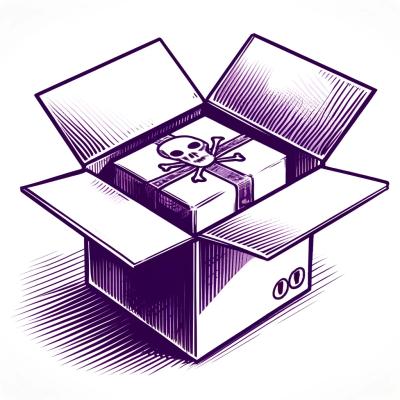
Security News
Research
Data Theft Repackaged: A Case Study in Malicious Wrapper Packages on npm
The Socket Research Team breaks down a malicious wrapper package that uses obfuscation to harvest credentials and exfiltrate sensitive data.
Rust toolbox for Efficient Global Optimization inspired by the EGO implementation in the SMT Python library.
The egobox
package is twofold:
Egor
and the surrogate model Gpx
, mixture of Gaussian processes, written in Rust.pip install egobox
import numpy as np
import egobox as egx
# Objective function
def f_obj(x: np.ndarray) -> np.ndarray:
return (x - 3.5) * np.sin((x - 3.5) / (np.pi))
# Minimize f_opt in [0, 25]
res = egx.Egor(egx.to_specs([[0.0, 25.0]]), seed=42).minimize(f_obj, max_iters=20)
print(f"Optimization f={res.y_opt} at {res.x_opt}") # Optimization f=[-15.12510323] at [18.93525454]
import numpy as np
import egobox as egx
# Training
xtrain = np.array([[0.0, 1.0, 2.0, 3.0, 4.0]]).T
ytrain = np.array([[0.0, 1.0, 1.5, 0.9, 1.0]]).T
gpx = egx.Gpx.builder().fit(xtrain, ytrain)
# Prediction
xtest = np.linspace(0, 4, 20).reshape((-1, 1))
ytest = gpx.predict(xtest)
See the tutorial notebooks and examples folder for more information on the usage of the optimizer and mixture of Gaussian processes surrogate model.
egobox
Rust libraries consists of the following sub-packages.
Name | Version | Documentation | Description |
---|---|---|---|
doe | sampling methods; contains LHS, FullFactorial, Random methods | ||
gp | gaussian process regression; contains Kriging, PLS dimension reduction and sparse methods | ||
moe | mixture of experts using GP models | ||
ego | efficient global optimization with constraints and mixed integer handling |
Depending on the sub-packages you want to use, you have to add following declarations to your Cargo.toml
[dependencies]
egobox-doe = { version = "0.24" }
egobox-gp = { version = "0.24" }
egobox-moe = { version = "0.24" }
egobox-ego = { version = "0.24" }
The table below presents the various features available depending on the subcrate
Name | doe | gp | moe | ego |
---|---|---|---|---|
serializable | ✔️ | ✔️ | ✔️ | |
persistent | ✔️ | ✔️(*) | ||
blas | ✔️ | ✔️ | ✔️ | |
nlopt | ✔️ | ✔️ |
(*) required for mixed-variable gaussian process
When selected, the serialization with serde crate is enabled.
When selected, the save and load as a json file with serde_json crate is enabled.
When selected, the usage of BLAS/LAPACK backend is possible, see below for more information.
When selected, the nlopt crate is used to provide optimizer implementations (ie Cobyla, Slsqp)
Examples (in examples/
sub-packages folder) are run as follows:
cd doe && cargo run --example samplings --release
cd gp && cargo run --example kriging --release
cd moe && cargo run --example clustering --release
cd ego && cargo run --example ackley --release
egobox
relies on linfa project for methods like clustering and dimension reduction, but also try to adopt as far as possible the same coding structures.
As for linfa
, the linear algebra routines used in gp
, moe
ad ego
are provided by the pure-Rust linfa-linalg crate, the default linear algebra provider.
