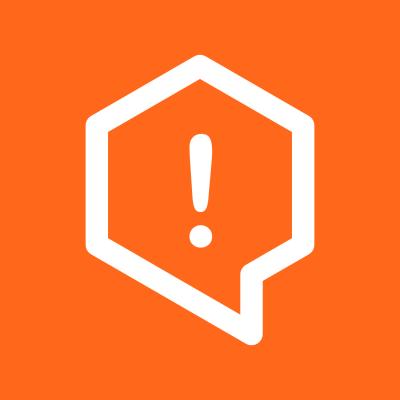
Security News
Fluent Assertions Faces Backlash After Abandoning Open Source Licensing
Fluent Assertions is facing backlash after dropping the Apache license for a commercial model, leaving users blindsided and questioning contributor rights.
Library of helper reference for common data tasks using AWS resources such as S3, MTurk and others
Larry is a library of utilities for common data tasks using AWS for data science and data engineering projects. While boto3 is a great interface for interacting with AWS services, it can be overly complex for data scientists and others who want to perform straightforward operations on data. Rather than spend time worrying about API-specific interactions and parameters. Larry makes it easy to use services like S3, MTurk, and other data-oriented AWS services in a far more functional manner to let you focus on the data rather than syntax. This library is designed to make getting tasks completed in Jupyter Notebooks or AWS Lambda functions as easy as possible by providing simplified interfaces while still giving you access to the underlying boto3 libraries when you need them.
pip install larry
In addition, you can add Larry to your AWS Lambda functions by adding one of the following public Layers:
arn:aws:lambda:us-east-1:981332165467:layer:Larry:7
arn:aws:lambda:us-east-1:981332165467:layer:LarryWithJinja:7
arn:aws:lambda:us-east-1:981332165467:layer:LarryWithJinjaPillow:7
arn:aws:lambda:us-west-2:981332165467:layer:Larry:7
arn:aws:lambda:us-west-2:981332165467:layer:LarryWithJinja:7
arn:aws:lambda:us-west-2:981332165467:layer:LarryWithJinjaPillow:7
By default Larry creates a boto3 session using your default AWS credentials that can be configured using the AWS CLI. To use a different profile, you can change using the following:
import larry as lry
lry.set_session(profile_name='my_profile')
Alternatively, you can pass AWS credentials directly
import larry as lry
lry.set_session(aws_access_key_id='XXXXXXXXXX', aws_secret_access_key='XXXXXXXXXXXXX')
When using boto3 alone, the following is how you would read in a JSON formatted object from S3 into a dict:
import boto3
import json
resource = boto3.resource('s3')
obj = resource.Bucket('mybucket').Object(key='myfile.json').get()
contents = obj['Body'].read()
my_dict = json.loads(contents.decode('utf-8'))
In contrast, Larry takes care of all those steps for you and let's you simply call one function to get your data.
import larry as lry
my_dict = lry.s3.read_dict(bucket='mybucket', key='myfile.json')
In addition to accessing data using bucket/key pairs, you can S3 URIs like those commonly used in SageMaker.
my_dict2 = lry.s3.read_dict(uri='s3://mybucket/myfile.json')
To write files to S3, simply call write_object
to write your object out in the appropriate format:
# Write json to S3
my_dict = {'key': 'val'}
lry.s3.write_object(my_dict, bucket='mybucket', key='myfile.json')
# Write a list of strings to S3 as rows
my_list = ['a','b','c','d']
lry.s3.write_object(my_list, bucket='mybucket', key='myfile.txt')
# Write a JSON lines file to S3 (commonly used for SageMaker manifest files)
my_dictlist = [{'a': 1}, {'b': 2}, {'c': 3}]
lry.s3.write_object(my_dictlist, bucket='mybucket', key='myfile.jsonl')
Larry is especially useful for services like MTurk which have more complex interaction patterns and legacy aspects of their APIs. The MTurk module includes a number of features to make using MTurk much easier:
The combination of these features means that creating a HIT in MTurk is as easy as the following:
import larry as lry
# Indicate we want to use the production environment
lry.mturk.use_production()
# Identify the task template we want to use and the parameters we'll populate in the template
template_uri = 's3://mybucket/templates/imageCat.html'
task_data = {'image_url': 'https://mywebsite.com/images/233.jpg'}
# Add some tracking information we can pass through in our RequesterAnnotation
task_data['request_id'] = 'MY_TRACKING_ID'
# Create a HIT
hit = lry.mturk.create_hit(title='Test task', description='Categorize images',
reward_cents=5, max_assignments=5, lifetime=86400, assignment_duration=600,
question_template_uri=template_uri, template_context=task_data, annotation=task_data)
# Display where the HIT can be viewed on the Worker website
print('HIT {} created, preview at {}'.format(hit.hit_id, hit.preview))
Getting the results from that task is as simple as the following:
import larry as lry
hit_id = 'HIT_ID_FROM_EARLIER'
# Indicate we want to use the production environment
lry.mturk.use_production()
# retrieve the HIT
hit = lry.mturk.get_hit(hit_id)
# retrieve the requester annotation data
task_data = hit.annotation
# get the results
hit.retrieve_assignments()
for assignment in hit.assignments:
print('Worker {} responded with {}'.format(assignment.worker_id, assignment.answer['category']))
More features will be added over time, feel free to submit your feature suggestions.
FAQs
Library of helper reference for common data tasks using AWS resources such as S3, MTurk and others
We found that larry demonstrated a healthy version release cadence and project activity because the last version was released less than a year ago. It has 1 open source maintainer collaborating on the project.
Did you know?
Socket for GitHub automatically highlights issues in each pull request and monitors the health of all your open source dependencies. Discover the contents of your packages and block harmful activity before you install or update your dependencies.
Security News
Fluent Assertions is facing backlash after dropping the Apache license for a commercial model, leaving users blindsided and questioning contributor rights.
Research
Security News
Socket researchers uncover the risks of a malicious Python package targeting Discord developers.
Security News
The UK is proposing a bold ban on ransomware payments by public entities to disrupt cybercrime, protect critical services, and lead global cybersecurity efforts.