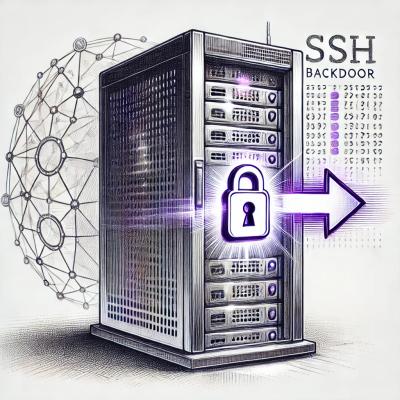
Research
Security News
Malicious npm Packages Inject SSH Backdoors via Typosquatted Libraries
Socket’s threat research team has detected six malicious npm packages typosquatting popular libraries to insert SSH backdoors.
A high-performance implementation of 3D RANSAC (Random Sample Consensus) algorithm using PyTorch and CUDA.
A high-performance implementation of 3D RANSAC algorithm using PyTorch and CUDA.
Explore the docs »
View Demo
·
Report Bug
·
Request Feature
Requirements: torch
Install with PyPI :
pip install torch-ransac3d
import torch
import numpy as np
from torch_ransac3d.line import line_fit
# Using PyTorch tensor
points_torch = torch.rand(1000, 3)
direction, point, inliers = line_fit(
pts=points_torch,
thresh=0.01,
max_iterations=1000,
iterations_per_batch=100,
epsilon=1e-8,
device=torch.device("cuda" if torch.cuda.is_available() else "cpu")
)
# Using NumPy array
points_numpy = np.random.rand(1000, 3)
direction, point, inliers = line_fit(
pts=points_numpy,
thresh=0.01,
max_iterations=1000,
iterations_per_batch=100,
epsilon=1e-8,
device=torch.device("cuda" if torch.cuda.is_available() else "cpu")
)
from torch_ransac3d.plane import plane_fit
# Works with both PyTorch tensors and NumPy arrays
points = torch.rand(1000, 3) # or np.random.rand(1000, 3)
equation, inliers = plane_fit(
pts=points,
thresh=0.05,
max_iterations=1000,
iterations_per_batch=100,
epsilon=1e-8,
device=torch.device("cuda" if torch.cuda.is_available() else "cpu")
)
from torch_ransac3d.sphere import sphere_fit
# Works with both PyTorch tensors and NumPy arrays
points = torch.rand(1000, 3) # or np.random.rand(1000, 3)
center, radius, inliers = sphere_fit(
pts=points,
thresh=0.05,
max_iterations=1000,
iterations_per_batch=100,
epsilon=1e-8,
device=torch.device("cuda" if torch.cuda.is_available() else "cpu")
)
pts
: Input point cloud (torch.Tensor or numpy.ndarray of shape (N, 3))thresh
: Distance threshold for considering a point as an inliermax_iterations
: Maximum number of RANSAC iterationsiterations_per_batch
: Number of iterations to process in parallelepsilon
: Small value to avoid division by zerodevice
: Torch device to run computations on (CPU or CUDA)All fitting functions support both PyTorch tensors and NumPy arrays as input. The library automatically converts NumPy arrays to PyTorch tensors internally, allowing for seamless integration with various data formats.
All fitting functions support batch processing to improve performance. The iterations_per_batch
parameter determines how many RANSAC iterations are processed in parallel, leading to significant speedups on GPU hardware.
This project is based on the work done at https://github.com/leomariga/pyRANSAC-3D/
Maintainer: Harry Dobbs
Email: harrydobbs87@gmail.com
FAQs
A high-performance implementation of 3D RANSAC (Random Sample Consensus) algorithm using PyTorch and CUDA.
We found that torch-ransac3d demonstrated a healthy version release cadence and project activity because the last version was released less than a year ago. It has 1 open source maintainer collaborating on the project.
Did you know?
Socket for GitHub automatically highlights issues in each pull request and monitors the health of all your open source dependencies. Discover the contents of your packages and block harmful activity before you install or update your dependencies.
Research
Security News
Socket’s threat research team has detected six malicious npm packages typosquatting popular libraries to insert SSH backdoors.
Security News
MITRE's 2024 CWE Top 25 highlights critical software vulnerabilities like XSS, SQL Injection, and CSRF, reflecting shifts due to a refined ranking methodology.
Security News
In this segment of the Risky Business podcast, Feross Aboukhadijeh and Patrick Gray discuss the challenges of tracking malware discovered in open source softare.