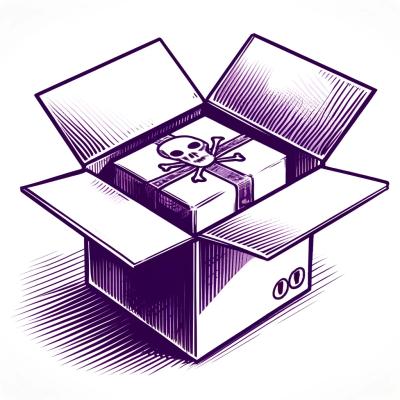
Security News
Research
Data Theft Repackaged: A Case Study in Malicious Wrapper Packages on npm
The Socket Research Team breaks down a malicious wrapper package that uses obfuscation to harvest credentials and exfiltrate sensitive data.
This project started as a POC that aimed to showcase the wonders that could be done if BigQuery provided a DataFrame API in Python similar to the one already available with PySpark or Snowpark. With time, I started to add more and more cool features :sunglasses:.
I tried to reproduce the most commonly used methods of the Spark DataFrame object. I aimed at making something as close as possible as PySpark, and tried to keep exactly the same naming and docstrings as PySpark's DataFrames.
For instance, this is a working example of PySpark code:
from pyspark.sql import SparkSession
from pyspark.sql import functions as f
spark = SparkSession.builder.master("local[1]").getOrCreate()
df = spark.sql("""
SELECT 1 as id, "Bulbasaur" as name, ARRAY("Grass", "Poison") as types, NULL as other_col
UNION ALL
SELECT 2 as id, "Ivysaur" as name, ARRAY("Grass", "Poison") as types, NULL as other_col
""")
df.select("id", "name", "types").createOrReplaceTempView("pokedex")
df2 = spark.sql("""SELECT * FROM pokedex""")\
.withColumn("nb_types", f.expr("SIZE(types)"))\
.withColumn("name", f.expr("LOWER(name)"))
df2.show()
# +---+---------+---------------+--------+
# | id| name| types|nb_types|
# +---+---------+---------------+--------+
# | 1|bulbasaur|[Grass, Poison]| 2|
# | 2| ivysaur|[Grass, Poison]| 2|
# +---+---------+---------------+--------+
And this is an equivalent working example using bigquery_frame, that runs on Google Big Query!
from bigquery_frame import BigQueryBuilder
from bigquery_frame.auth import get_bq_client
from bigquery_frame import functions as f
bigquery = BigQueryBuilder(get_bq_client())
df = bigquery.sql("""
SELECT 1 as id, "Bulbasaur" as name, ["Grass", "Poison"] as types, NULL as other_col
UNION ALL
SELECT 2 as id, "Ivysaur" as name, ["Grass", "Poison"] as types, NULL as other_col
""")
df.select("id", "name", "types").createOrReplaceTempView("pokedex")
df2 = bigquery.sql("""SELECT * FROM pokedex""")\
.withColumn("nb_types", f.expr("ARRAY_LENGTH(types)"))\
.withColumn("name", f.expr("LOWER(name)"), replace=True)
df2.show()
# +----+-----------+---------------------+----------+
# | id | name | types | nb_types |
# +----+-----------+---------------------+----------+
# | 1 | bulbasaur | ['Grass', 'Poison'] | 2 |
# | 2 | ivysaur | ['Grass', 'Poison'] | 2 |
# +----+-----------+---------------------+----------+
I believe that DataFrames are super cool to organise SQL code as it allows us to several things that are much harder, or even impossible, in pure-SQL:
But that deserves a blog article.
bigquery-frame is available on PyPi.
pip install bigquery-frame
There are three possible methods detailed in AUTH.md:
Either run gcloud auth application-default login
and set the environment variable GCP_PROJECT
to your project name.
If you do that bigquery-frame will inherit use own credentials.
Create a service account, configure its rights and set the environment variable GCP_CREDENTIALS_PATH
to point to
your service account's credential file.
Create a google.cloud.bigquery.Client
object yourself and pass it to the BigQueryBuilder
.
from bigquery_frame import BigQueryBuilder
bq = BigQueryBuilder()
df = bq.table('bigquery-public-data.utility_us.country_code_iso')
df.printSchema()
df.show()
Very simply, by generating SQL queries that are sent to BigQuery.
