing backlash after dropping the Apache license for a commercial model, leaving users blindsided and questioning contributor rights.
Research
Security News
Socket researchers uncover the risks of a malicious Python package targeting Discord developers.
Security News
The UK is proposing a bold ban on ransomware payments by public entities to disrupt cybercrime, protect critical services, and lead global cybersecurity efforts.