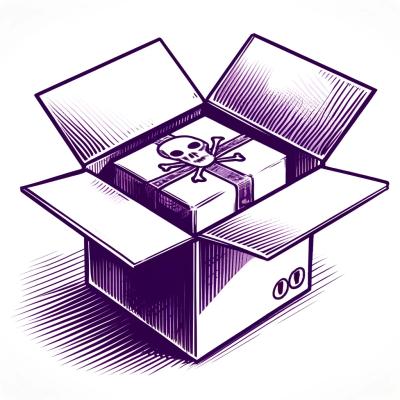
Security News
Research
Data Theft Repackaged: A Case Study in Malicious Wrapper Packages on npm
The Socket Research Team breaks down a malicious wrapper package that uses obfuscation to harvest credentials and exfiltrate sensitive data.
Leva is a Ruby on Rails framework for evaluating Language Models (LLMs) using ActiveRecord datasets on production models. It provides a flexible structure for creating experiments, managing datasets, and implementing various evaluation logic on production data with security in mind.
Add this line to your application's Gemfile:
gem 'leva'
And then execute:
bundle install
Add the migrations to your database:
rails leva:install:migrations
rails db:migrate
First, create a dataset and add any ActiveRecord records you want to evaluate against. To make your models compatible with Leva, include the Leva::Recordable
concern in your model:
class TextContent < ApplicationRecord
include Leva::Recordable
# @return [String] The ground truth label for the record
def ground_truth
expected_label
end
# @return [Hash] A hash of attributes to be displayed in the dataset records index
def index_attributes
{
text: text,
expected_label: expected_label,
created_at: created_at.strftime('%Y-%m-%d %H:%M:%S')
}
end
# @return [Hash] A hash of attributes to be displayed in the dataset record show view
def show_attributes
{
text: text,
expected_label: expected_label,
created_at: created_at.strftime('%Y-%m-%d %H:%M:%S')
}
end
# @return [Hash] A hash of attributes to be displayed in the dataset record show view
def to_llm_context
{
text: text,
expected_label: expected_label,
created_at: created_at.strftime('%Y-%m-%d %H:%M:%S')
}
end
end
dataset = Leva::Dataset.create(name: "Sentiment Analysis Dataset") dataset.add_record TextContent.create(text: "I love this product!", expected_label: "Positive") dataset.add_record TextContent.create(text: "Terrible experience", expected_label: "Negative") dataset.add_record TextContent.create(text: "It's ok", expected_label: "Neutral")
### 2. Implementing Runs
Create a run class to handle the execution of your inference logic:
```bash
rails generate leva:runner sentiment
class SentimentRun < Leva::BaseRun
def execute(record)
# Your model execution logic here
# This could involve calling an API, running a local model, etc.
# Return the model's output
end
end
Create one or more eval classes to evaluate the model's output:
rails generate leva:eval sentiment_accuracy
class SentimentAccuracyEval < Leva::BaseEval
def evaluate(prediction, record)
score = prediction == record.expected_label ? 1.0 : 0.0
[score, record.expected_label]
end
end
class SentimentF1Eval < Leva::BaseEval
def evaluate(prediction, record)
# Calculate F1 score
# ...
[f1_score, record.f1_score]
end
end
You can run experiments with different runs and evals:
experiment = Leva::Experiment.create!(name: "Sentiment Analysis", dataset: dataset)
run = SentimentRun.new
evals = [SentimentAccuracyEval.new, SentimentF1Eval.new]
Leva.run_evaluation(experiment: experiment, run: run, evals: evals)
You can also use prompts with your runs:
prompt = Leva::Prompt.create!(
name: "Sentiment Analysis",
version: 1,
system_prompt: "You are an expert at analyzing text and returning the sentiment.",
user_prompt: "Please analyze the following text and return the sentiment as Positive, Negative, or Neutral.\n\n{{TEXT}}",
metadata: { model: "gpt-4", temperature: 0.5 }
)
experiment = Leva::Experiment.create!(
name: "Sentiment Analysis with LLM",
dataset: dataset,
prompt: prompt
)
run = SentimentRun.new
evals = [SentimentAccuracyEval.new, SentimentF1Eval.new]
Leva.run_evaluation(experiment: experiment, run: run, evals: evals)
After the experiments are complete, analyze the results:
experiment.evaluation_results.group_by(&:evaluator_class).each do |evaluator_class, results|
average_score = results.average(&:score)
puts "#{evaluator_class.capitalize} Average Score: #{average_score}"
end
Ensure you set up any required API keys or other configurations in your Rails credentials or environment variables.
Leva
: Handles the process of running experiments.Leva::BaseRun
: Base class for run implementations.Leva::BaseEval
: Base class for eval implementations.Leva::Dataset
: Represents a collection of data to be evaluated.Leva::DatasetRecord
: Represents individual records within a dataset.Leva::Experiment
: Represents a single run of an evaluation on a dataset.Leva::RunnerResult
: Stores the results of each run execution.Leva::EvaluationResult
: Stores the results of each evaluation.Leva::Prompt
: Represents a prompt for an LLM.Bug reports and pull requests are welcome on GitHub at https://github.com/kieranklaassen/leva.
The gem is available as open source under the terms of the MIT License.
FAQs
Unknown package
We found that leva demonstrated a healthy version release cadence and project activity because the last version was released less than a year ago. It has 1 open source maintainer collaborating on the project.
Did you know?
Socket for GitHub automatically highlights issues in each pull request and monitors the health of all your open source dependencies. Discover the contents of your packages and block harmful activity before you install or update your dependencies.
Security News
Research
The Socket Research Team breaks down a malicious wrapper package that uses obfuscation to harvest credentials and exfiltrate sensitive data.
Research
Security News
Attackers used a malicious npm package typosquatting a popular ESLint plugin to steal sensitive data, execute commands, and exploit developer systems.
Security News
The Ultralytics' PyPI Package was compromised four times in one weekend through GitHub Actions cache poisoning and failure to rotate previously compromised API tokens.