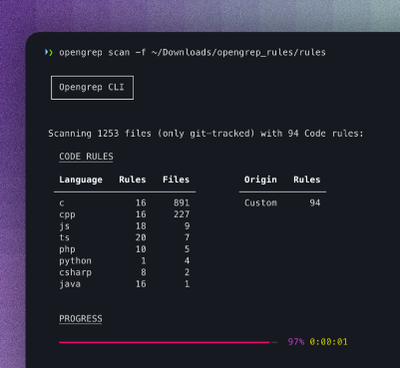
Security News
Opengrep Emerges as Open Source Alternative Amid Semgrep Licensing Controversy
Opengrep forks Semgrep to preserve open source SAST in response to controversial licensing changes.
Tifffile is a Python library to
(1) store NumPy arrays in TIFF (Tagged Image File Format) files, and (2) read image and metadata from TIFF-like files used in bioimaging.
Image and metadata can be read from TIFF, BigTIFF, OME-TIFF, GeoTIFF, Adobe DNG, ZIF (Zoomable Image File Format), MetaMorph STK, Zeiss LSM, ImageJ hyperstack, Micro-Manager MMStack and NDTiff, SGI, NIHImage, Olympus FluoView and SIS, ScanImage, Molecular Dynamics GEL, Aperio SVS, Leica SCN, Roche BIF, PerkinElmer QPTIFF (QPI, PKI), Hamamatsu NDPI, Argos AVS, and Philips DP formatted files.
Image data can be read as NumPy arrays or Zarr 2 arrays/groups from strips, tiles, pages (IFDs), SubIFDs, higher order series, and pyramidal levels.
Image data can be written to TIFF, BigTIFF, OME-TIFF, and ImageJ hyperstack compatible files in multi-page, volumetric, pyramidal, memory-mappable, tiled, predicted, or compressed form.
Many compression and predictor schemes are supported via the imagecodecs library, including LZW, PackBits, Deflate, PIXTIFF, LZMA, LERC, Zstd, JPEG (8 and 12-bit, lossless), JPEG 2000, JPEG XR, JPEG XL, WebP, PNG, EER, Jetraw, 24-bit floating-point, and horizontal differencing.
Tifffile can also be used to inspect TIFF structures, read image data from multi-dimensional file sequences, write fsspec ReferenceFileSystem for TIFF files and image file sequences, patch TIFF tag values, and parse many proprietary metadata formats.
:Author: Christoph Gohlke <https://www.cgohlke.com>
_
:License: BSD 3-Clause
:Version: 2025.1.10
:DOI: 10.5281/zenodo.6795860 <https://doi.org/10.5281/zenodo.6795860>
_
Install the tifffile package and all dependencies from the
Python Package Index <https://pypi.org/project/tifffile/>
_::
python -m pip install -U tifffile[all]
Tifffile is also available in other package repositories such as Anaconda, Debian, and MSYS2.
The tifffile library is type annotated and documented via docstrings::
python -c "import tifffile; help(tifffile)"
Tifffile can be used as a console script to inspect and preview TIFF files::
python -m tifffile --help
See Examples
_ for using the programming interface.
Source code and support are available on
GitHub <https://github.com/cgohlke/tifffile>
_.
Support is also provided on the
image.sc <https://forum.image.sc/tag/tifffile>
_ forum.
This revision was tested with the following requirements and dependencies (other versions may work):
CPython <https://www.python.org>
_ 3.10.11, 3.11.9, 3.12.8, 3.13.1 64-bitNumPy <https://pypi.org/project/numpy/>
_ 2.2.1Imagecodecs <https://pypi.org/project/imagecodecs/>
_ 2024.12.30
(required for encoding or decoding LZW, JPEG, etc. compressed segments)Matplotlib <https://pypi.org/project/matplotlib/>
_ 3.10.0
(required for plotting)Lxml <https://pypi.org/project/lxml/>
_ 5.3.0
(required only for validating and printing XML)Zarr <https://pypi.org/project/zarr/>
_ 2.18.4
(required only for opening Zarr stores; Zarr 3 is not compatible)Fsspec <https://pypi.org/project/fsspec/>
_ 2024.12.0
(required only for opening ReferenceFileSystem files)2025.1.10
2024.12.12
2024.9.20
2024.8.30
2024.8.28
2024.8.24
2024.8.10
2024.7.24
2024.7.21
2024.7.2
2024.6.18
2024.5.22
2024.5.10
2024.5.3
2024.4.24
2024.4.18
2024.2.12
2024.1.30
2023.12.9
Refer to the CHANGES file for older revisions.