You can get the query by calling the method DataFrame.compile()
.
For instance, if we reuse the example from the beginning:
print(df2.compile())
This will print the following SQL query:
WITH pokedex AS (
WITH _default_alias_1 AS (
SELECT 1 as id, "Bulbasaur" as name, ["Grass", "Poison"] as types, NULL as other_col
UNION ALL
SELECT 2 as id, "Ivysaur" as name, ["Grass", "Poison"] as types, NULL as other_col
)
SELECT
id,
name,
types
FROM _default_alias_1
)
, _default_alias_3 AS (
SELECT * FROM pokedex
)
, _default_alias_4 AS (
SELECT
*,
ARRAY_LENGTH(types) AS nb_types
FROM _default_alias_3
)
SELECT
* REPLACE (
LOWER(name) AS name
)
FROM _default_alias_4
This feature list is arbitrarily sorted by decreasing order of coolness.
Just like git diff, but for data!
Performs a diff between two dataframes.
It can be called from Python or directly via the command line if you made a pip install bigquery-frame
.
Example with the command line:
Please make sure you followed the Installation section first.
In this example, I compared two snapshots of the public table bigquery-public-data.utility_us.country_code_iso
made at 6 days interval and noticed that a bug had been introduced, as you can see from the diff: the new values
for the columns continent_code
and continent_name
look like they have been inverted.
$ bq-diff --tables test_us.country_code_iso_snapshot_20220921 test_us.country_code_iso_snapshot_20220927 --join-cols country_name
Analyzing differences...
We will try to find the differences by joining the DataFrames together using the provided column: country_name
100%|███████████████████████████████████████████████████████████████████████████████████| 1/1 [00:04<00:00, 4.66s/it]
Schema: ok (10)
diff NOT ok
Summary:
Row count ok: 278 rows
28 (10.07%) rows are identical
250 (89.93%) rows have changed
100%|███████████████████████████████████████████████████████████████████████████████████| 1/1 [00:04<00:00, 4.67s/it]
Found the following differences:
+----------------+---------------+---------------+--------------------+----------------+
| column_name | total_nb_diff | left | right | nb_differences |
+----------------+---------------+---------------+--------------------+----------------+
| continent_code | 250 | NA | Caribbean | 26 |
| continent_code | 250 | AF | Eastern Africa | 20 |
| continent_code | 250 | AS | Western Asia | 18 |
| continent_code | 250 | AF | Western Africa | 17 |
| continent_code | 250 | EU | Southern Europe | 16 |
| continent_code | 250 | EU | Northern Europe | 15 |
| continent_code | 250 | SA | South America | 14 |
| continent_code | 250 | AS | South-Eastern Asia | 11 |
| continent_code | 250 | EU | Eastern Europe | 10 |
| continent_code | 250 | OC | Polynesia | 10 |
| continent_name | 250 | Africa | AF | 58 |
| continent_name | 250 | Asia | AS | 54 |
| continent_name | 250 | Europe | EU | 53 |
| continent_name | 250 | North America | NA | 39 |
| continent_name | 250 | Oceania | OC | 26 |
| continent_name | 250 | South America | SA | 15 |
| continent_name | 250 | Antarctica | AN | 5 |
+----------------+---------------+---------------+--------------------+----------------+
An equivalent analysis made with Python is available at examples/data_diff/country_code_iso.py.
Example with Python:
Please make sure you followed the Installation section first.
