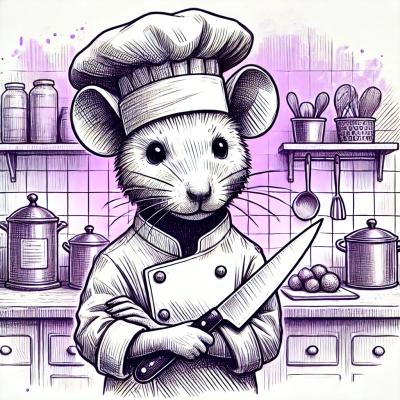
Research
Security News
Quasar RAT Disguised as an npm Package for Detecting Vulnerabilities in Ethereum Smart Contracts
Socket researchers uncover a malicious npm package posing as a tool for detecting vulnerabilities in Etherium smart contracts.
ml-spectra-fitting
Advanced tools
This is a spectra fitting package to optimize the position (x), max intensity (y), full width at half maximum (FWHM = width) and the ratio of gaussian contribution (mu) if it's required. It supports three kind of shapes:
Name | Equation |
---|---|
Gaussian | |
Lorentzian | |
Pseudo Voigt |
where
It is a wrapper of ml-levenberg-marquardt
$ npm install ml-spectra-fitting
import { optimize } from 'ml-spectra-fitting';
import { SpectrumGenerator } from 'spectrum-generator';
const generator = new SpectrumGenerator({
nbPoints: 101,
from: -1,
to: 1,
});
// by default the kind of shape is gaussian;
generator.addPeak({ x: 0.5, y: 0.2 }, { fwhm: 0.2 });
generator.addPeak(
{ x: -0.5, y: 0.2 },
{
shape: {
kind: 'lorentzian',
fwhm: 0.1,
},
},
);
//points to fit {x, y};
let data = generator.getSpectrum();
console.log(JSON.stringify({ x: Array.from(data.x), y: Array.from(data.y) }));
//the approximate values to be optimized, It could coming from a peak picking with ml-gsd
let peaks = [
{
x: -0.5,
y: 0.22,
shape: {
kind: 'gaussian',
fwhm: 0.25,
},
},
{
x: 0.52,
y: 0.18,
shape: {
kind: 'gaussian',
fwhm: 0.18,
},
},
];
// the function receive an array of peak with {x, y, fwhm} as a guess
// and return a list of objects
let fittedParams = optimize(data, peaks, { shape: { kind: 'pseudoVoigt' } });
console.log(fittedParams);
const result = {
error: 0.12361588652854476,
iterations: 100,
peaks: [
{
x: -0.5000014532421942,
y: 0.19995307937326137,
shape: {
kind: 'pseudoVoigt',
fwhm: 0.10007670374735196,
mu: 0.004731136777288483,
},
},
{
x: 0.5001051783652894,
y: 0.19960010175400406,
shape: {
kind: 'pseudoVoigt',
fwhm: 0.19935932346969124,
mu: 1,
},
},
],
};
For data with and combination of signals with shapes between gaussian and lorentzians, we could use the kind pseudovoigt to fit the data.
import { optimize } from 'ml-spectra-fitting';
import { SpectrumGenerator } from 'spectrum-generator';
const generator = new SpectrumGenerator({
nbPoints: 101,
from: -1,
to: 1,
});
// by default the kind of shape is gaussian;
generator.addPeak({ x: 0.5, y: 0.2 }, { fwhm: 0.2 });
generator.addPeak(
{ x: -0.5, y: 0.2 },
{
shape: {
kind: 'lorentzian',
fwhm: 0.1,
},
},
);
//points to fit {x, y};
let data = generator.getSpectrum();
console.log(JSON.stringify({ x: Array.from(data.x), y: Array.from(data.y) }));
//the approximate values to be optimized, It could coming from a peak picking with ml-gsd
let peaks = [
{
x: -0.5,
y: 0.22,
shape: {
kind: 'gaussian',
fwhm: 0.25,
},
},
{
x: 0.52,
y: 0.18,
shape: {
kind: 'gaussian',
fwhm: 0.18,
},
},
];
// the function receive an array of peak with {x, y, fwhm} as a guess
// and return a list of objects
let fittedParams = optimize(data, peaks, { shape: { kind: 'pseudoVoigt' } });
console.log(fittedParams);
const result = {
error: 0.12361588652854476,
iterations: 100,
peaks: [
{
x: -0.5000014532421942,
y: 0.19995307937326137,
shape: {
kind: 'pseudoVoigt',
fwhm: 0.10007670374735196,
mu: 0.004731136777288483,
},
},
{
x: 0.5001051783652894,
y: 0.19960010175400406,
shape: {
kind: 'pseudoVoigt',
fwhm: 0.19935932346969124,
mu: 1,
},
},
],
};
FAQs
Fit spectra using gaussian or lorentzian
The npm package ml-spectra-fitting receives a total of 150 weekly downloads. As such, ml-spectra-fitting popularity was classified as not popular.
We found that ml-spectra-fitting demonstrated a healthy version release cadence and project activity because the last version was released less than a year ago. It has 0 open source maintainers collaborating on the project.
Did you know?
Socket for GitHub automatically highlights issues in each pull request and monitors the health of all your open source dependencies. Discover the contents of your packages and block harmful activity before you install or update your dependencies.
Research
Security News
Socket researchers uncover a malicious npm package posing as a tool for detecting vulnerabilities in Etherium smart contracts.
Security News
Research
A supply chain attack on Rspack's npm packages injected cryptomining malware, potentially impacting thousands of developers.
Research
Security News
Socket researchers discovered a malware campaign on npm delivering the Skuld infostealer via typosquatted packages, exposing sensitive data.