TIFF, the Tagged Image File Format, was created by the Aldus Corporation and Adobe Systems Incorporated.
Tifffile supports a subset of the TIFF6 specification, mainly 8, 16, 32, and 64-bit integer, 16, 32 and 64-bit float, grayscale and multi-sample images. Specifically, CCITT and OJPEG compression, chroma subsampling without JPEG compression, color space transformations, samples with differing types, or IPTC, ICC, and XMP metadata are not implemented.
Besides classic TIFF, tifffile supports several TIFF-like formats that do not strictly adhere to the TIFF6 specification. Some formats allow file and data sizes to exceed the 4 GB limit of the classic TIFF:
Other libraries for reading, writing, inspecting, or manipulating scientific
TIFF files from Python are
aicsimageio <https://pypi.org/project/aicsimageio>
,
apeer-ometiff-library <https://github.com/apeer-micro/apeer-ometiff-library>
,
bigtiff <https://pypi.org/project/bigtiff>
,
fabio.TiffIO <https://github.com/silx-kit/fabio>
,
GDAL <https://github.com/OSGeo/gdal/>
,
imread <https://github.com/luispedro/imread>
,
large_image <https://github.com/girder/large_image>
,
openslide-python <https://github.com/openslide/openslide-python>
,
opentile <https://github.com/imi-bigpicture/opentile>
,
pylibtiff <https://github.com/pearu/pylibtiff>
,
pylsm <https://launchpad.net/pylsm>
,
pymimage <https://github.com/ardoi/pymimage>
,
python-bioformats <https://github.com/CellProfiler/python-bioformats>
,
pytiff <https://github.com/FZJ-INM1-BDA/pytiff>
,
scanimagetiffreader-python <https://gitlab.com/vidriotech/scanimagetiffreader-python>
,
SimpleITK <https://github.com/SimpleITK/SimpleITK>
,
slideio <https://gitlab.com/bioslide/slideio>
,
tiffslide <https://github.com/bayer-science-for-a-better-life/tiffslide>
,
tifftools <https://github.com/DigitalSlideArchive/tifftools>
,
tyf <https://github.com/Moustikitos/tyf>
,
xtiff <https://github.com/BodenmillerGroup/xtiff>
, and
ndtiff <https://github.com/micro-manager/NDTiffStorage>
.
Write a NumPy array to a single-page RGB TIFF file:
data = numpy.random.randint(0, 255, (256, 256, 3), 'uint8') imwrite('temp.tif', data, photometric='rgb')
Read the image from the TIFF file as NumPy array:
image = imread('temp.tif') image.shape (256, 256, 3)
Use the photometric
and planarconfig
arguments to write a 3x3x3 NumPy
array to an interleaved RGB, a planar RGB, or a 3-page grayscale TIFF:
data = numpy.random.randint(0, 255, (3, 3, 3), 'uint8') imwrite('temp.tif', data, photometric='rgb') imwrite('temp.tif', data, photometric='rgb', planarconfig='separate') imwrite('temp.tif', data, photometric='minisblack')
Use the extrasamples
argument to specify how extra components are
interpreted, for example, for an RGBA image with unassociated alpha channel:
data = numpy.random.randint(0, 255, (256, 256, 4), 'uint8') imwrite('temp.tif', data, photometric='rgb', extrasamples=['unassalpha'])
Write a 3-dimensional NumPy array to a multi-page, 16-bit grayscale TIFF file:
data = numpy.random.randint(0, 2**12, (64, 301, 219), 'uint16') imwrite('temp.tif', data, photometric='minisblack')
Read the whole image stack from the multi-page TIFF file as NumPy array:
image_stack = imread('temp.tif') image_stack.shape (64, 301, 219) image_stack.dtype dtype('uint16')
Read the image from the first page in the TIFF file as NumPy array:
image = imread('temp.tif', key=0) image.shape (301, 219)
Read images from a selected range of pages:
images = imread('temp.tif', key=range(4, 40, 2)) images.shape (18, 301, 219)
Iterate over all pages in the TIFF file and successively read images:
with TiffFile('temp.tif') as tif: ... for page in tif.pages: ... image = page.asarray() ...