from bigquery_frame.data_diff import DataframeComparator
from bigquery_frame import BigQueryBuilder
from bigquery_frame.auth import get_bq_client
bq = BigQueryBuilder(get_bq_client())
df1 = bq.sql("""
SELECT * FROM UNNEST ([
STRUCT(1 as id, [STRUCT(1 as a, 2 as b, 3 as c)] as my_array),
STRUCT(2 as id, [STRUCT(1 as a, 2 as b, 3 as c)] as my_array),
STRUCT(3 as id, [STRUCT(1 as a, 2 as b, 3 as c)] as my_array)
])
""")
df2 = bq.sql("""
SELECT * FROM UNNEST ([
STRUCT(1 as id, [STRUCT(1 as a, 2 as b, 3 as c, 4 as d)] as my_array),
STRUCT(2 as id, [STRUCT(2 as a, 2 as b, 3 as c, 4 as d)] as my_array),
STRUCT(4 as id, [STRUCT(1 as a, 2 as b, 3 as c, 4 as d)] as my_array)
])
""")
df1.show()
# +----+----------------------------+
# | id | my_array |
# +----+----------------------------+
# | 1 | [{'a': 1, 'b': 2, 'c': 3}] |
# | 2 | [{'a': 1, 'b': 2, 'c': 3}] |
# | 3 | [{'a': 1, 'b': 2, 'c': 3}] |
# +----+----------------------------+
df2.show()
# +----+------------------------------------+
# | id | my_array |
# +----+------------------------------------+
# | 1 | [{'a': 1, 'b': 2, 'c': 3, 'd': 4}] |
# | 2 | [{'a': 2, 'b': 2, 'c': 3, 'd': 4}] |
# | 4 | [{'a': 1, 'b': 2, 'c': 3, 'd': 4}] |
# +----+------------------------------------+
diff_result = DataframeComparator().compare_df(df1, df2)
diff_result.display()
Will produce the following output:
Schema has changed:
@@ -2,3 +2,4 @@
my_array!.a INTEGER
my_array!.b INTEGER
my_array!.c INTEGER
+my_array!.d INTEGER
WARNING: columns that do not match both sides will be ignored
diff NOT ok
Summary:
Row count ok: 3 rows
1 (25.0%) rows are identical
1 (25.0%) rows have changed
1 (25.0%) rows are only in 'left'
1 (25.0%) rows are only in 'right
100%|██████████| 1/1 [00:04<00:00, 4.26s/it]
Found the following differences:
+-------------+---------------+-----------------------+-----------------------+----------------+
| column_name | total_nb_diff | left | right | nb_differences |
+-------------+---------------+-----------------------+-----------------------+----------------+
| my_array | 1 | [{"a":1,"b":2,"c":3}] | [{"a":2,"b":2,"c":3}] | 1 |
+-------------+---------------+-----------------------+-----------------------+----------------+
1 rows were only found in 'left' :
Analyzing 4 columns ...
+---------------+-------------+-------------+-------+----------------+------------+-----+-----+------------------------------+
| column_number | column_name | column_type | count | count_distinct | count_null | min | max | approx_top_100 |
+---------------+-------------+-------------+-------+----------------+------------+-----+-----+------------------------------+
| 0 | id | INTEGER | 1 | 1 | 0 | 3 | 3 | [{'value': '3', 'count': 1}] |
| 1 | my_array!.a | INTEGER | 1 | 1 | 0 | 1 | 1 | [{'value': '1', 'count': 1}] |
| 2 | my_array!.b | INTEGER | 1 | 1 | 0 | 2 | 2 | [{'value': '2', 'count': 1}] |
| 3 | my_array!.c | INTEGER | 1 | 1 | 0 | 3 | 3 | [{'value': '3', 'count': 1}] |
+---------------+-------------+-------------+-------+----------------+------------+-----+-----+------------------------------+
1 rows were only found in 'right':
Analyzing 4 columns ...
+---------------+-------------+-------------+-------+----------------+------------+-----+-----+------------------------------+
| column_number | column_name | column_type | count | count_distinct | count_null | min | max | approx_top_100 |
+---------------+-------------+-------------+-------+----------------+------------+-----+-----+------------------------------+
| 0 | id | INTEGER | 1 | 1 | 0 | 4 | 4 | [{'value': '4', 'count': 1}] |
| 1 | my_array!.a | INTEGER | 1 | 1 | 0 | 1 | 1 | [{'value': '1', 'count': 1}] |
| 2 | my_array!.b | INTEGER | 1 | 1 | 0 | 2 | 2 | [{'value': '2', 'count': 1}] |
| 3 | my_array!.c | INTEGER | 1 | 1 | 0 | 3 | 3 | [{'value': '3', 'count': 1}] |
+---------------+-------------+-------------+-------+----------------+------------+-----+-----+------------------------------+
Perform an analysis on a DataFrame, return aggregated stats for each column, such as count, count distinct, count null, min, max, top 100 most frequent values.
