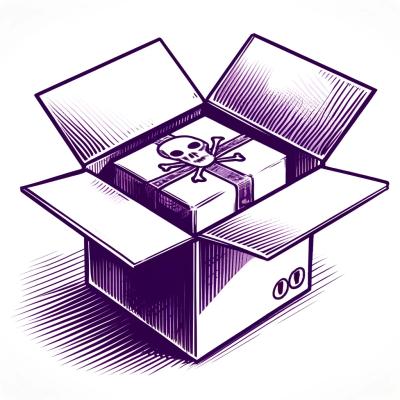
Security News
Research
Data Theft Repackaged: A Case Study in Malicious Wrapper Packages on npm
The Socket Research Team breaks down a malicious wrapper package that uses obfuscation to harvest credentials and exfiltrate sensitive data.
Version | Docs | Tests | Coverage | Style | PyPI | Python | PyTorch | Docker |
---|---|---|---|---|---|---|---|---|
torchlayers is a library based on PyTorch
providing automatic shape and dimensionality inference of torch.nn
layers + additional
building blocks featured in current SOTA architectures (e.g. Efficient-Net).
Above requires no user intervention (except single call to torchlayers.build
)
similarly to the one seen in Keras.
torch.nn
module (convolutional, recurrent, transformer, attention and linear layers)torchlayers.Conv
working as torch.nn.Conv1d/2d/3d
based on input shape
)torchlayers.Reshape
or torchlayers.StandardNormalNoise
)"same"
padding and default kernel_size=3
for Conv
, dropout rates etc.)Keep in mind this library works almost exactly like PyTorch originally.
What that means is you can use Sequential
, define your own networks of any complexity using
torch.nn.Module
, create new layers with shape inference etc.
See below to get some intuition about library.
For full functionality please check torchlayers documentation. Below examples should introduce all necessary concepts you should know.
All torch.nn
modules can be used through torchlayers
and each module with input shape
will be appropriately modified with it's input inferable counterpart.
import torchlayers as tl
class Classifier(tl.Module):
def __init__(self):
super().__init__()
self.conv1 = tl.Conv2d(64, kernel_size=6)
self.conv2 = tl.Conv2d(128, kernel_size=3)
self.conv3 = tl.Conv2d(256, kernel_size=3, padding=1)
# New layer, more on that in the next example
self.pooling = tl.GlobalMaxPool()
self.dense = tl.Linear(10)
def forward(self, x):
x = torch.relu(self.conv1(x))
x = torch.relu(self.conv2(x))
x = torch.relu(self.conv3(x))
return self.dense(self.pooling(x))
# Pass model and any example inputs afterwards
clf = tl.build(Classifier(), torch.randn(1, 3, 32, 32))
Above torchlayers.Linear(out_features=10)
is used. It is "equivalent" to
original PyTorch's torch.nn.Linear(in_features=?, out_features=10)
where in_features
will be inferred from example input input during torchlayers.build
call.
Same thing happens with torch.nn.Conv2d(in_channels, out_channels, kernel_size, ...)
which can be replaced directly by tl.Conv2d(out_channels, kernel_size, ...)
.
Just remember to pass example input through the network!
