Think training a ResNet-18 on CIFAR-10 is a breeze? 🌬️💨
It might seem simple at first — until you find yourself drowning in boilerplate code:
- Setting up data loaders
- Defining model architectures
- Configuring loss functions
- Choosing and tuning optimizers
- ...and so much more! 🤯
What if you could skip all that hassle?
With this approach, you won't have to write a single line of code — just define a YAML configuration file:
batch_size: 256
num_workers: 8
epochs: 90
init_lr: 1.e-1
optimizer: SGD
optimizer_cfg:
momentum: 0.9
weight_decay: 1.e-4
lr_scheduler: StepLR
lr_scheduler_cfg:
step_size: 30
gamma: 0.1
criterion: CrossEntropyLoss
and simply run:
wah train --dataset cifar10 --dataset-root ./dataset --model resnet18 \
--cfg-path ./config.yaml --log-root ./logs --device auto
What Happens Next?
This single command will:
✅ Automatically download CIFAR-10 to ./dataset
✅ Train a ResNet-18 model on it
✅ Save checkpoints and TensorBoard logs to ./logs
✅ Detect available hardware (CPU/GPU) with multi-GPU support (DDP)
No tedious setup, no redundant scripting — just efficient, streamlined model training. 🚀
And that’s just the beginning!
You’ve found more than just a training tool — a powerful, flexible framework designed to accelerate deep learning research.
Produly presents:
WAH
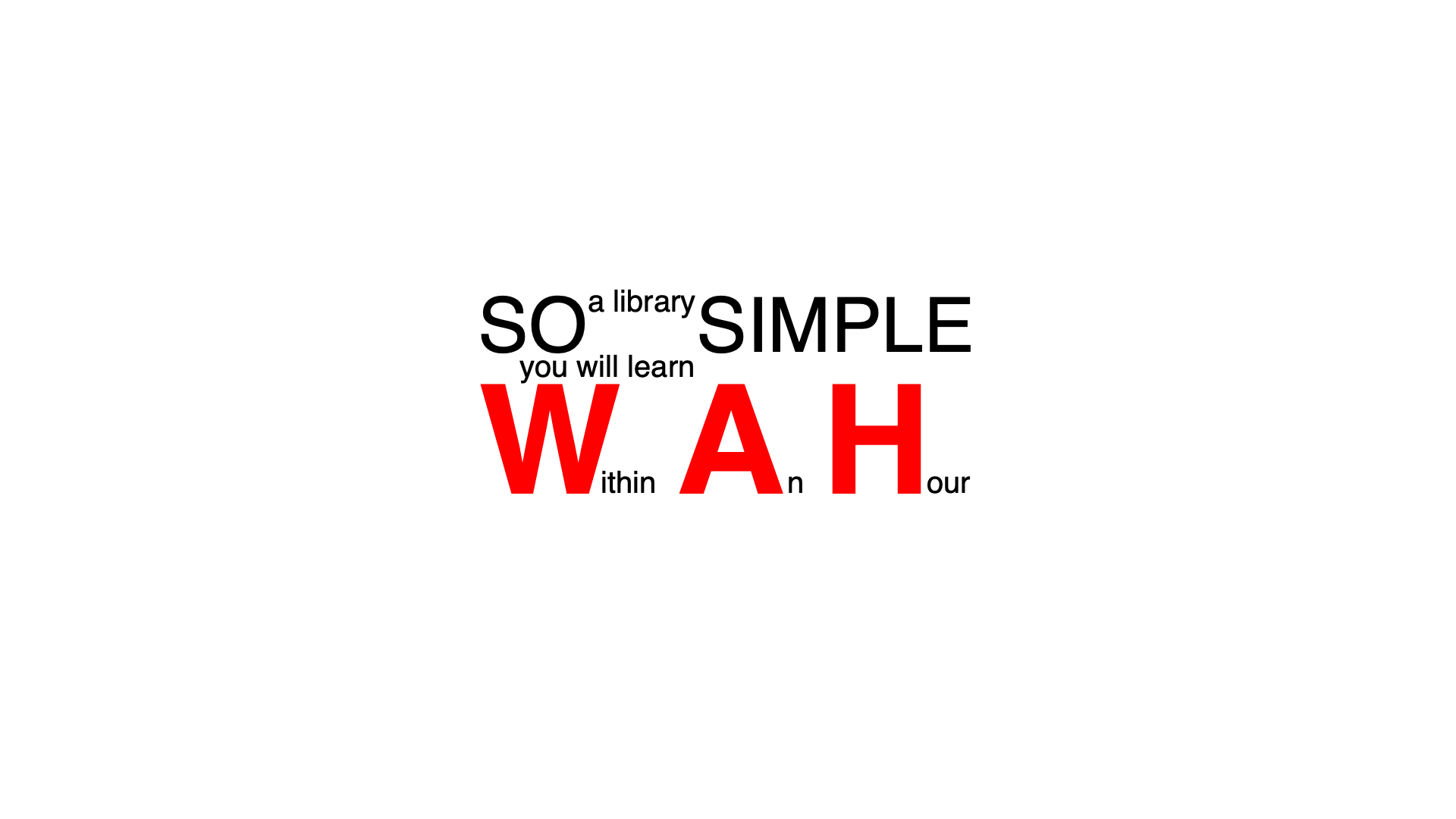
Install
pip install wah
Requirements
You might want to manually install PyTorch
for GPU computation.
lightning
matplotlib
numpy
pandas
pillow
requests
timm
torch
torchmetrics
torchvision
tqdm
yaml
Structure
wah
classification
datasets
CIFAR10
CIFAR100
compute_mean_and_std
ImageNet
load_dataloader
portion_dataset
STL10
models
FeatureExtractor
load
load_state_dict
replace
gelu_with_relu
relu_with_gelu
bn_with_ln
ln_with_bn
summary
test
Trainer
dicts
fun
lists
mods
path
random
tensor
time
utils
ArgumentParser
download
zips