Example:
from bigquery_frame.transformations_impl.analyze import __get_test_df
from bigquery_frame.transformations import analyze
df = __get_test_df()
df.show()
# +----+------------+---------------------+--------------------------------------------+
# | id | name | types | evolution |
# +----+------------+---------------------+--------------------------------------------+
# | 1 | Bulbasaur | ['Grass', 'Poison'] | {'can_evolve': True, 'evolves_from': None} |
# | 2 | Ivysaur | ['Grass', 'Poison'] | {'can_evolve': True, 'evolves_from': 1} |
# | 3 | Venusaur | ['Grass', 'Poison'] | {'can_evolve': False, 'evolves_from': 2} |
# | 4 | Charmander | ['Fire'] | {'can_evolve': True, 'evolves_from': None} |
# | 5 | Charmeleon | ['Fire'] | {'can_evolve': True, 'evolves_from': 4} |
# | 6 | Charizard | ['Fire', 'Flying'] | {'can_evolve': False, 'evolves_from': 5} |
# | 7 | Squirtle | ['Water'] | {'can_evolve': True, 'evolves_from': None} |
# | 8 | Wartortle | ['Water'] | {'can_evolve': True, 'evolves_from': 7} |
# | 9 | Blastoise | ['Water'] | {'can_evolve': False, 'evolves_from': 8} |
# +----+------------+---------------------+--------------------------------------------+
analyze(df).show()
# +---------------+------------------------+-------------+-------+----------------+------------+-----------+-----------+------------------------------------------------------------------------------------------------------------------------------------------------------------------------------------------------------------------------------------------------------------------------------------------------------------------------------------+
# | column_number | column_name | column_type | count | count_distinct | count_null | min | max | approx_top_100 |
# +---------------+------------------------+-------------+-------+----------------+------------+-----------+-----------+------------------------------------------------------------------------------------------------------------------------------------------------------------------------------------------------------------------------------------------------------------------------------------------------------------------------------------+
# | 0 | id | INTEGER | 9 | 9 | 0 | 1 | 9 | [{'value': '1', 'count': 1}, {'value': '2', 'count': 1}, {'value': '3', 'count': 1}, {'value': '4', 'count': 1}, {'value': '5', 'count': 1}, {'value': '6', 'count': 1}, {'value': '7', 'count': 1}, {'value': '8', 'count': 1}, {'value': '9', 'count': 1}] |
# | 1 | name | STRING | 9 | 9 | 0 | Blastoise | Wartortle | [{'value': 'Bulbasaur', 'count': 1}, {'value': 'Ivysaur', 'count': 1}, {'value': 'Venusaur', 'count': 1}, {'value': 'Charmander', 'count': 1}, {'value': 'Charmeleon', 'count': 1}, {'value': 'Charizard', 'count': 1}, {'value': 'Squirtle', 'count': 1}, {'value': 'Wartortle', 'count': 1}, {'value': 'Blastoise', 'count': 1}] |
# | 2 | types! | STRING | 13 | 5 | 0 | Fire | Water | [{'value': 'Grass', 'count': 3}, {'value': 'Poison', 'count': 3}, {'value': 'Fire', 'count': 3}, {'value': 'Water', 'count': 3}, {'value': 'Flying', 'count': 1}] |
# | 3 | evolution.can_evolve | BOOLEAN | 9 | 2 | 0 | false | true | [{'value': 'true', 'count': 6}, {'value': 'false', 'count': 3}] |
# | 4 | evolution.evolves_from | INTEGER | 9 | 6 | 3 | 1 | 8 | [{'value': 'NULL', 'count': 3}, {'value': '1', 'count': 1}, {'value': '2', 'count': 1}, {'value': '4', 'count': 1}, {'value': '5', 'count': 1}, {'value': '7', 'count': 1}, {'value': '8', 'count': 1}] |
# +---------------+------------------------+-------------+-------+----------------+------------+-----------+-----------+------------------------------------------------------------------------------------------------------------------------------------------------------------------------------------------------------------------------------------------------------------------------------------------------------------------------------------+
Example with custom aggregation and column groups:
from bigquery_frame.transformations_impl import analyze_aggs
from bigquery_frame.transformations import analyze
aggs = [