Get information about the image stack in the TIFF file without reading any image data:
tif = TiffFile('temp.tif') len(tif.pages) # number of pages in the file 64 page = tif.pages[0] # get shape and dtype of image in first page page.shape (301, 219) page.dtype dtype('uint16') page.axes 'YX' series = tif.series[0] # get shape and dtype of first image series series.shape (64, 301, 219) series.dtype dtype('uint16') series.axes 'QYX' tif.close()
Inspect the "XResolution" tag from the first page in the TIFF file:
with TiffFile('temp.tif') as tif: ... tag = tif.pages[0].tags['XResolution'] ... tag.value (1, 1) tag.name 'XResolution' tag.code 282 tag.count 1 tag.dtype <DATATYPE.RATIONAL: 5>
Iterate over all tags in the TIFF file:
with TiffFile('temp.tif') as tif: ... for page in tif.pages: ... for tag in page.tags: ... tag_name, tag_value = tag.name, tag.value ...
Overwrite the value of an existing tag, for example, XResolution:
with TiffFile('temp.tif', mode='r+') as tif: ... _ = tif.pages[0].tags['XResolution'].overwrite((96000, 1000)) ...
Write a 5-dimensional floating-point array using BigTIFF format, separate color components, tiling, Zlib compression level 8, horizontal differencing predictor, and additional metadata:
data = numpy.random.rand(2, 5, 3, 301, 219).astype('float32') imwrite( ... 'temp.tif', ... data, ... bigtiff=True, ... photometric='rgb', ... planarconfig='separate', ... tile=(32, 32), ... compression='zlib', ... compressionargs={'level': 8}, ... predictor=True, ... metadata={'axes': 'TZCYX'}, ... )
Write a 10 fps time series of volumes with xyz voxel size 2.6755x2.6755x3.9474 micron^3 to an ImageJ hyperstack formatted TIFF file:
volume = numpy.random.randn(6, 57, 256, 256).astype('float32') image_labels = [f'{i}' for i in range(volume.shape[0] * volume.shape[1])] imwrite( ... 'temp.tif', ... volume, ... imagej=True, ... resolution=(1.0 / 2.6755, 1.0 / 2.6755), ... metadata={ ... 'spacing': 3.947368, ... 'unit': 'um', ... 'finterval': 1 / 10, ... 'fps': 10.0, ... 'axes': 'TZYX', ... 'Labels': image_labels, ... }, ... )
Read the volume and metadata from the ImageJ hyperstack file:
with TiffFile('temp.tif') as tif: ... volume = tif.asarray() ... axes = tif.series[0].axes ... imagej_metadata = tif.imagej_metadata ... volume.shape (6, 57, 256, 256) axes 'TZYX' imagej_metadata['slices'] 57 imagej_metadata['frames'] 6
Memory-map the contiguous image data in the ImageJ hyperstack file:
memmap_volume = memmap('temp.tif') memmap_volume.shape (6, 57, 256, 256) del memmap_volume
Create a TIFF file containing an empty image and write to the memory-mapped NumPy array (note: this does not work with compression or tiling):
memmap_image = memmap( ... 'temp.tif', shape=(256, 256, 3), dtype='float32', photometric='rgb' ... ) type(memmap_image) <class 'numpy.memmap'> memmap_image[255, 255, 1] = 1.0 memmap_image.flush() del memmap_image
Write two NumPy arrays to a multi-series TIFF file (note: other TIFF readers will not recognize the two series; use the OME-TIFF format for better interoperability):
series0 = numpy.random.randint(0, 255, (32, 32, 3), 'uint8') series1 = numpy.random.randint(0, 255, (4, 256, 256), 'uint16') with TiffWriter('temp.tif') as tif: ... tif.write(series0, photometric='rgb') ... tif.write(series1, photometric='minisblack') ...
