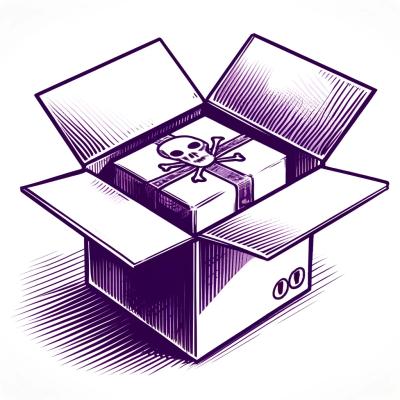
Security News
Research
Data Theft Repackaged: A Case Study in Malicious Wrapper Packages on npm
The Socket Research Team breaks down a malicious wrapper package that uses obfuscation to harvest credentials and exfiltrate sensitive data.
A trainer based on pytorch including a train loop for MDN (Mixture Density Network), a data loader, plot line chart and a couple of techniques for avoid over fitting
This package needs Python>=3.7 and the version of Pytorch used in development is 1.13.1 and cuda11.2, considering the different version of cuda, the package will not install Pytorch automatically. You should check your cuda's version, install the suitable pytorch first. Then, run the command below:
pip install tortreinador
from tortreinador.utils.plot import plot_line_2
from tortreinador.utils.preprocessing import load_data
from tortreinador.train import TorchTrainer, config_generator
from tortreinador.models.MDN import mdn, Mixture, NLLLoss
from tortreinador.utils.tools import xavier_init
from tortreinador.utils.View import init_weights, split_weights
import torch
import pandas as pd
data = pd.read_excel('')
data['M_total (M_E)'] = data['Mcore (M_J/10^3)'] + data['Menv (M_E)']
# Support index, e.g input_parameters = [0, 1, 2]
input_parameters = [
'Mass (M_J)',
'Radius (R_E)',
'T_sur (K)',
]
output_parameters = [
'M_total (M_E)',
'T_int (K)',
'P_CEB (Mbar)',
'T_CEB (K)'
]
# Load Data, random status default as 42
t_loader, v_loader, test_x, test_y, s_x, s_y = load_data(data=data, input_parameters=input_parameters,
output_parameters=output_parameters,
if_normal=True, if_shuffle=True, batch_size=512, feature_range=(0, 1), if_double=True, n_workers=4)
model = mdn(len(input_parameters), len(output_parameters), 20, 512)
criterion = NLLLoss()
optim = torch.optim.Adam(xavier_init(model), lr=0.0001, weight_decay=0.001)
'''
Overwrite function 'calculate'
'''
# class Trainer(TorchTrainer):
# def calculate(self, x, y, mode='t'):
# x_o, x_n = x.chunk(2, dim=1)
# pi, mu, sig = model(x_o, x_n)
# loss = self.criterion(pi, mu, sig, y)
# pdf = mixture(pi, mu, sig)
# y_pred = pdf.sample()
# metric_per = r2_score(y, y_pred)
# return self._standard_return(loss=loss, metric_per=metric_per, mode=mode, y=y, y_pred=y_pred)
# trainer = Trainer(is_gpu=True, epoch=50, optimizer=optim, model=model, criterion=criterion)
trainer = TorchTrainer(is_gpu=True, epoch=50, optimizer=optim, model=model, criterion=criterion)
save_file_path = '/notebooks/DeepExo/Resource/MDN_ATTN_15_error/'
config = config_generator(save_file_path, warmup_epochs=5, best_metric=0.8, lr_milestones=[12, 22, 36, 67, 75, 89, 106], lr_decay_rate=0.7)
# Training
result = trainer.fit(t_loader, v_loader, **config)
# Plot line chart
result_pd = pd.DataFrame()
result_pd['epoch'] = len(result[0])
result_pd['train_r2_avg'] = result[4]
result_pd['val_r2_avg'] = result[3]
plot_line_2(y_1='train_r2_avg', y_2='val_r2_avg', df=result_pd, fig_size=(10, 6))
# If specify 'mode' in TorchTrainer as 'csv'
saved_result = pd.read_csv('/notebooks/DeepExo/train_log/log_202408280744.csv')
plot_line_2(y_1='train_loss', y_2='val_loss', df=saved_result)
Please visit https://ardentex.github.io/tortreinador/
FAQs
A trainer based on Pytorch
We found that tortreinador demonstrated a healthy version release cadence and project activity because the last version was released less than a year ago. It has 1 open source maintainer collaborating on the project.
Did you know?
Socket for GitHub automatically highlights issues in each pull request and monitors the health of all your open source dependencies. Discover the contents of your packages and block harmful activity before you install or update your dependencies.
Security News
Research
The Socket Research Team breaks down a malicious wrapper package that uses obfuscation to harvest credentials and exfiltrate sensitive data.
Research
Security News
Attackers used a malicious npm package typosquatting a popular ESLint plugin to steal sensitive data, execute commands, and exploit developer systems.
Security News
The Ultralytics' PyPI Package was compromised four times in one weekend through GitHub Actions cache poisoning and failure to rotate previously compromised API tokens.