analyze_aggs.column_number,
analyze_aggs.column_name,
analyze_aggs.count,
analyze_aggs.count_distinct,
analyze_aggs.count_null,
]
analyze(df, group_by="evolution.can_evolve", _aggs=aggs).orderBy('group.can_evolve', 'column_number').show()
# +-----------------------+---------------+------------------------+-------+----------------+------------+
# | group | column_number | column_name | count | count_distinct | count_null |
# +-----------------------+---------------+------------------------+-------+----------------+------------+
# | {'can_evolve': False} | 0 | id | 3 | 3 | 0 |
# | {'can_evolve': False} | 1 | name | 3 | 3 | 0 |
# | {'can_evolve': False} | 2 | types! | 5 | 5 | 0 |
# | {'can_evolve': False} | 4 | evolution.evolves_from | 3 | 3 | 0 |
# | {'can_evolve': True} | 0 | id | 6 | 6 | 0 |
# | {'can_evolve': True} | 1 | name | 6 | 6 | 0 |
# | {'can_evolve': True} | 2 | types! | 8 | 4 | 0 |
# | {'can_evolve': True} | 4 | evolution.evolves_from | 6 | 3 | 3 |
# +-----------------------+---------------+------------------------+-------+----------------+------------+
All queries are run on BigQuery, so BigQuery usual billing will apply on your queries. The following operations trigger a query execution:
df.show()
df.persist()
df.createOrReplaceTempTable()
df.write
The operation df.schema
also triggers a query execution, but it uses a LIMIT 0
, which will make BigQuery return
0 rows and charge 0 cent for it.
Since version 0.4.0, the queries generated by bigquery-frame are now 100% deterministic, so executing the same DataFrame code twice should send the same SQL query to BigQuery, thus leveraging BigQuery's query caching.
Since version 0.4.2, the BigQueryBuilder gather statistics about billing.
Example:
from bigquery_frame import BigQueryBuilder
bq = BigQueryBuilder()
df = bq.sql(f"SELECT 1 as a").persist()
df.show()
print(bq.stats.human_readable())
will print:
+---+
| a |
+---+
| 1 |
+---+
Estimated bytes processed : 8.00 B
Total bytes processed : 8.00 B
Total bytes billed : 10.00 MiB
The number of bytes billed is larger because BigQuery applies a minimal pricing on all queries run
to account for execution overhead (except those with LIMIT 0
).
More details in the BigQuery Documentation.
Some advanced transformations, like analyze
and DataframeComparator.compare_df
, persist intermediary results.
analyze
scans each column of the table exactly once, and stores very small intermediary results, which should
cost no more than 10 MiB per 50 column in the table analyzed. This is negligible and the cost of analyze
should be comparable to that of a regular SELECT *
.
DataframeComparator.compare_df
persists multiple intermediary results, which should never exceed 2 times the size
of the input tables. It also has a built-in security to prevent any combinatorial explosion, should the user provide
a join key that has duplicate. Overall, the cost of a diff should be comparable to scanning both input tables
4 to 6 times.
If you have already used Spark, you probably remember times when you tried to troubleshoot a complex transformation pipeline but had trouble finding at which exact line the bug was hidden, because Spark's lazy evaluation often makes it crash several steps after the actual buggy line.
To make debugging easier, I added a debug
flag that when set to True, will validate each transformation
step instead of doing a lazy evaluation at the end.
Examples:
from bigquery_frame import BigQueryBuilder
bq = BigQueryBuilder(client)
df1 = bq.sql("""SELECT 1 as a""")
# This line is wrong, but since we don't fetch any result here, the error will be caught later.
df2 = df1.select("b")
# The execution fails at this line.
df2.show()
from bigquery_frame import BigQueryBuilder
bq = BigQueryBuilder(client)
# This time, we activate the "debug mode"
bq.debug = True
df1 = bq.sql("""SELECT 1 as a""")
# Since debug mode is enabled, the execution will fail at this line.
df2 = df1.select("b")
df2.show()
Of course, this is costly in performance, even if query validation does not
incur extra query costs, and it is advised to keep this
option to False
by default and use it only for troubleshooting.
