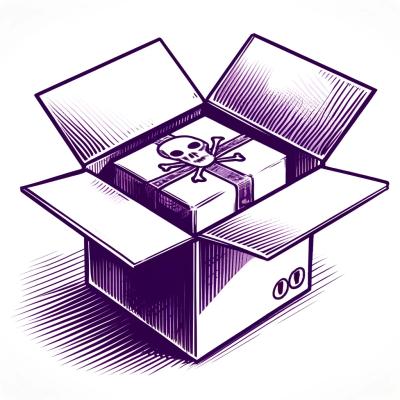
Security News
Research
Data Theft Repackaged: A Case Study in Malicious Wrapper Packages on npm
The Socket Research Team breaks down a malicious wrapper package that uses obfuscation to harvest credentials and exfiltrate sensitive data.
a Python Polars Interface to kdb+/q
k type | n | size | python type | note |
---|---|---|---|---|
boolean | 1 | 1 | bool | |
guid | 2 | 16 | str | |
byte | 4 | 1 | int | |
short | 5 | 2 | int | |
int | 6 | 4 | int | |
long | 7 | 8 | int | |
real | 8 | 4 | float | |
float | 9 | 8 | float | |
char | 10 | 1 | str | |
string | 10 | 1 | str | |
symbol | 11 | * | str | |
timestamp | 12 | 8 | datetime | |
month | 13 | 4 | - | |
date | 14 | 4 | date | 0001.01.01 - 9999.12.31 |
datetime | 15 | 8 | datetime | |
timespan | 16 | 8 | timedelta | |
minute | 17 | 4 | time | 00:00 - 23:59 |
second | 18 | 4 | time | 00:00:00 - 23:59:59 |
time | 19 | 4 | time | 00:00:00.000 - 23:59:59.999 |
k type | n | size | python type |
---|---|---|---|
boolean list | 1 | 1 | pl.Boolean |
guid list | 2 | 16 | pl.List(pl.Binary(16)) |
byte list | 4 | 1 | pl.Uint8 |
short list | 5 | 2 | pl.Int16 |
int list | 6 | 4 | pl.Int32 |
long list | 7 | 8 | pl.Int64 |
real list | 8 | 4 | pl.Float32 |
float list | 9 | 8 | pl.Float64 |
char list | 10 | 1 | pl.Utf8 |
string list | 10 | 1 | pl.Utf8 |
symbol list | 11 | * | pl.Categorical |
timestamp list | 12 | 8 | pl.Datetime |
month list | 13 | 4 | - |
date list | 14 | 4 | pl.Date |
datetime list | 15 | 8 | pl.Datetime |
timespan list | 16 | 8 | pl.Duration |
minute list | 17 | 4 | pl.Time |
second list | 18 | 4 | pl.Time |
time list | 19 | 4 | pl.Time |
table | 98 | * | pl.DataFrame |
dictionary | 99 | * | - |
keyed table | 99 | * | pl.DataFrame |
performance is impacted by converting guid to string, deserialize the uuid to 16 fixed binary list, use .hex() to convert binary to string if required
real/float 0n is mapped to Polars null not NaN
short/int/long 0Nh/i/j, 0Wh/i/j and -0Wh/i/j are mapped to null
df.with_columns([
(pl.col("uuid").apply(lambda u: u.hex()))
])
python type | k type | note |
---|---|---|
bool | boolean | |
int | long | |
float | float | |
str | symbol | |
bytes | string | |
datetime | timestamp | |
date | date | 0001.01.01 - 9999.12.31 |
datetime | datetime | |
timedelta | timespan | |
time | time | 00:00:00.000 - 23:59:59.999 |
python type | k type |
---|---|
dict | dict |
pl.Boolean | boolean |
pl.List(pl.Binary(16)) | guid |
pl.Uint8 | byte |
pl.Int16 | short |
pl.Int32 | int |
pl.Int64 | long |
pl.Float32 | real |
pl.Float64 | float |
pl.Utf8 | char |
pl.Categorical | symbol |
pl.Datetime | timestamp |
pl.Date | date |
pl.Datetime | datetime |
pl.Duration | timespan |
pl.Time | time |
pl.DataFrame | table |
Limited Support for dictionary as arguments, requires
string
as keys.
import polars as pl
import kola
q = kola.Q('localhost', 1800)
# with retries for IO Errors, 1s, 2s, 4s ...
q = kola.Q('localhost', 1800, retries=3)
# with read timeout error, 2s, "Resource temporarily unavailable"
q = kola.Q('localhost', 1800, retries=3, timeout=2)
Automatically connect when querying q process
q.connect()
Automatically disconnect if any IO error
q.disconnect()
q.sync("select from trade where date=last date")
When the first string starts with {
and ends with }
, it is treated as a lambda.
d = {"a": 1, "b": 2}
q.sync("{key x}", d)
For functional query, kola
supports Python Basic Data Type, pl.Series
, pl.DataFrame
and Python Dictionary with string keys and Python Basic Data Type and pl.Series
values.
from datetime import date, time
q.sync(
".gw.query",
"table",
{
"date": date(2023, 11, 21),
"syms": pl.Series("", ["sym0", "sym1"], pl.Categorical),
# 09:00
"startTime": time(9),
# 11:30
"endTime": time(11, 30),
},
)
# pl_df is a Polars DataFrame
q.sync("upsert", "table", pl_df)
# pd_df is a Pandas DataFrame, use pl.DateFrame to cast Pandas DataFrame
q.sync("upsert", "table", pl.DataFrame(pd_df))
# pl_df is a Polars DataFrame
q.asyn("upsert", "table", pl_df)
from kola import QType
q.sync(".u.sub", pl.Series("", ["table1", "table2"], QType.Symbol), "")
# specify symbol filter
q.sync(
".u.sub",
pl.Series("", ["table1", "table2"], QType.Symbol),
pl.Series("", ["sym1", "sym2"], QType.Symbol),
)
while true:
# ("upd", "table", pl.Dataframe)
upd = self.q.receive()
print(upd)
import polars as pl
from kola import generate_ipc
df = pl.DataFrame(
{
"sym": pl.Series("sym", ["a", "b", "c"], pl.Categorical),
"price": [1, 2, 3],
}
)
# without compression
buffer = generate_ipc("sync", False, ["upd", "table", df])
# with compression
buffer = generate_ipc("sync", True, ["upd", "table", df])
Refer to
FAQs
a Python Polars interface to kdb+/q
We found that kola demonstrated a healthy version release cadence and project activity because the last version was released less than a year ago. It has 1 open source maintainer collaborating on the project.
Did you know?
Socket for GitHub automatically highlights issues in each pull request and monitors the health of all your open source dependencies. Discover the contents of your packages and block harmful activity before you install or update your dependencies.
Security News
Research
The Socket Research Team breaks down a malicious wrapper package that uses obfuscation to harvest credentials and exfiltrate sensitive data.
Research
Security News
Attackers used a malicious npm package typosquatting a popular ESLint plugin to steal sensitive data, execute commands, and exploit developer systems.
Security News
The Ultralytics' PyPI Package was compromised four times in one weekend through GitHub Actions cache poisoning and failure to rotate previously compromised API tokens.