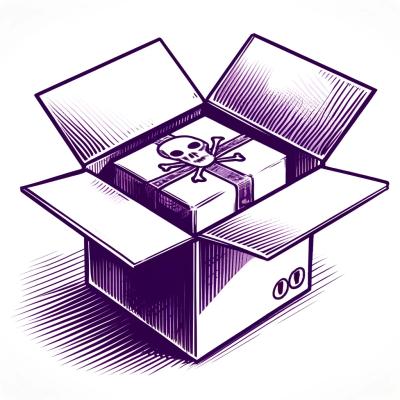
Security News
Research
Data Theft Repackaged: A Case Study in Malicious Wrapper Packages on npm
The Socket Research Team breaks down a malicious wrapper package that uses obfuscation to harvest credentials and exfiltrate sensitive data.
Key Features | Latest Updates | Vision | Quick Start | Reference docs | License
EasyDeL is an open-source framework designed to enhance and streamline the training process of machine learning models, with a primary focus on Jax/Flax. It provides convenient and effective solutions for training and serving Flax/Jax models on TPU/GPU at scale.
EasyDeL stands out by providing unparalleled flexibility and transparency:
Open Architecture: Every single component of EasyDeL is open for inspection, modification, and customization. There are no black boxes here.
Hackability at Its Core: We believe in giving you full control. Whether you want to tweak a small function or completely overhaul a training loop, EasyDeL lets you do it.
Custom Code Access: All custom implementations are readily available and well-documented, allowing you to understand, learn from, and modify the internals as needed.
Encourage Experimentation: We actively encourage users to experiment, extend, and improve upon the existing codebase. Your innovations could become the next big feature!
Community-Driven Development: Share your custom implementations and improvements with the community, fostering a collaborative environment for advancing ML research and development.
With EasyDeL, you're not constrained by rigid frameworks. Instead, you have a flexible, powerful toolkit that adapts to your needs, no matter how unique or specialized they may be. Whether you're conducting cutting-edge research or building production-ready ML systems, EasyDeL provides the freedom to innovate without limitations.
EasyDeL provides unparalleled flexibility in customizing and optimizing your models:
Sharding Strategies: Easily customize and experiment with different sharding strategies to optimize performance across multiple devices.
Algorithm Customization: Modify and fine-tune algorithms to suit your specific needs and hardware configurations.
Attention Mechanisms: Choose from over 10 types of attention mechanisms optimized for GPU/TPU/CPU, including:
This level of customization allows you to squeeze every ounce of performance from your hardware while tailoring the model behavior to your exact requirements.
EasyDeL is constantly evolving to meet the needs of the machine learning community. In upcoming updates, we plan to introduce:
Flexibility: EasyDeL offers a modular design that allows researchers and developers to easily mix and match components, experiment with different architectures (including Transformers, Mamba, RWKV, and ...), and adapt models to specific use cases.
Performance: Leveraging the power of JAX and Flax, EasyDeL provides high-performance implementations of state-of-the-art models and training techniques, optimized for both TPUs and GPUs.
Scalability: From small experiments to large-scale model training, EasyDeL provides tools and optimizations to efficiently scale your models and workflows.
Ease of Use: Despite its powerful features, EasyDeL maintains an intuitive API, making it accessible for both beginners and experienced practitioners.
Cutting-Edge Research: quickly implementing the latest advancements in model architectures, training techniques, and optimization methods.
pip install easydel
import easydel as ed
ed.FlexibleAttentionModule.run_attention_benchmarks()
Comprehensive documentation and examples are available at EasyDeL Documentation.
Here's an improved version of your latest updates:
olmo2
, qwen2_moe
, mamba2
, and others.vInference
Engine: Introduced as a robust inference engine for LLMs, with Long-Term Support (LTS).vInferenceApiServer
: Added as a backend for the inference engine, fully compatible with OpenAI APIs.The vInference
class provides a streamlined interface for text generation using pre-trained language models within JAX.
import easydel as ed
from transformers import AutoTokenizer
model, params = ed.AutoEasyDeLModelForCausalLM.from_pretrained(...)
tokenizer = AutoTokenizer.from_pretrained(...)
inference = ed.vInference(
model=model,
params=params,
tokenizer=tokenizer,
generation_config=ed.vInferenceConfig(
temperature=model.generation_config.temperature,
top_k=model.generation_config.top_k,
top_p=model.generation_config.top_p,
bos_token_id=model.generation_config.bos_token_id,
eos_token_id=model.generation_config.eos_token_id,
pad_token_id=model.generation_config.pad_token_id,
streaming_chunks=32,
max_new_tokens=1024,
),
)
vInferenceApiServer
is a Serve API Engine for production or research purposes, providing a stable, efficient, and OpenAI API like API.
import easydel as ed
api_inference = ed.vInferenceApiServer(
{inference.inference_name: inference}
) # you can load multi inferences together
api_inference.fire()
EasyDeLState
acts as a comprehensive container for your EasyDeL model, including training progress, model parameters, and optimizer information.
from easydel import EasyDeLState
state = EasyDeLState.from_pretrained(
pretrained_model_name_or_path="model_name",
dtype=jnp.bfloat16,
param_dtype=jnp.bfloat16,
sharding_axis_dims=(1, -1, 1, 1)
)
from easydel import SFTTrainer, TrainingArguments
trainer = SFTTrainer(
arguments=train_arguments,
train_dataset=train_dataset,
eval_dataset=eval_dataset,
tokenizer=tokenizer,
formatting_func=prompter,
packing=True,
num_of_sequences=max_length,
)
output = trainer.train(flax.core.FrozenDict({"params": params}))
from easydel import DPOTrainer
dpo_trainer = DPOTrainer(
model_state=state,
ref_model_state=ref_state,
beta=0.1,
train_dataset=train_dataset,
eval_dataset=eval_dataset,
tokenizer=tokenizer,
arguments=arguments,
max_length=max_length,
max_completion_length=max_completion_length,
max_prompt_length=max_prompt_length,
)
output = dpo_trainer.train()
Contributions to EasyDeL are welcome! Please fork the repository, make your changes, and submit a pull request.
EasyDeL is released under the Apache v2 license. See the LICENSE file for more details.
If you have any questions or comments about EasyDeL, you can reach out to me at erfanzare810@gmail.com.
To cite EasyDeL in your work:
@misc{Zare Chavoshi_2023,
title={EasyDeL: An open-source library for enhancing and streamlining the training process of machine learning models},
url={https://github.com/erfanzar/EasyDeL},
author={Zare Chavoshi, Erfan},
year={2023}
}
FAQs
An open-source library to make training faster and more optimized in JAX
We found that easydel demonstrated a healthy version release cadence and project activity because the last version was released less than a year ago. It has 1 open source maintainer collaborating on the project.
Did you know?
Socket for GitHub automatically highlights issues in each pull request and monitors the health of all your open source dependencies. Discover the contents of your packages and block harmful activity before you install or update your dependencies.
Security News
Research
The Socket Research Team breaks down a malicious wrapper package that uses obfuscation to harvest credentials and exfiltrate sensitive data.
Research
Security News
Attackers used a malicious npm package typosquatting a popular ESLint plugin to steal sensitive data, execute commands, and exploit developer systems.
Security News
The Ultralytics' PyPI Package was compromised four times in one weekend through GitHub Actions cache poisoning and failure to rotate previously compromised API tokens.