This project started as a POC, and while I keep improving it with time, I did take some shortcuts and did not try to optimize the query length. In particular, bigquery-frame generates a lot of CTEs, and any serious project trying to use it might reach the maximum query length very quickly.
Here is a list of other known limitations, please also see the Further developments section for a list of missing features.
DataFrame.withColumn
:
replace=True
must be passed.DataFrame.createOrReplaceTempView
:
DataFrame.join
:
Functions not supported yet:
DataFrame.groupBy
explode
, explode_outer
, etc.Other improvements:
BigQueryBuilder.sql
, functions.expr
).Also, it would be cool to expand this to other SQL engines than BigQuery (contributors are welcome :wink: ).
ibis-project provides a Python API inspired by pandas and dplyr that generates queries against multiple analytics (SQL or DataFrame-based) backends, such as Pandas, Dask, PySpark and BigQuery.
I played with ibis very shortly, so I might have misunderstood some parts, but from my understanding, I noted the following differences between bigquery-frame and ibis-bigquery:
Unlike bigquery-frame (at least for now), Ibis is always schema-conscious which has several upsides:
But this also have some downsides:
Another key difference between ibis-bigquery and bigquery-frame is that ibis-bigquery is part of a larger
cross-platform framework, while bigquery-frame only support BigQuery at the moment. This allows bigquery-frame
to support more easily some of BigQuery's exotic features. For instance bigquery-frame offers a good
support for nested repeated fields (array of structs) thanks to the flatten_schema
function,
and the functions.transform
, DataFrame.with_nested_columns
and DataFrame.select_nested_columns
functions.
I plan to address most of bigquery-frame's downsides in the future, whenever I get the time.
I hope that it will motivate the teams working on BigQuery (or Redshift, or Azure Synapse) to propose a real python DataFrame API on top of their massively parallel SQL engines. But not something that generates ugly SQL strings, more something like Spark Catalyst, which directly generates logical plans out of the DataFrame API without passing through the "SQL string" step.
After starting this POC, I realized Snowflake already understood this and developed Snowpark, a Java/Scala (and soon Python) API to run complex workflows on Snowflake, and Snowpark's DataFrame API which was clearly borrowed from Spark's DataFrame (= DataSet[Row]) API (we recognize several key method names: cache, createOrReplaceTempView, where/filter, collect, toLocalIterator).
I believe such project could open the gate to hundreds of very cool applications. For instance, did you know that, in its early versions at least, Dataiku Shaker was just a GUI that chained transformations on Pandas DataFrame, and later Spark DataFrame ?
Another possible evolution would be to make a DataFrame API capable of speaking multiple SQL dialects. By using it, projects that generate SQL for multiple platforms, like Malloy, could all use the same DataFrame abstraction. Adding support for a new SQL platform would immediately allow all the project based on it to support this new platform.
I recently discovered several other projects that have similar goals:
Given that the ibis-project is much more advanced than mine when it comes to multilingualism, I think I will pursue my efforts into deep-diving more advanced features for BigQuery only. Perhaps one day I or someone else will port them to the ibis-project.
Would anyone be interested in trying to POC an extension of this project to RedShift, Postgres, Azure Synapse, or any other SQL engines SQL engine, I would be very glad to discuss it.
Improvements:
DataFrame.groupBy
. It also supports DataFrame.groupBy(...).pivot(...)
.transformations.pivot
now support doing multiple aggregations at the same time.
It's signature has changed: The arguments agg_fun
and agg_col
have been replaced with aggs
and the argument implem_version
has been removed: The first implementation version has been removed.DataFrame.show_string
that returns the string resulting from DataFrame.show
without printing itDataFrame.transform
that provides a concise syntax for chaining custom transformations.functions.array_agg
now support passing multiple columns in the order_by argument.functions
:
avg
and mean
lower
and upper
regexp_replace
explode
, explode_outer
, posexplode_outer
and posexplode_outer
functions.lit
can now generate most BigQuery types:
Column.isin
.functions.sort_array
and functions.transform
, they can now be correctly used together.