torch.nn
and torchlayers
:import torch
import torchlayers as tl
# torch.nn and torchlayers can be mixed easily
model = torch.nn.Sequential(
tl.Conv(64), # specify ONLY out_channels
torch.nn.ReLU(), # use torch.nn wherever you wish
tl.BatchNorm(), # BatchNormNd inferred from input
tl.Conv(128), # Default kernel_size equal to 3
tl.ReLU(),
tl.Conv(256, kernel_size=11), # "same" padding as default
tl.GlobalMaxPool(), # Known from Keras
tl.Linear(10), # Output for 10 classes
)
print(model)
Above would give you model's summary like this (notice question marks for not yet inferred values):
Sequential(
(0): Conv(in_channels=?, out_channels=64, kernel_size=3, stride=1, padding=same, dilation=1, groups=1, bias=True, padding_mode=zeros)
(1): ReLU()
(2): BatchNorm(num_features=?, eps=1e-05, momentum=0.1, affine=True, track_running_stats=True)
(3): Conv(in_channels=?, out_channels=128, kernel_size=3, stride=1, padding=same, dilation=1, groups=1, bias=True, padding_mode=zeros)
(4): ReLU()
(5): Conv(in_channels=?, out_channels=256, kernel_size=11, stride=1, padding=same, dilation=1, groups=1, bias=True, padding_mode=zeros)
(6): GlobalMaxPool()
(7): Linear(in_features=?, out_features=10, bias=True)
)
mnist_model = tl.build(model, torch.randn(1, 3, 28, 28))
input shape
(e.g. for text classification using 300
dimensional pretrained embedding):# [batch, embedding, timesteps], first dimension > 1 for BatchNorm1d to work
text_model = tl.build(model, torch.randn(2, 300, 1))
print
both models after instantiation, provided below side
by-side for readability (notice different dimenstionality, e.g. Conv2d
vs Conv1d
after torchlayers.build
): # TEXT CLASSIFIER MNIST CLASSIFIER
Sequential( Sequential(
(0): Conv1d(300, 64) (0): Conv2d(3, 64)
(1): ReLU() (1): ReLU()
(2): BatchNorm1d(64) (2): BatchNorm2d(64)
(3): Conv1d(64, 128) (3): Conv2d(64, 128)
(4): ReLU() (4): ReLU()
(5): Conv1d(128, 256) (5): Conv2d(128, 256)
(6): GlobalMaxPool() (6): GlobalMaxPool()
(7): Linear(256, 10) (7): Linear(256, 10)
) )
As you can see both modules "compiled" into original pytorch
layers.
User can define any module and make it shape inferable with torchlayers.infer
function:
# Class defined with in_features
# It might be a good practice to use _ prefix and Impl as postfix
# to differentiate from shape inferable version
class _MyLinearImpl(torch.nn.Module):
def __init__(self, in_features: int, out_features: int):
super().__init__()
self.weight = torch.nn.Parameter(torch.randn(out_features, in_features))
self.bias = torch.nn.Parameter(torch.randn(out_features))
def forward(self, inputs):
return torch.nn.functional.linear(inputs, self.weight, self.bias)
MyLinear = tl.infer(_MyLinearImpl)
# Build and use just like any other layer in this library
layer =tl.build(MyLinear(out_features=32), torch.randn(1, 64))
layer(torch.randn(1, 64))
By default inputs.shape[1]
will be used as in_features
value
during initial forward
pass. If you wish to use different index
(e.g. to infer using
inputs.shape[3]
) use MyLayer = tl.infer(_MyLayerImpl, index=3)
as a decorator.
Please check code comments and documentation if needed. If you are unsure what autoencoder is you could see this example blog post.
Below is a convolutional denoising autoencoder example for ImageNet
-like images.
Think of it like a demonstration of capabilities of different layers
and building blocks provided by torchlayers
.
