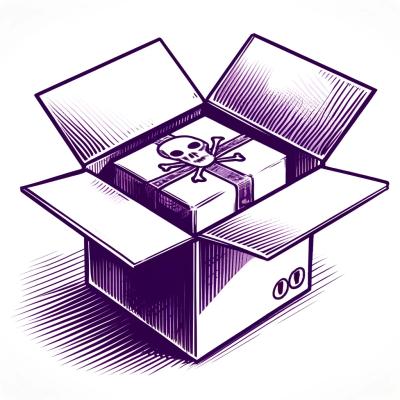
Security News
Research
Data Theft Repackaged: A Case Study in Malicious Wrapper Packages on npm
The Socket Research Team breaks down a malicious wrapper package that uses obfuscation to harvest credentials and exfiltrate sensitive data.
Unlock the power of AutoViz to visualize any dataset, any size, with just a single line of code! Plus, now you can get a quick assessment of your dataset's quality and fix DQ issues through the FixDQ() function.
With AutoViz, you can easily and quickly generate insightful visualizations for your data. Whether you're a beginner or an expert in data analysis, AutoViz can help you explore your data and uncover valuable insights. Try it out and see the power of automated visualization for yourself!
The latest updates about autoviz
library can be found in Updates page.
Your feedback is crucial! If you encounter any issues or have suggestions, please let us know through GitHub Issues
Thank you for your continued support and happy visualizing!
If you use AutoViz in your research project or paper, please use the following format for citations:
"Seshadri, Ram (2020). GitHub - AutoViML/AutoViz: Automatically Visualize any dataset, any size with a single line of code. source code: https://github.com/AutoViML/AutoViz"
Current citations for AutoVizThe motivation behind the creation of AutoViz is to provide a more efficient, user-friendly, and automated approach to exploratory data analysis (EDA) through quick and easy data visualization plus data quality. The library is designed to help users understand patterns, trends, and relationships in the data by creating insightful visualizations with minimal effort. AutoViz is particularly useful for beginners in data analysis as it abstracts away the complexities of various plotting libraries and techniques. For experts, it provides another expert tool that they can use to provide inights into data that they may have missed.
AutoViz is a powerful tool for generating insightful visualizations with minimal effort. Here are some of its key selling points compared to other automated EDA tools:
Prerequisites
Create a new environment and install the required dependencies to clone AutoViz:
From PyPi:
cd <AutoViz_Destination>
git clone git@github.com:AutoViML/AutoViz.git
# or download and unzip https://github.com/AutoViML/AutoViz/archive/master.zip
conda create -n <your_env_name> python=3.7 anaconda
conda activate <your_env_name> # ON WINDOWS: `source activate <your_env_name>`
cd AutoViz
For Python versions below 3.10, install dependencies as follows:
pip install -r requirements.txt
For Python 3.10, please use:
pip install -r requirements-py310.txt
For Python 3.11 and above, it's recommended to use:
pip install -r requirements-py311.txt
These requirement files ensure that AutoViz works seamlessly with your Python environment by installing compatible versions of libraries like HoloViews, Bokeh, and hvPlot. Please select the requirement file that corresponds to your Python version to enjoy a smooth experience with AutoViz.
Discover how to use AutoViz in this Medium article.
In the AutoViz directory, open a Jupyter Notebook or open a command palette (terminal) and use the following code to instantiate the AutoViz_Class. You can simply run this code step by step:
from autoviz import AutoViz_Class
AV = AutoViz_Class()
dft = AV.AutoViz(filename)
AutoViz can use any input either filename (in CSV, txt, or JSON format) or a pandas dataframe. If you have a large dataset, you can set the max_rows_analyzed
and max_cols_analyzed
arguments to speed up the visualization by asking autoviz to sample your dataset.
AutoViz can also create charts in multiple formats using the chart_format
setting:
chart_format ='png'
or 'svg'
or 'jpg'
: Matplotlib charts are plotted inline.