Read the second image series from the TIFF file:
series1 = imread('temp.tif', series=1) series1.shape (4, 256, 256)
Successively write the frames of one contiguous series to a TIFF file:
data = numpy.random.randint(0, 255, (30, 301, 219), 'uint8') with TiffWriter('temp.tif') as tif: ... for frame in data: ... tif.write(frame, contiguous=True) ...
Append an image series to the existing TIFF file (note: this does not work with ImageJ hyperstack or OME-TIFF files):
data = numpy.random.randint(0, 255, (301, 219, 3), 'uint8') imwrite('temp.tif', data, photometric='rgb', append=True)
Create a TIFF file from a generator of tiles:
data = numpy.random.randint(0, 2**12, (31, 33, 3), 'uint16') def tiles(data, tileshape): ... for y in range(0, data.shape[0], tileshape[0]): ... for x in range(0, data.shape[1], tileshape[1]): ... yield data[y : y + tileshape[0], x : x + tileshape[1]] ... imwrite( ... 'temp.tif', ... tiles(data, (16, 16)), ... tile=(16, 16), ... shape=data.shape, ... dtype=data.dtype, ... photometric='rgb', ... )
Write a multi-dimensional, multi-resolution (pyramidal), multi-series OME-TIFF file with optional metadata. Sub-resolution images are written to SubIFDs. Limit parallel encoding to 2 threads. Write a thumbnail image as a separate image series:
data = numpy.random.randint(0, 255, (8, 2, 512, 512, 3), 'uint8') subresolutions = 2 pixelsize = 0.29 # micrometer with TiffWriter('temp.ome.tif', bigtiff=True) as tif: ... metadata = { ... 'axes': 'TCYXS', ... 'SignificantBits': 8, ... 'TimeIncrement': 0.1, ... 'TimeIncrementUnit': 's', ... 'PhysicalSizeX': pixelsize, ... 'PhysicalSizeXUnit': 'µm', ... 'PhysicalSizeY': pixelsize, ... 'PhysicalSizeYUnit': 'µm', ... 'Channel': {'Name': ['Channel 1', 'Channel 2']}, ... 'Plane': {'PositionX': [0.0] * 16, 'PositionXUnit': ['µm'] * 16}, ... 'Description': 'A multi-dimensional, multi-resolution image', ... 'MapAnnotation': { # for OMERO ... 'Namespace': 'openmicroscopy.org/PyramidResolution', ... '1': '256 256', ... '2': '128 128', ... }, ... } ... options = dict( ... photometric='rgb', ... tile=(128, 128), ... compression='jpeg', ... resolutionunit='CENTIMETER', ... maxworkers=2, ... ) ... tif.write( ... data, ... subifds=subresolutions, ... resolution=(1e4 / pixelsize, 1e4 / pixelsize), ... metadata=metadata, ... **options, ... ) ... # write pyramid levels to the two subifds ... # in production use resampling to generate sub-resolution images ... for level in range(subresolutions): ... mag = 2 ** (level + 1) ... tif.write( ... data[..., ::mag, ::mag, :], ... subfiletype=1, ... resolution=(1e4 / mag / pixelsize, 1e4 / mag / pixelsize), ... **options, ... ) ... # add a thumbnail image as a separate series ... # it is recognized by QuPath as an associated image ... thumbnail = (data[0, 0, ::8, ::8] >> 2).astype('uint8') ... tif.write(thumbnail, metadata={'Name': 'thumbnail'}) ...
Access the image levels in the pyramidal OME-TIFF file:
baseimage = imread('temp.ome.tif') second_level = imread('temp.ome.tif', series=0, level=1) with TiffFile('temp.ome.tif') as tif: ... baseimage = tif.series[0].asarray() ... second_level = tif.series[0].levels[1].asarray() ... number_levels = len(tif.series[0].levels) # includes base level ...
Iterate over and decode single JPEG compressed tiles in the TIFF file:
with TiffFile('temp.ome.tif') as tif: ... fh = tif.filehandle ... for page in tif.pages: ... for index, (offset, bytecount) in enumerate( ... zip(page.dataoffsets, page.databytecounts) ... ): ... _ = fh.seek(offset) ... data = fh.read(bytecount) ... tile, indices, shape = page.decode( ... data, index, jpegtables=page.jpegtables ... ) ...