# Input - 3 x 256 x 256 for ImageNet reconstruction
class AutoEncoder(torch.nn.Module):
def __init__(self):
super().__init__()
self.encoder = tl.Sequential(
tl.StandardNormalNoise(), # Apply noise to input images
tl.Conv(64, kernel_size=7),
tl.activations.Swish(), # Direct access to module .activations
tl.InvertedResidualBottleneck(squeeze_excitation=False),
tl.AvgPool(), # shape 64 x 128 x 128, kernel_size=2 by default
tl.HardSwish(), # Access simply through tl
tl.SeparableConv(128), # Up number of channels to 128
tl.InvertedResidualBottleneck(), # Default with squeeze excitation
torch.nn.ReLU(),
tl.AvgPool(), # shape 128 x 64 x 64, kernel_size=2 by default
tl.DepthwiseConv(256), # DepthwiseConv easier to use
# Pass input thrice through the same weights like in PolyNet
tl.Poly(tl.InvertedResidualBottleneck(), order=3),
tl.ReLU(), # all torch.nn can be accessed via tl
tl.MaxPool(), # shape 256 x 32 x 32
tl.Fire(out_channels=512), # shape 512 x 32 x 32
tl.SqueezeExcitation(hidden=64),
tl.InvertedResidualBottleneck(),
tl.MaxPool(), # shape 512 x 16 x 16
tl.InvertedResidualBottleneck(squeeze_excitation=False),
# Randomly switch off the last two layers with 0.5 probability
tl.StochasticDepth(
torch.nn.Sequential(
tl.InvertedResidualBottleneck(squeeze_excitation=False),
tl.InvertedResidualBottleneck(squeeze_excitation=False),
),
p=0.5,
),
tl.AvgPool(), # shape 512 x 8 x 8
)
# This one is more "standard"
self.decoder = tl.Sequential(
tl.Poly(tl.InvertedResidualBottleneck(), order=2),
# Has ICNR initialization by default after calling `build`
tl.ConvPixelShuffle(out_channels=512, upscale_factor=2),
# Shape 512 x 16 x 16 after PixelShuffle
tl.Poly(tl.InvertedResidualBottleneck(), order=3),
tl.ConvPixelShuffle(out_channels=256, upscale_factor=2),
# Shape 256 x 32 x 32
tl.Poly(tl.InvertedResidualBottleneck(), order=3),
tl.ConvPixelShuffle(out_channels=128, upscale_factor=2),
# Shape 128 x 64 x 64
tl.Poly(tl.InvertedResidualBottleneck(), order=4),
tl.ConvPixelShuffle(out_channels=64, upscale_factor=2),
# Shape 64 x 128 x 128
tl.InvertedResidualBottleneck(),
tl.Conv(256),
tl.Dropout(), # Defaults to 0.5 and Dropout2d for images
tl.Swish(),
tl.InstanceNorm(),
tl.ConvPixelShuffle(out_channels=32, upscale_factor=2),
# Shape 32 x 256 x 256
tl.Conv(16),
tl.Swish(),
tl.Conv(3),
# Shape 3 x 256 x 256
)
def forward(self, inputs):
return self.decoder(self.encoder(inputs))
Now one can instantiate the module and use it with torch.nn.MSELoss
as per usual.
autoencoder = tl.build(AutoEncoder(), torch.randn(1, 3, 256, 256))
pip install --user torchlayers
pip install --user torchlayers-nightly
CPU standalone and various versions of GPU enabled images are available at dockerhub.
For CPU quickstart, issue:
docker pull szymonmaszke/torchlayers:18.04
Nightly builds are also available, just prefix tag with nightly_
. If you are going for GPU
image make sure you have
nvidia/docker installed and it's runtime set.
If you find issue or would like to see some functionality (or implement one), please open new Issue or create Pull Request.
FAQs
Input shape inference and SOTA custom layers for PyTorch.
We found that torchlayers-nightly demonstrated a healthy version release cadence and project activity because the last version was released less than a year ago. It has 1 open source maintainer collaborating on the project.
Did you know?
Socket for GitHub automatically highlights issues in each pull request and monitors the health of all your open source dependencies. Discover the contents of your packages and block harmful activity before you install or update your dependencies.
Security News
Research
The Socket Research Team breaks down a malicious wrapper package that uses obfuscation to harvest credentials and exfiltrate sensitive data.
Research
Security News
Attackers used a malicious npm package typosquatting a popular ESLint plugin to steal sensitive data, execute commands, and exploit developer systems.
Security News
The Ultralytics' PyPI Package was compromised four times in one weekend through GitHub Actions cache poisoning and failure to rotate previously compromised API tokens.