verbose=2
setting) or displayed (verbose=1
) in Jupyter Notebooks.chart_format='bokeh'
: Interactive Bokeh charts are plotted in Jupyter Notebooks.chart_format='server'
, dashboards will pop up for each kind of chart on your browser.chart_format='html'
, interactive Bokeh charts will be created and silently saved as HTML files under the AutoViz_Plots
directory (under working folder) or any other directory that you specify using the save_plot_dir
setting (during input).Arguments for AV.AutoViz()
method:
filename
: Use an empty string ("") if there's no associated filename and you want to use a dataframe. In that case, using the dfte
argument for the dataframe. Otherwise provide a filename and leave dfte
argument with an empty string. Only one of them can be used.sep
: File separator (comma, semi-colon, tab, or any column-separating value) if you use a filename above.depVar
: Target variable in your dataset; set it as an empty string if not applicable.dfte
: name of the pandas dataframe for plotting charts; leave it as empty string if using a filename.header
: set the row number of the header row in your file (0 for the first row). Otherwise leave it as 0.verbose
: 0 for minimal info and charts, 1 for more info and charts, or 2 for saving charts locally without display.lowess
: Use regression lines for each pair of continuous variables against the target variable in small datasets; avoid using for large datasets (>100,000 rows).chart_format
: 'svg', 'png', 'jpg', 'bokeh', 'server', or 'html' for displaying or saving charts in various formats, depending on the verbose option.max_rows_analyzed
: Limit the max number of rows to use for visualization when dealing with very large datasets (millions of rows). A statistically valid sample will be used by autoviz. Default is 150000 rows.max_cols_analyzed
: Limit the number of continuous variables to be analyzed. Defaul is 30 columns.save_plot_dir
: Directory for saving plots. Default is None, which saves plots under the current directory in a subfolder named AutoViz_Plots. If the save_plot_dir doesn't exist, it will be created.Here are some examples to help you get started with AutoViz. If you need full jupyter notebooks with code samples they can be found in examples folder.
from autoviz import AutoViz_Class
AV = AutoViz_Class()
filename = "your_file.csv"
target_variable = "your_target_variable"
dft = AV.AutoViz(
filename,
sep=",",
depVar=target_variable,
dfte=None,
header=0,
verbose=1,
lowess=False,
chart_format="svg",
max_rows_analyzed=150000,
max_cols_analyzed=30,
save_plot_dir=None
)
import pandas as pd
from autoviz import AutoViz_Class
AV = AutoViz_Class()
data = {'col1': [1, 2, 3, 4, 5], 'col2': [5, 4, 3, 2, 1]}
df = pd.DataFrame(data)
dft = AV.AutoViz(
"",
sep=",",
depVar="",
dfte=df,
header=0,
verbose=1,
lowess=False,
chart_format="server",
max_rows_analyzed=150000,
max_cols_analyzed=30,
save_plot_dir=None
)
from autoviz import AutoViz_Class
AV = AutoViz_Class()
filename = "your_file.csv"
target_variable = "your_target_variable"
custom_plot_dir = "your_custom_plot_directory"
dft = AV.AutoViz(
filename,
sep=",",
depVar=target_variable,
dfte=None,
header=0,
verbose=2,
lowess=False,
chart_format="bokeh",
max_rows_analyzed=150000,
max_cols_analyzed=30,
save_plot_dir=custom_plot_dir
)
These examples should give you an idea of how to use AutoViz with different scenarios and settings. By tailoring the options and settings, you can generate visualizations that best suit your needs, whether you're working with large datasets, interactive charts, or simply exploring the relationships between variables.
AutoViz is actively maintained and improved by a team of dedicated developers. If you have any questions, suggestions, or issues, feel free to reach out to the maintainers:
We welcome contributions from the community! If you're interested in contributing to AutoViz, please follow these steps:
AutoViz is released under the Apache License, Version 2.0. By using AutoViz, you agree to the terms and conditions specified in the license.
Here are some additional tips and reminders to help you make the most of the library:
This project is not an official Google project. It is not supported by Google, and Google specifically disclaims all warranties as to its quality, merchantability, or fitness for a particular purpose.
FAQs
Automatically Visualize any dataset, any size with a single line of code
We found that autoviz demonstrated a healthy version release cadence and project activity because the last version was released less than a year ago. It has 2 open source maintainers collaborating on the project.
Did you know?
Socket for GitHub automatically highlights issues in each pull request and monitors the health of all your open source dependencies. Discover the contents of your packages and block harmful activity before you install or update your dependencies.
Security News
Research
The Socket Research Team breaks down a malicious wrapper package that uses obfuscation to harvest credentials and exfiltrate sensitive data.
Research
Security News
Attackers used a malicious npm package typosquatting a popular ESLint plugin to steal sensitive data, execute commands, and exploit developer systems.
Security News
The Ultralytics' PyPI Package was compromised four times in one weekend through GitHub Actions cache poisoning and failure to rotate previously compromised API tokens.