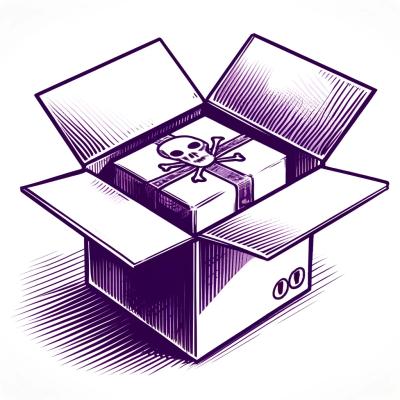
Security News
Research
Data Theft Repackaged: A Case Study in Malicious Wrapper Packages on npm
The Socket Research Team breaks down a malicious wrapper package that uses obfuscation to harvest credentials and exfiltrate sensitive data.
Warning: Starting from version 1.0.1, there are breaking changes in this library. Please review the Usage section for details and update your code accordingly.
The Hampel filter is generally used to detect anomalies in data with a timeseries structure. It basically consists of a sliding window of a parameterizable size. For each window, each observation will be compared with the Median Absolute Deviation (MAD). The observation will be considered an outlier in the case in which it exceeds the MAD by n times (the parameter n is also parameterizable).
For more details, see the Related Links section.
To use the Hampel filter in your Python project, you can install it via pip:
pip install hampel
Here's a simple example of how to use the Hampel filter:
import pandas as pd
from hampel import hampel
# Sample data as a pandas.Series
data = pd.Series([1.0, 2.0, 3.0, 100.0, 4.0, 5.0, 6.0])
# Apply the Hampel filter
result = hampel(data, window_size=3, n_sigma=3.0)
print(result.filtered_data)
When you apply the Hampel filter, it returns a Result
object with the following attributes:
filtered_data
: The data with outliers replaced.
outlier_indices
: Indices of the detected outliers.
medians
: Median values within the sliding window.
median_absolute_deviations
: Median Absolute Deviation (MAD) values within the sliding window.
thresholds
: Threshold values for outlier detection.
You can access these attributes as follows:
result = hampel(data, window_size=3, n_sigma=3.0)
filtered_data = result.filtered_data
outlier_indices = result.outlier_indices
medians = result.medians
mad_values = result.median_absolute_deviations
thresholds = result.thresholds
If you want to directly apply hampel filter to multiple columns in a pandas.Dataframe
,
follow this code:
import pandas as pd
from hampel import hampel
df = pd.DataFrame({
'A': [1.0, 2.0, 3.0, 100.0, 4.0, 5.0, 6.0],
'B': [7.0, 8.0, 9.0, 10.0, 11.0, 12.0, 13.0]
})
# We are just getting the filtered data in this case
filtered_df = df.apply(lambda x: hampel(x).filtered_data, axis=0)
print(df)
data
: The input 1-dimensional data to be filtered (pandas.Series or numpy.ndarray).window_size
(optional): The size of the moving window for outlier detection (default is 5).n_sigma
(optional): The number of standard deviations for outlier detection (default is 3.0).If you want to run the tests, simple run:
make test
See the LICENSE file for details.
Contributions are welcome! Feel free to open issues, submit pull requests, or suggest improvements.
https://medium.com/wwblog/clean-up-your-time-series-data-with-a-hampel-filter-58b0bb3ebb04
FAQs
Python implementation of the Hampel Filter
We found that hampel demonstrated a healthy version release cadence and project activity because the last version was released less than a year ago. It has 1 open source maintainer collaborating on the project.
Did you know?
Socket for GitHub automatically highlights issues in each pull request and monitors the health of all your open source dependencies. Discover the contents of your packages and block harmful activity before you install or update your dependencies.
Security News
Research
The Socket Research Team breaks down a malicious wrapper package that uses obfuscation to harvest credentials and exfiltrate sensitive data.
Research
Security News
Attackers used a malicious npm package typosquatting a popular ESLint plugin to steal sensitive data, execute commands, and exploit developer systems.
Security News
The Ultralytics' PyPI Package was compromised four times in one weekend through GitHub Actions cache poisoning and failure to rotate previously compromised API tokens.