But their signature has changed. They now take functions as arguments instead of strings."s!.id"
in join_cols will now explode the
corresponding array and perform the diff on it).join_cols
does not exist.Breaking changes:
DataFrame.join
does not supports string expression. For instance df1.join(df2, on="col1 == col2")
must be rewritten df1.join(df2, on=df1["col1"] == df2["col2"])
transformations.pivot
method has changed:
The arguments agg_fun
and agg_col
have been replaced with aggs
and the argument implem_version
has been removed: The first implementation version has been removed.functions.sort_array
method has changed.
The second argument sort_cols: Union[Column, List[Column]]
was replaced
with sort_keys: Optional[Callable[[Column], Union[Column, List[Column]]]] = None
.functions.transform
method has changed.
The second argument transform_col: Column
was replaced with func: Callable[[Column], Column]
bigquery_frame.data_diff.DataframeComparator
object has been removed.
Please use directly the method bigquery_frame.data_diff.compare_dataframes
.bigquery_frame.data_diff.diff_results
has been renamed to diff_result
.Bugfixes:
transformations.unpivot
now works with an empty pivot_columns
list.flatten_schema
method has been moved from transformations
to data_type_utils
.functions.array_agg
method.graph.connected_component
computes the connected components in a graph using the
"small star - large star" algorithm which is conjectured to perform in O(n log(n))
.functions.from_base_32
and functions.from_base_64
.print(bq.stats.human_readable())
BigQueryBuilder.debug
attribute. When set to true, each step of a DataFrame
transformation flow will be validated instead of compiling the query lazily when we need to fetch a result.bq-diff
command that can be called directly from bash. Run bq-diff --help
for more information.DataFrame.write
feature. It currently supports partitioning, table-level options, and several insertion modes:
append
: Append contents of this :class:DataFrame
to existing table.overwrite
: Replace destination table with the new data if it already exists.error
or errorifexists
: Throw an exception if destination table already exists.ignore
: Silently ignore this operation if destination table already exists.BigQueryBuilder
now tries to create its own BigQuery client if none is passedSeveral new features that make working with nested structure easier were added.
DataFrame.select_nested_columns
and DataFrame.with_nested_columns
, which
make transformation of nested values much easierfunctions.transform
, useful to transform arraystransformations.normalize_arrays
which automatically sort all arrays in a DataFrame, including
arrays of arrays of arrays...transformations.harmonize_dataframes
which takes two DataFrames and transform them into DataFrames
with the same schema.functions.to_base32
and functions.to_base64
.
from_base_32
and from_base_64
will be added later,
once a bug in python-tabulate is fixed.Column.__mod__
(e.g. f.when(c % 2 == 0)
)Column.when.otherwise
. Now functions.when
returns a WhenColumn
, a
special type of Column
with two extra methods: when
and otherwise
.functions.sort_array
's signature to make it consistent with transform
Column[...]
(__getitem
) that can be used to access struct or array elements.transformations.analyze
bigquery_frame.transformations.analyze
now return one extra column named column_number
asc
, desc
replace
, array
,Dataframe.sort
now works on aliased columnsColumn.alias
now works on sql keywordsfunctions.eqNullSafe
now never returns NULLColumn
constructor no longer accept alias
as argument. Use Column.alias()
instead.cast
, ~
, isNull
, isNotNull
, eqNullSafe
concat
, length
, sort_array
, substring
Column.when().otherwise().alias()
functions.cast()
methodDataFrame.join()
. This is a first implementation which is a little clumsy.df.alias()
FAQs
A DataFrame API for Google BigQuery
We found that bigquery-frame demonstrated a healthy version release cadence and project activity because the last version was released less than a year ago. It has 1 open source maintainer collaborating on the project.
Did you know?
Socket for GitHub automatically highlights issues in each pull request and monitors the health of all your open source dependencies. Discover the contents of your packages and block harmful activity before you install or update your dependencies.
Security News
Research
The Socket Research Team breaks down a malicious wrapper package that uses obfuscation to harvest credentials and exfiltrate sensitive data.
Research
Security News
Attackers used a malicious npm package typosquatting a popular ESLint plugin to steal sensitive data, execute commands, and exploit developer systems.
Security News
The Ultralytics' PyPI Package was compromised four times in one weekend through GitHub Actions cache poisoning and failure to rotate previously compromised API tokens.