Otherwise, you can choose an external BLAS/LAPACK backend available through the ndarray-linalg crate. In this case, you have to specify the blas
feature and a linfa
BLAS/LAPACK backend feature (more information in linfa features).
Thus, for instance, to use gp
with the Intel MKL BLAS/LAPACK backend, you could specify in your Cargo.toml
the following features:
[dependencies]
egobox-gp = { version = "0.24", features = ["blas", "linfa/intel-mkl-static"] }
or you could run the gp
example as follows:
cd gp && cargo run --example kriging --release --features blas,linfa/intel-mkl-static
If you find this project useful for your research, you may cite it as follows:
@article{
Lafage2022,
author = {Rémi Lafage},
title = {egobox, a Rust toolbox for efficient global optimization},
journal = {Journal of Open Source Software}
year = {2022},
doi = {10.21105/joss.04737},
url = {https://doi.org/10.21105/joss.04737},
publisher = {The Open Journal},
volume = {7},
number = {78},
pages = {4737},
}
Additionally, you may consider adding a star to the repository. This positive feedback improves the visibility of the project.
Bartoli, N., Lefebvre, T., Dubreuil, S., Olivanti, R., Priem, R., Bons, N., Martins, J. R. R. A., & Morlier, J. (2019). Adaptive modeling strategy for constrained global optimization with application to aerodynamic wing design. Aerospace Science and Technology, 90, 85–102. https://doi.org/10.1016/j.ast.2019.03.041
Bouhlel, M. A., Bartoli, N., Otsmane, A., & Morlier, J. (2016). Improving kriging surrogates of high-dimensional design models by partial least squares dimension reduction. Structural and Multidisciplinary Optimization, 53(5), 935–952. https://doi.org/10.1007/s00158-015-1395-9
Bouhlel, M. A., Hwang, J. T., Bartoli, N., Lafage, R., Morlier, J., & Martins, J. R. R. A. (2019). A python surrogate modeling framework with derivatives. Advances in Engineering Software, 102662. https://doi.org/10.1016/j.advengsoft.2019.03.005
Dubreuil, S., Bartoli, N., Gogu, C., & Lefebvre, T. (2020). Towards an efficient global multi- disciplinary design optimization algorithm. Structural and Multidisciplinary Optimization, 62(4), 1739–1765. https://doi.org/10.1007/s00158-020-02514-6
Jones, D. R., Schonlau, M., & Welch, W. J. (1998). Efficient global optimization of expensive black-box functions. Journal of Global Optimization, 13(4), 455–492. https://www.researchgate.net/publication/235709802_Efficient_Global_Optimization_of_Expensive_Black-Box_Functions
Diouane, Youssef, et al. "TREGO: a trust-region framework for efficient global optimization." Journal of Global Optimization 86.1 (2023): 1-23. https://arxiv.org/pdf/2101.06808
smtorg. (2018). Surrogate modeling toolbox. In GitHub repository. GitHub. https://github.com/SMTOrg/smt
Licensed under the Apache License, Version 2.0 http://www.apache.org/licenses/LICENSE-2.0
FAQs
A toolbox for efficient global optimization
We found that egobox demonstrated a healthy version release cadence and project activity because the last version was released less than a year ago. It has 1 open source maintainer collaborating on the project.
Did you know?
Socket for GitHub automatically highlights issues in each pull request and monitors the health of all your open source dependencies. Discover the contents of your packages and block harmful activity before you install or update your dependencies.
Security News
Research
The Socket Research Team breaks down a malicious wrapper package that uses obfuscation to harvest credentials and exfiltrate sensitive data.
Research
Security News
Attackers used a malicious npm package typosquatting a popular ESLint plugin to steal sensitive data, execute commands, and exploit developer systems.
Security News
The Ultralytics' PyPI Package was compromised four times in one weekend through GitHub Actions cache poisoning and failure to rotate previously compromised API tokens.