Use Zarr 2 to read parts of the tiled, pyramidal images in the TIFF file:
import zarr store = imread('temp.ome.tif', aszarr=True) z = zarr.open(store, mode='r') z <zarr.hierarchy.Group '/' read-only> z[0] # base layer <zarr.core.Array '/0' (8, 2, 512, 512, 3) uint8 read-only> z[0][2, 0, 128:384, 256:].shape # read a tile from the base layer (256, 256, 3) store.close()
Load the base layer from the Zarr 2 store as a dask array:
import dask.array store = imread('temp.ome.tif', aszarr=True) dask.array.from_zarr(store, 0) dask.array<...shape=(8, 2, 512, 512, 3)...chunksize=(1, 1, 128, 128, 3)... store.close()
Write the Zarr 2 store to a fsspec ReferenceFileSystem in JSON format:
store = imread('temp.ome.tif', aszarr=True) store.write_fsspec('temp.ome.tif.json', url='file://') store.close()
Open the fsspec ReferenceFileSystem as a Zarr group:
import fsspec import imagecodecs.numcodecs imagecodecs.numcodecs.register_codecs() mapper = fsspec.get_mapper( ... 'reference://', fo='temp.ome.tif.json', target_protocol='file' ... ) z = zarr.open(mapper, mode='r') z <zarr.hierarchy.Group '/' read-only>
Create an OME-TIFF file containing an empty, tiled image series and write to it via the Zarr 2 interface (note: this does not work with compression):
imwrite( ... 'temp2.ome.tif', ... shape=(8, 800, 600), ... dtype='uint16', ... photometric='minisblack', ... tile=(128, 128), ... metadata={'axes': 'CYX'}, ... ) store = imread('temp2.ome.tif', mode='r+', aszarr=True) z = zarr.open(store, mode='r+') z <zarr.core.Array (8, 800, 600) uint16> z[3, 100:200, 200:300:2] = 1024 store.close()
Read images from a sequence of TIFF files as NumPy array using two I/O worker threads:
imwrite('temp_C001T001.tif', numpy.random.rand(64, 64)) imwrite('temp_C001T002.tif', numpy.random.rand(64, 64)) image_sequence = imread( ... ['temp_C001T001.tif', 'temp_C001T002.tif'], ioworkers=2, maxworkers=1 ... ) image_sequence.shape (2, 64, 64) image_sequence.dtype dtype('float64')
Read an image stack from a series of TIFF files with a file name pattern as NumPy or Zarr 2 arrays:
image_sequence = TiffSequence('temp_C0*.tif', pattern=r'_(C)(\d+)(T)(\d+)') image_sequence.shape (1, 2) image_sequence.axes 'CT' data = image_sequence.asarray() data.shape (1, 2, 64, 64) store = image_sequence.aszarr() zarr.open(store, mode='r') <zarr.core.Array (1, 2, 64, 64) float64 read-only> image_sequence.close()
Write the Zarr 2 store to a fsspec ReferenceFileSystem in JSON format:
store = image_sequence.aszarr() store.write_fsspec('temp.json', url='file://')
Open the fsspec ReferenceFileSystem as a Zarr 2 array:
import fsspec import tifffile.numcodecs tifffile.numcodecs.register_codec() mapper = fsspec.get_mapper( ... 'reference://', fo='temp.json', target_protocol='file' ... ) zarr.open(mapper, mode='r') <zarr.core.Array (1, 2, 64, 64) float64 read-only>
Inspect the TIFF file from the command line::
$ python -m tifffile temp.ome.tif
FAQs
Read and write TIFF files
We found that tifffile demonstrated a healthy version release cadence and project activity because the last version was released less than a year ago. It has 1 open source maintainer collaborating on the project.
Did you know?
Socket for GitHub automatically highlights issues in each pull request and monitors the health of all your open source dependencies. Discover the contents of your packages and block harmful activity before you install or update your dependencies.
Security News
Opengrep forks Semgrep to preserve open source SAST in response to controversial licensing changes.
Security News
Critics call the Node.js EOL CVE a misuse of the system, sparking debate over CVE standards and the growing noise in vulnerability databases.
Security News
cURL and Go security teams are publicly rejecting CVSS as flawed for assessing vulnerabilities and are calling for more accurate, context-aware approaches.