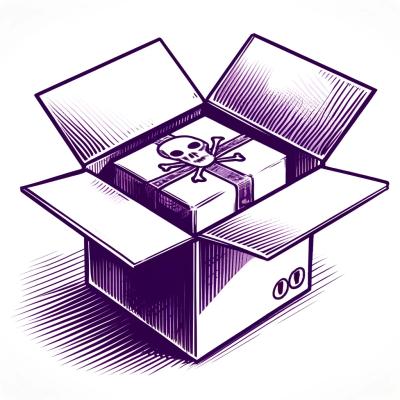
Security News
Research
Data Theft Repackaged: A Case Study in Malicious Wrapper Packages on npm
The Socket Research Team breaks down a malicious wrapper package that uses obfuscation to harvest credentials and exfiltrate sensitive data.
Plain Ruby implementation of Levenshtein distance algorithm that measures the similarity of two strings.
This implementation is intended for educational use to study the algorithm itself by the clean syntactic structure of Ruby. Use it in production with caution. To compare really large strings you may want to use a Ruby gem that relies on native C code extensions or similar like
levenshtein_rb was inspired by this example implementation.
During my heuristically flavoured (and therefore not really meaningful) benchmark I took 1.28 seconds to compute the Levenshtein distance of strings of length 1000 and ~ 30 seconds to compute the Levenshtein distance of strings of length 10000. Theoretically the algorithm itself is of complexity O(mn) where m and n are the sizes of the input strings.
Add this line to your application's Gemfile:
gem 'levenshtein_rb'
And then execute:
$ bundle
Or install it yourself as:
$ gem install levenshtein_rb
LevenshteinRb::LevenshteinDistance.new('Tor', 'Tier').to_i # => 2
The mathematical formalism is well-explained at wikipedia, so let's concentrate on the example here. Let's examine the algorithm on explaining the steps with the example word-word combination x For a human being it is convenient to perform the algorithm by "filling a table". The table of numbers is the "recurrence matrix" in the Ruby code. In the mathematical literature, the recurrence matrix usually is denoted by D.
One starts with the upper left corner that refers to the pair of words (ε,ε). The corresponding matrix entry D[0,0] gets as value a zero, which means: Zero changes are necessary to turn the word ε into ε.
Let's now have a look on the first row:
| ε T o r
-----------
ε | 0 1 2 3
The semantics of the entries D[0,1], D[0,2] and D[0,3] are as follows: It takes one elementary change (namely an addition) to turn the word ε into εT. And it takes 2 additions to turn ε into εTo and 3 additions to turn ε into εTor. The whole first column workds similar.
More interesting are the values (a.k.a "costs") of an entry that corresponds to an inner element of the matrix.
Take, for example the matrix entry D[1,1] with the assumption that the first row and the whole first column already was calculated (which is easy because it's the same procedure for any word-word combination). So concentrate on the submatrix:
| ε T
-------
ε | 0 1
T | 1 ?
↑
This is D[1,1]
D[1,1] corresponds to the question: How many steps does it take to turn εT into εT? And the answer is easy: We've added the same character ("T") to the word ε on both dimensions. So the answer is: Zero.
Now we have obtained D[1,1], lets continue and fill the whole second row:
| ε T o r
-----------
ε | 0 1 2 3
T | 1 0 ? ?
How to get D[1,2] ? The "same character logic" is not the case here. D[1,2] corresponds to an addition of the character "o" (or a deletion if you see it vice versa). 1 o needs to be added so it costs exactly one more starting from? Right, we choose "the best path" so we choose the mininal value that is a neighbor an already has been computed. In our case it is D[1,1]. D[1,2] has exactly +1 costs more and therefore D[1,2] = D[1,1] + 1 = 1.
This is the central trick: One always looks for the "minimum value" that corresponds to a neighbor entry calculated before and adds a cost (in our case we define all costs by +1)
If one follows the strategy successively one obtains:
| ε T o r
-----------
ε | 0 1 2 3
T | 1 0 1 2
i | 2 1 1 2
e | 3 2 2 2
r | 4 3 3 2
↑
This last value is the Levenshtein distance
The entry D[m,n] is called the Levenshtein distance of the two input words.
FAQs
Unknown package
We found that levenshtein_rb demonstrated a not healthy version release cadence and project activity because the last version was released a year ago. It has 1 open source maintainer collaborating on the project.
Did you know?
Socket for GitHub automatically highlights issues in each pull request and monitors the health of all your open source dependencies. Discover the contents of your packages and block harmful activity before you install or update your dependencies.
Security News
Research
The Socket Research Team breaks down a malicious wrapper package that uses obfuscation to harvest credentials and exfiltrate sensitive data.
Research
Security News
Attackers used a malicious npm package typosquatting a popular ESLint plugin to steal sensitive data, execute commands, and exploit developer systems.
Security News
The Ultralytics' PyPI Package was compromised four times in one weekend through GitHub Actions cache poisoning and failure to rotate previously compromised API tokens.