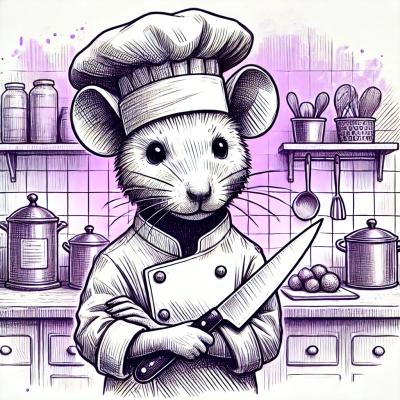
Research
Security News
Quasar RAT Disguised as an npm Package for Detecting Vulnerabilities in Ethereum Smart Contracts
Socket researchers uncover a malicious npm package posing as a tool for detecting vulnerabilities in Etherium smart contracts.
ml-savitzky-golay-generalized
Advanced tools
General Least-Squares Smoothing and Differentiation by the Convolution (Savitzky-Golay) Method Peter A. Gorry
Pretty much the same as the savitzky-golay method, but without border problems, and without inventing points. It can be maybe merged into the savitzky-golay project but by the now this is the version used in the last GSD project.
I'll try an automatic parameter tunning based on the SNR or in the entropy of the signal.
npm i ml-savitzky-golay-generalized
const {sgg} = require("ml-savitzky-golay-generalized");
sgg(dataY, deltaX|X, options)
The data to be filtered.
deltaX specifies the difference between 2 consecutive points of the independent: deltaX = X[i+1] - X[i]. Specficiying a deltaX suppose that all your points are equally spaced on the independent variable. If your points are not equally spaced in the ordinate variable, then you have to provide explicitly your X values. The algorithm will use the average deltaX within each bin of 'windowSize' points to approximate the derivatives. This fast approximation only works if the X is almost locally equally spaced.
The odd number of points to approximate the regression polynomial. Default 9
The grade of the derivative. 0 by default (Smoothing)
The order of the regression polynomial. Default 3
const { sgg } = require('ml-savitzky-golay-generalized');
const options = {
windowSize: 15,
derivative: 0,
polynomial: 3,
};
const noiseLevel = 0.1;
const data = new Array(200);
for (let i = 0; i < data.length; i++)
data[i] =
Math.sin((i * Math.PI * 2) / data.length) +
(Math.random() - 0.5) * noiseLevel;
const answer = sgg(data, (Math.PI * 2) / data.length, options);
console.log(answer);
const options = {
windowSize: 45,
derivative: 1,
polynomial: 3,
};
const noiseLevel = 0.1;
const data = new Array(200);
for (let i = 0; i < data.length; i++) {
data[i] =
Math.sin((i * Math.PI * 2) / data.length) +
(Math.random() - 0.5) * noiseLevel;
}
const answer = sgg(data, (Math.PI * 2) / data.length, options);
console.log(answer);
const options = {
windowSize: 47,
derivative: 1,
polynomial: 3,
};
const noiseLevel = 0.1;
const data = new Array(200);
const x = new Array(200);
for (let i = 0; i < data.length; i++) {
data[i] =
Math.sin((i * Math.PI * 2) / data.length) +
(Math.random() - 0.5) * noiseLevel;
x[i] = (i * Math.PI * 2) / data.length;
}
const ans = sgg(data, (Math.PI * 2) / data.length, options);
const ans2 = sgg(data, x, options);
FAQs
Savitzky–Golay filter in Javascript
The npm package ml-savitzky-golay-generalized receives a total of 2,084 weekly downloads. As such, ml-savitzky-golay-generalized popularity was classified as popular.
We found that ml-savitzky-golay-generalized demonstrated a healthy version release cadence and project activity because the last version was released less than a year ago. It has 5 open source maintainers collaborating on the project.
Did you know?
Socket for GitHub automatically highlights issues in each pull request and monitors the health of all your open source dependencies. Discover the contents of your packages and block harmful activity before you install or update your dependencies.
Research
Security News
Socket researchers uncover a malicious npm package posing as a tool for detecting vulnerabilities in Etherium smart contracts.
Security News
Research
A supply chain attack on Rspack's npm packages injected cryptomining malware, potentially impacting thousands of developers.
Research
Security News
Socket researchers discovered a malware campaign on npm delivering the Skuld infostealer via typosquatted packages, exposing sensitive data.